Nonlinear optimal control of a mean-field model of neural population dynamics
- PMID: 35990368
- PMCID: PMC9382303
- DOI: 10.3389/fncom.2022.931121
Nonlinear optimal control of a mean-field model of neural population dynamics
Abstract
We apply the framework of nonlinear optimal control to a biophysically realistic neural mass model, which consists of two mutually coupled populations of deterministic excitatory and inhibitory neurons. External control signals are realized by time-dependent inputs to both populations. Optimality is defined by two alternative cost functions that trade the deviation of the controlled variable from its target value against the "strength" of the control, which is quantified by the integrated 1- and 2-norms of the control signal. We focus on a bistable region in state space where one low- ("down state") and one high-activity ("up state") stable fixed points coexist. With methods of nonlinear optimal control, we search for the most cost-efficient control function to switch between both activity states. For a broad range of parameters, we find that cost-efficient control strategies consist of a pulse of finite duration to push the state variables only minimally into the basin of attraction of the target state. This strategy only breaks down once we impose time constraints that force the system to switch on a time scale comparable to the duration of the control pulse. Penalizing control strength via the integrated 1-norm (2-norm) yields control inputs targeting one or both populations. However, whether control inputs to the excitatory or the inhibitory population dominate, depends on the location in state space relative to the bifurcation lines. Our study highlights the applicability of nonlinear optimal control to understand neuronal processing under constraints better.
Keywords: bistability; control of neural dynamics; delay differential-algebraic equations (DDAEs); neural mass models; nonlinear optimal control; nonlinear population dynamics.
Copyright © 2022 Salfenmoser and Obermayer.
Conflict of interest statement
The authors declare that the research was conducted in the absence of any commercial or financial relationships that could be construed as a potential conflict of interest.
Figures
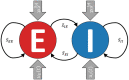
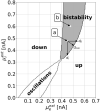
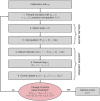
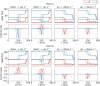
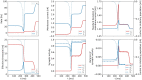
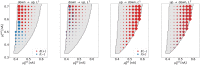
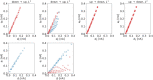
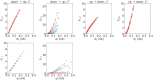
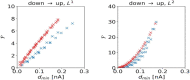
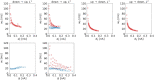
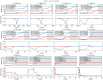
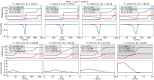
Similar articles
-
Optimal control of a Wilson-Cowan model of neural population dynamics.Chaos. 2023 Apr 1;33(4):043135. doi: 10.1063/5.0144682. Chaos. 2023. PMID: 37097962
-
Koopman Invariant Subspaces and Finite Linear Representations of Nonlinear Dynamical Systems for Control.PLoS One. 2016 Feb 26;11(2):e0150171. doi: 10.1371/journal.pone.0150171. eCollection 2016. PLoS One. 2016. PMID: 26919740 Free PMC article.
-
Multiple oscillatory states in models of collective neuronal dynamics.Int J Neural Syst. 2014 Sep;24(6):1450020. doi: 10.1142/S0129065714500208. Epub 2014 May 30. Int J Neural Syst. 2014. PMID: 25081428
-
Finite-size and correlation-induced effects in mean-field dynamics.J Comput Neurosci. 2011 Nov;31(3):453-84. doi: 10.1007/s10827-011-0320-5. Epub 2011 Mar 8. J Comput Neurosci. 2011. PMID: 21384156 Review.
-
Is there chaos in the brain? II. Experimental evidence and related models.C R Biol. 2003 Sep;326(9):787-840. doi: 10.1016/j.crvi.2003.09.011. C R Biol. 2003. PMID: 14694754 Review.
References
-
- Au J., Karsten C., Buschkuehl M., Jaeggi S. (2017). Optimizing transcranial direct current stimulation protocols to promote long-term learning. J. Cogn. Enhan. 1, 65–72. 10.1007/s41465-017-0007-6 - DOI
-
- Berkovitz L., Medhin N. (2012). Nonlinear Optimal Control Theory. Boca Raton, FL: Chapman & Hall; CRC Applied Mathematics &Nonlinear Science. Taylor & Francis.
LinkOut - more resources
Full Text Sources