Investigating differential abundance methods in microbiome data: A benchmark study
- PMID: 36074761
- PMCID: PMC9488820
- DOI: 10.1371/journal.pcbi.1010467
Investigating differential abundance methods in microbiome data: A benchmark study
Abstract
The development of increasingly efficient and cost-effective high throughput DNA sequencing techniques has enhanced the possibility of studying complex microbial systems. Recently, researchers have shown great interest in studying the microorganisms that characterise different ecological niches. Differential abundance analysis aims to find the differences in the abundance of each taxa between two classes of subjects or samples, assigning a significance value to each comparison. Several bioinformatic methods have been specifically developed, taking into account the challenges of microbiome data, such as sparsity, the different sequencing depth constraint between samples and compositionality. Differential abundance analysis has led to important conclusions in different fields, from health to the environment. However, the lack of a known biological truth makes it difficult to validate the results obtained. In this work we exploit metaSPARSim, a microbial sequencing count data simulator, to simulate data with differential abundance features between experimental groups. We perform a complete comparison of recently developed and established methods on a common benchmark with great effort to the reliability of both the simulated scenarios and the evaluation metrics. The performance overview includes the investigation of numerous scenarios, studying the effect on methods' results on the main covariates such as sample size, percentage of differentially abundant features, sequencing depth, feature variability, normalisation approach and ecological niches. Mainly, we find that methods show a good control of the type I error and, generally, also of the false discovery rate at high sample size, while recall seem to depend on the dataset and sample size.
Conflict of interest statement
The authors have declared that no competing interests exist.
Figures
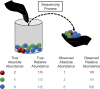
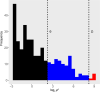
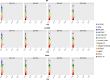
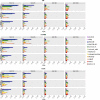
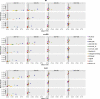
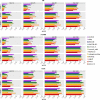
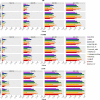
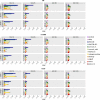
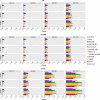
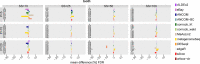
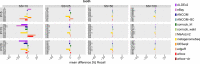
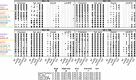
Similar articles
-
Depressing time: Waiting, melancholia, and the psychoanalytic practice of care.In: Kirtsoglou E, Simpson B, editors. The Time of Anthropology: Studies of Contemporary Chronopolitics. Abingdon: Routledge; 2020. Chapter 5. In: Kirtsoglou E, Simpson B, editors. The Time of Anthropology: Studies of Contemporary Chronopolitics. Abingdon: Routledge; 2020. Chapter 5. PMID: 36137063 Free Books & Documents. Review.
-
Far Posterior Approach for Rib Fracture Fixation: Surgical Technique and Tips.JBJS Essent Surg Tech. 2024 Dec 6;14(4):e23.00094. doi: 10.2106/JBJS.ST.23.00094. eCollection 2024 Oct-Dec. JBJS Essent Surg Tech. 2024. PMID: 39650795 Free PMC article.
-
Qualitative evidence synthesis informing our understanding of people's perceptions and experiences of targeted digital communication.Cochrane Database Syst Rev. 2019 Oct 23;10(10):ED000141. doi: 10.1002/14651858.ED000141. Cochrane Database Syst Rev. 2019. PMID: 31643081 Free PMC article.
-
RETRACTED: Hydroxychloroquine and azithromycin as a treatment of COVID-19: results of an open-label non-randomized clinical trial.Int J Antimicrob Agents. 2020 Jul;56(1):105949. doi: 10.1016/j.ijantimicag.2020.105949. Epub 2020 Mar 20. Int J Antimicrob Agents. 2020. Retraction in: Int J Antimicrob Agents. 2025 Jan;65(1):107416. doi: 10.1016/j.ijantimicag.2024.107416 PMID: 32205204 Free PMC article. Retracted. Clinical Trial.
-
Pharmacological treatments in panic disorder in adults: a network meta-analysis.Cochrane Database Syst Rev. 2023 Nov 28;11(11):CD012729. doi: 10.1002/14651858.CD012729.pub3. Cochrane Database Syst Rev. 2023. PMID: 38014714 Free PMC article. Review.
Cited by
-
Emergent Functional Organization of Gut Microbiomes in Health and Diseases.Biomolecules. 2023 Dec 20;14(1):5. doi: 10.3390/biom14010005. Biomolecules. 2023. PMID: 38275746 Free PMC article.
-
Interpretable machine learning decodes soil microbiome's response to drought stress.Environ Microbiome. 2024 May 29;19(1):35. doi: 10.1186/s40793-024-00578-1. Environ Microbiome. 2024. PMID: 38812054 Free PMC article.
-
Examining the relationship between the oral microbiome, alcohol intake and alcohol-comorbid neuropsychological disorders: protocol for a scoping review.BMJ Open. 2024 Mar 21;14(3):e079823. doi: 10.1136/bmjopen-2023-079823. BMJ Open. 2024. PMID: 38514150 Free PMC article.
-
Tryptophan metabolism, gut microbiota, and carotid artery plaque in women with and without HIV infection.AIDS. 2024 Feb 1;38(2):223-233. doi: 10.1097/QAD.0000000000003596. Epub 2023 May 11. AIDS. 2024. PMID: 37199567
-
Uncover a microbiota signature of upper respiratory tract in patients with SARS-CoV-2 + .Sci Rep. 2023 Oct 6;13(1):16867. doi: 10.1038/s41598-023-43040-x. Sci Rep. 2023. PMID: 37803040 Free PMC article.
References
-
- Daisley BA, Chanyi RM, Abdur-Rashid K, Al KF, Gibbons S, Chmiel JA, et al.. Abiraterone acetate preferentially enriches for the gut commensal Akkermansia muciniphila in castrate-resistant prostate cancer patients. Nat Commun. 2020. Sep;11(1):4822. doi: 10.1038/s41467-020-18649-5 - DOI - PMC - PubMed
Publication types
MeSH terms
Grants and funding
LinkOut - more resources
Full Text Sources
Other Literature Sources