BIONIC: biological network integration using convolutions
- PMID: 36192463
- PMCID: PMC11236286
- DOI: 10.1038/s41592-022-01616-x
BIONIC: biological network integration using convolutions
Abstract
Biological networks constructed from varied data can be used to map cellular function, but each data type has limitations. Network integration promises to address these limitations by combining and automatically weighting input information to obtain a more accurate and comprehensive representation of the underlying biology. We developed a deep learning-based network integration algorithm that incorporates a graph convolutional network framework. Our method, BIONIC (Biological Network Integration using Convolutions), learns features that contain substantially more functional information compared to existing approaches. BIONIC has unsupervised and semisupervised learning modes, making use of available gene function annotations. BIONIC is scalable in both size and quantity of the input networks, making it feasible to integrate numerous networks on the scale of the human genome. To demonstrate the use of BIONIC in identifying new biology, we predicted and experimentally validated essential gene chemical-genetic interactions from nonessential gene profiles in yeast.
© 2022. The Author(s), under exclusive licence to Springer Nature America, Inc.
Figures

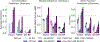
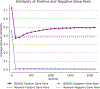
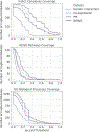
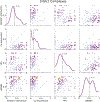
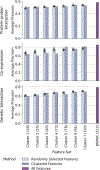
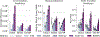
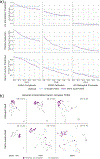
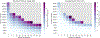
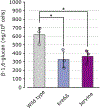
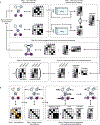
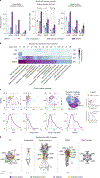
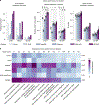
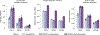
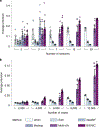
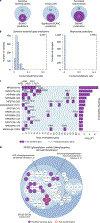
Similar articles
-
Predicting gene function using similarity learning.BMC Genomics. 2013;14 Suppl 4(Suppl 4):S4. doi: 10.1186/1471-2164-14-S4-S4. Epub 2013 Oct 1. BMC Genomics. 2013. PMID: 24266903 Free PMC article.
-
BIONIC: discovering new biology through deep learning-based network integration.Nat Methods. 2022 Oct;19(10):1185-1186. doi: 10.1038/s41592-022-01617-w. Nat Methods. 2022. PMID: 36192466 No abstract available.
-
Implementing artificial neural networks through bionic construction.PLoS One. 2019 Feb 22;14(2):e0212368. doi: 10.1371/journal.pone.0212368. eCollection 2019. PLoS One. 2019. PMID: 30794587 Free PMC article.
-
An Experimental Approach to Genome Annotation: This report is based on a colloquium sponsored by the American Academy of Microbiology held July 19-20, 2004, in Washington, DC.Washington (DC): American Society for Microbiology; 2004. Washington (DC): American Society for Microbiology; 2004. PMID: 33001599 Free Books & Documents. Review.
-
Graph- and rule-based learning algorithms: a comprehensive review of their applications for cancer type classification and prognosis using genomic data.Brief Bioinform. 2020 Mar 23;21(2):368-394. doi: 10.1093/bib/bby120. Brief Bioinform. 2020. PMID: 30649169 Free PMC article. Review.
Cited by
-
Big data and deep learning for RNA biology.Exp Mol Med. 2024 Jun;56(6):1293-1321. doi: 10.1038/s12276-024-01243-w. Epub 2024 Jun 14. Exp Mol Med. 2024. PMID: 38871816 Free PMC article. Review.
-
Joint representation of molecular networks from multiple species improves gene classification.PLoS Comput Biol. 2024 Jan 10;20(1):e1011773. doi: 10.1371/journal.pcbi.1011773. eCollection 2024 Jan. PLoS Comput Biol. 2024. PMID: 38198480 Free PMC article.
-
Mapping the Multiscale Proteomic Organization of Cellular and Disease Phenotypes.Annu Rev Biomed Data Sci. 2024 Aug;7(1):369-389. doi: 10.1146/annurev-biodatasci-102423-113534. Epub 2024 Jul 24. Annu Rev Biomed Data Sci. 2024. PMID: 38748859 Free PMC article. Review.
-
Heterogeneous network approaches to protein pathway prediction.Comput Struct Biotechnol J. 2024 Jun 27;23:2727-2739. doi: 10.1016/j.csbj.2024.06.022. eCollection 2024 Dec. Comput Struct Biotechnol J. 2024. PMID: 39035835 Free PMC article. Review.
-
Interpreting biologically informed neural networks for enhanced proteomic biomarker discovery and pathway analysis.Nat Commun. 2023 Sep 2;14(1):5359. doi: 10.1038/s41467-023-41146-4. Nat Commun. 2023. PMID: 37660105 Free PMC article.
References
-
- Fraser AG & Marcotte EM A probabilistic view of gene function. Nat. Genet 36, 559 (2004). - PubMed
Publication types
MeSH terms
Grants and funding
LinkOut - more resources
Full Text Sources
Molecular Biology Databases