A universal model of RNA.DNA:DNA triplex formation accurately predicts genome-wide RNA-DNA interactions
- PMID: 36239395
- PMCID: PMC9677506
- DOI: 10.1093/bib/bbac445
A universal model of RNA.DNA:DNA triplex formation accurately predicts genome-wide RNA-DNA interactions
Abstract
RNA.DNA:DNA triple helix (triplex) formation is a form of RNA-DNA interaction which regulates gene expression but is difficult to study experimentally in vivo. This makes accurate computational prediction of such interactions highly important in the field of RNA research. Current predictive methods use canonical Hoogsteen base pairing rules, which whilst biophysically valid, may not reflect the plastic nature of cell biology. Here, we present the first optimization approach to learn a probabilistic model describing RNA-DNA interactions directly from motifs derived from triplex sequencing data. We find that there are several stable interaction codes, including Hoogsteen base pairing and novel RNA-DNA base pairings, which agree with in vitro measurements. We implemented these findings in TriplexAligner, a program that uses the determined interaction codes to predict triplex binding. TriplexAligner predicts RNA-DNA interactions identified in all-to-all sequencing data more accurately than all previously published tools in human and mouse and also predicts previously studied triplex interactions with known regulatory functions. We further validated a novel triplex interaction using biophysical experiments. Our work is an important step towards better understanding of triplex formation and allows genome-wide analyses of RNA-DNA interactions.
Keywords: DNA; RNA; RNA–DNA interaction; Triplex; machine learning.
© The Author(s) 2022. Published by Oxford University Press.
Figures
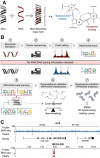
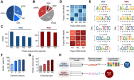
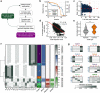
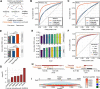
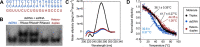
Similar articles
-
Computational Methods to Study DNA:DNA:RNA Triplex Formation by lncRNAs.Noncoding RNA. 2023 Jan 21;9(1):10. doi: 10.3390/ncrna9010010. Noncoding RNA. 2023. PMID: 36827543 Free PMC article. Review.
-
Isolation and genome-wide characterization of cellular DNA:RNA triplex structures.Nucleic Acids Res. 2019 Mar 18;47(5):2306-2321. doi: 10.1093/nar/gky1305. Nucleic Acids Res. 2019. PMID: 30605520 Free PMC article.
-
Purine- and pyrimidine-triple-helix-forming oligonucleotides recognize qualitatively different target sites at the ribosomal DNA locus.RNA. 2018 Mar;24(3):371-380. doi: 10.1261/rna.063800.117. Epub 2017 Dec 8. RNA. 2018. PMID: 29222118 Free PMC article.
-
The Mapping of Predicted Triplex DNA:RNA in the Drosophila Genome Reveals a Prominent Location in Development- and Morphogenesis-Related Genes.G3 (Bethesda). 2017 Jul 5;7(7):2295-2304. doi: 10.1534/g3.117.042911. G3 (Bethesda). 2017. PMID: 28515050 Free PMC article.
-
New approaches toward recognition of nucleic acid triple helices.Acc Chem Res. 2011 Feb 15;44(2):134-46. doi: 10.1021/ar100113q. Epub 2010 Nov 12. Acc Chem Res. 2011. PMID: 21073199 Free PMC article. Review.
Cited by
-
3plex enables deep computational investigation of triplex forming lncRNAs.Comput Struct Biotechnol J. 2023 May 17;21:3091-3102. doi: 10.1016/j.csbj.2023.05.016. eCollection 2023. Comput Struct Biotechnol J. 2023. PMID: 37273849 Free PMC article.
-
Computational Methods to Study DNA:DNA:RNA Triplex Formation by lncRNAs.Noncoding RNA. 2023 Jan 21;9(1):10. doi: 10.3390/ncrna9010010. Noncoding RNA. 2023. PMID: 36827543 Free PMC article. Review.
-
LINC01137/miR-186-5p/WWOX: a novel axis identified from WWOX-related RNA interactome in bladder cancer.Front Genet. 2023 Jul 13;14:1214968. doi: 10.3389/fgene.2023.1214968. eCollection 2023. Front Genet. 2023. PMID: 37519886 Free PMC article.