Only Words Count; the Rest Is Mere Chattering: A Cross-Disciplinary Approach to the Verbal Expression of Emotional Experience
- PMID: 36004863
- PMCID: PMC9404916
- DOI: 10.3390/bs12080292
Only Words Count; the Rest Is Mere Chattering: A Cross-Disciplinary Approach to the Verbal Expression of Emotional Experience
Abstract
The analysis of sequences of words and prosody, meter, and rhythm provided in an interview addressing the capacity to identify and describe emotions represents a powerful tool to reveal emotional processing. The ability to express and identify emotions was analyzed by means of the Toronto Structured Interview for Alexithymia (TSIA), and TSIA transcripts were analyzed by Natural Language Processing to shed light on verbal features. The brain correlates of the capacity to translate emotional experience into words were determined through cortical thickness measures. A machine learning methodology proved that individuals with deficits in identifying and describing emotions (n = 7) produced language distortions, frequently used the present tense of auxiliary verbs, and few possessive determiners, as well as scarcely connected the speech, in comparison to individuals without deficits (n = 7). Interestingly, they showed high cortical thickness at left temporal pole and low at isthmus of the right cingulate cortex. Overall, we identified the neuro-linguistic pattern of the expression of emotional experience.
Keywords: Natural Language Processing; alexithymia; cortical thickness; emotional processing; machine learning.
Conflict of interest statement
The authors declare no conflict of interest.
Figures
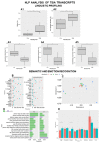
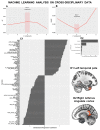
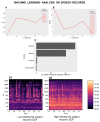
Similar articles
-
Alexithymia according to Bucci's multiple code theory: A preliminary investigation with healthy and hypertensive individuals.Psychol Psychother. 2018 Jun;91(2):232-247. doi: 10.1111/papt.12158. Epub 2017 Oct 3. Psychol Psychother. 2018. PMID: 28972694
-
Effect of experience information on emotional word processing in alexithymia.J Affect Disord. 2019 Dec 1;259:251-258. doi: 10.1016/j.jad.2019.08.068. Epub 2019 Aug 20. J Affect Disord. 2019. PMID: 31446387
-
Does the 20-item Toronto Alexithymia Scale measure alexithymia? A study of natural language use.Psychol Rep. 2009 Dec;105(3 Pt 1):945-56. doi: 10.2466/PR0.105.3.945-956. Psychol Rep. 2009. PMID: 20099558
-
[Anatomic and topographic models of the cerebral areas that activates during the linguistic functions].Rev Neurol. 2008 Dec 16-31;47(12):653-8. Rev Neurol. 2008. PMID: 19085883 Review. Spanish.
-
Cortical influences in emotion.J Clin Neurophysiol. 1998 Sep;15(5):409-23. doi: 10.1097/00004691-199809000-00005. J Clin Neurophysiol. 1998. PMID: 9821068 Review.
Cited by
-
The Cerebellum as an Embodying Machine.Neuroscientist. 2024 Apr;30(2):229-246. doi: 10.1177/10738584221120187. Epub 2022 Sep 2. Neuroscientist. 2024. PMID: 36052895 Free PMC article. Review.
References
-
- Sauter D.A. Is There a Role for Language in Emotion Perception? Emot. Rev. 2018;10:111–115. doi: 10.1177/1754073917693924. - DOI
Grants and funding
LinkOut - more resources
Full Text Sources