Cancer-driving mutations are enriched in genic regions intolerant to germline variation
- PMID: 36026442
- PMCID: PMC9417173
- DOI: 10.1126/sciadv.abo6371
Cancer-driving mutations are enriched in genic regions intolerant to germline variation
Abstract
Large reference datasets of protein-coding variation in human populations have allowed us to determine which genes and genic subregions are intolerant to germline genetic variation. There is also a growing number of genes implicated in severe Mendelian diseases that overlap with genes implicated in cancer. We hypothesized that cancer-driving mutations might be enriched in genic subregions that are depleted of germline variation relative to somatic variation. We introduce a new metric, OncMTR (oncology missense tolerance ratio), which uses 125,748 exomes in the Genome Aggregation Database (gnomAD) to identify these genic subregions. We demonstrate that OncMTR can significantly predict driver mutations implicated in hematologic malignancies. Divergent OncMTR regions were enriched for cancer-relevant protein domains, and overlaying OncMTR scores on protein structures identified functionally important protein residues. Last, we performed a rare variant, gene-based collapsing analysis on an independent set of 394,694 exomes from the UK Biobank and find that OncMTR markedly improves genetic signals for hematologic malignancies.
Figures
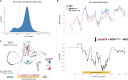
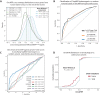
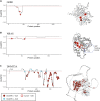
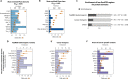
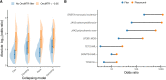
Similar articles
-
Origins and characterization of variants shared between databases of somatic and germline human mutations.BMC Bioinformatics. 2020 Jun 4;21(1):227. doi: 10.1186/s12859-020-3508-8. BMC Bioinformatics. 2020. PMID: 32498674 Free PMC article.
-
Framework for microRNA variant annotation and prioritization using human population and disease datasets.Hum Mutat. 2019 Jan;40(1):73-89. doi: 10.1002/humu.23668. Epub 2018 Nov 8. Hum Mutat. 2019. PMID: 30302893 Free PMC article.
-
Association of Inherited Mutations in DNA Repair Genes with Localized Prostate Cancer.Eur Urol. 2022 Jun;81(6):559-567. doi: 10.1016/j.eururo.2021.09.029. Epub 2021 Oct 25. Eur Urol. 2022. PMID: 34711450 Free PMC article.
-
Germline mutations and blood malignancy (Review).Oncol Rep. 2021 Jan;45(1):49-57. doi: 10.3892/or.2020.7846. Epub 2020 Nov 11. Oncol Rep. 2021. PMID: 33200226 Review.
-
Improving the detection of patients with inherited predispositions to hematologic malignancies using next-generation sequencing-based leukemia prognostication panels.Cancer. 2018 Jul 1;124(13):2704-2713. doi: 10.1002/cncr.31331. Epub 2018 Apr 6. Cancer. 2018. PMID: 29682723 Review.
Cited by
-
Disease prediction with multi-omics and biomarkers empowers case-control genetic discoveries in the UK Biobank.Nat Genet. 2024 Sep;56(9):1821-1831. doi: 10.1038/s41588-024-01898-1. Epub 2024 Sep 11. Nat Genet. 2024. PMID: 39261665 Free PMC article.
-
The frequency of somatic mutations in cancer predicts the phenotypic relevance of germline mutations.Front Genet. 2023 Jan 9;13:1045301. doi: 10.3389/fgene.2022.1045301. eCollection 2022. Front Genet. 2023. PMID: 36699457 Free PMC article.
References
-
- Karczewski K. J., Francioli L. C., Tiao G., Cummings B. B., Alföldi J., Wang Q., Collins R. L., Laricchia K. M., Ganna A., Birnbaum D. P., Gauthier L. D., Brand H., Solomonson M., Watts N. A., Rhodes D., Singer-Berk M., England E. M., Seaby E. G., Kosmicki J. A., Walters R. K., Tashman K., Farjoun Y., Banks E., Poterba T., Wang A., Seed C., Whiffin N., Chong J. X., Samocha K. E., Pierce-Hoffman E., Zappala Z., O’Donnell-Luria A. H., Minikel E. V., Weisburd B., Lek M., Ware J. S., Vittal C., Armean I. M., Bergelson L., Cibulskis K., Connolly K. M., Covarrubias M., Donnelly S., Ferriera S., Gabriel S., Gentry J., Gupta N., Jeandet T., Kaplan D., Llanwarne C., Munshi R., Novod S., Petrillo N., Roazen D., Ruano-Rubio V., Saltzman A., Schleicher M., Soto J., Tibbetts K., Tolonen C., Wade G., Talkowski M. E.; Genome Aggregation Database Consortium, Aguilar Salinas C. A., Ahmad T., Albert C. M., Ardissino D., Atzmon G., Barnard J., Beaugerie L., Benjamin E. J., Boehnke M., Bonnycastle L. L., Bottinger E. P., Bowden D. W., Bown M. J., Chambers J. C., Chan J. C., Chasman D., Cho J., Chung M. K., Cohen B., Correa A., Dabelea D., Daly M. J., Darbar D., Duggirala R., Dupuis J., Ellinor P. T., Elosua R., Erdmann J., Esko T., Färkkilä M., Florez J., Franke A., Getz G., Glaser B., Glatt S. J., Goldstein D., Gonzalez C., Groop L., Haiman C., Hanis C., Harms M., Hiltunen M., Holi M. M., Hultman C. M., Kallela M., Kaprio J., Kathiresan S., Kim B. J., Kim Y. J., Kirov G., Kooner J., Koskinen S., Krumholz H. M., Kugathasan S., Kwak S. H., Laakso M., Lehtimäki T., Loos R. J. F., Lubitz S. A., Ma R. C. W., MacArthur D. G., Marrugat J., Mattila K. M., McCarroll S., McCarthy M. I., McGovern D., McPherson R., Meigs J. B., Melander O., Metspalu A., Neale B. M., Nilsson P. M., O’Donovan M. C., Ongur D., Orozco L., Owen M. J., Palmer C. N. A., Palotie A., Park K. S., Pato C., Pulver A. E., Rahman N., Remes A. M., Rioux J. D., Ripatti S., Roden D. M., Saleheen D., Salomaa V., Samani N. J., Scharf J., Schunkert H., Shoemaker M. B., Sklar P., Soininen H., Sokol H., Spector T., Sullivan P. F., Suvisaari J., Tai E. S., Teo Y. Y., Tiinamaija T., Tsuang M., Turner D., Tusie-Luna T., Vartiainen E., Vawter M. P., Ware J. S., Watkins H., Weersma R. K., Wessman M., Wilson J. G., Xavier R. J., Neale B. M., Daly M. J., MacArthur D. G., The mutational constraint spectrum quantified from variation in 141,456 humans. Nature 581, 434–443 (2020). - PMC - PubMed
-
- Wang Q., Dhindsa R. S., Carss K., Harper A. R., Nag A., Tachmazidou I., Vitsios D., Deevi S. V. V., Mackay A., Muthas D., Hühn M., Monkley S., Olsson H.; Astra Zeneca Genomics Initiative, Wasilewski S., Smith K. R., March R., Platt A., Haefliger C., Petrovski S., Rare variant contribution to human disease in 281,104 UK Biobank exomes. Nature 597, 527–532 (2021). - PMC - PubMed
-
- Samocha K. E., Robinson E. B., Sanders S. J., Stevens C., Sabo A., McGrath L. M., Kosmicki J. A., Rehnström K., Mallick S., Kirby A., Wall D. P., MacArthur D. G., Gabriel S. B., DePristo M., Purcell S. M., Palotie A., Boerwinkle E., Buxbaum J. D., Cook E. H. Jr., Gibbs R. A., Schellenberg G. D., Sutcliffe J. S., Devlin B., Roeder K., Neale B. M., Daly M. J., A framework for the interpretation of de novo mutation in human disease. Nat. Genet. 46, 944–950 (2014). - PMC - PubMed
MeSH terms
Grants and funding
LinkOut - more resources
Full Text Sources