Guiding principle of reservoir computing based on "small-world" network
- PMID: 36202989
- PMCID: PMC9537422
- DOI: 10.1038/s41598-022-21235-y
Guiding principle of reservoir computing based on "small-world" network
Abstract
Reservoir computing is a computational framework of recurrent neural networks and is gaining attentions because of its drastically simplified training process. For a given task to solve, however, the methodology has not yet been established how to construct an optimal reservoir. While, "small-world" network has been known to represent networks in real-world such as biological systems and social community. This network is categorized amongst those that are completely regular and totally disordered, and it is characterized by highly-clustered nodes with a short path length. This study aims at providing a guiding principle of systematic synthesis of desired reservoirs by taking advantage of controllable parameters of the small-world network. We will validate the methodology using two different types of benchmark tests-classification task and prediction task.
© 2022. The Author(s).
Conflict of interest statement
The author declares no competing interests.
Figures
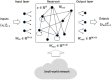
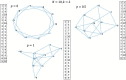
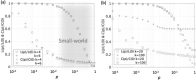
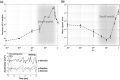
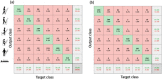
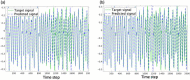
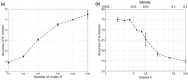
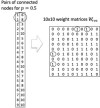
Similar articles
-
Reservoir computing models based on spiking neural P systems for time series classification.Neural Netw. 2024 Jan;169:274-281. doi: 10.1016/j.neunet.2023.10.041. Epub 2023 Oct 28. Neural Netw. 2024. PMID: 37918270
-
Reservoir computing using networks of memristors: effects of topology and heterogeneity.Nanoscale. 2023 Jun 8;15(22):9663-9674. doi: 10.1039/d2nr07275k. Nanoscale. 2023. PMID: 37211815
-
On the correlation between reservoir metrics and performance for time series classification under the influence of synaptic plasticity.PLoS One. 2014 Jul 10;9(7):e101792. doi: 10.1371/journal.pone.0101792. eCollection 2014. PLoS One. 2014. PMID: 25010415 Free PMC article.
-
Recent advances in physical reservoir computing: A review.Neural Netw. 2019 Jul;115:100-123. doi: 10.1016/j.neunet.2019.03.005. Epub 2019 Mar 20. Neural Netw. 2019. PMID: 30981085 Review.
-
Rotating neurons for all-analog implementation of cyclic reservoir computing.Nat Commun. 2022 Mar 23;13(1):1549. doi: 10.1038/s41467-022-29260-1. Nat Commun. 2022. PMID: 35322037 Free PMC article.
References
-
- Verstraeten D, Schrauwen B, Stroobandt D, Van Campenhout J. Isolated word recognition with the liquid state machine: A case study. Inf. Process. Lett. 2005;95:521–528. doi: 10.1016/j.ipl.2005.05.019. - DOI
-
- Lukoševicius M, Jaeger H. Reservoir computing approaches to recurrent neural network training. Comput. Sci. Rev. 2009;3:127–149. doi: 10.1016/j.cosrev.2009.03.005. - DOI
-
- Jaeger. H. The ‘echo state’ approach to analyzing and training recurrent neural networks. Technical Report GMD Report148, German National Research Center for Information Technology (2001).
MeSH terms
LinkOut - more resources
Full Text Sources