Prognosis of Alzheimer's Disease Using Quantitative Mass Spectrometry of Human Blood Plasma Proteins and Machine Learning
- PMID: 35887259
- PMCID: PMC9318764
- DOI: 10.3390/ijms23147907
Prognosis of Alzheimer's Disease Using Quantitative Mass Spectrometry of Human Blood Plasma Proteins and Machine Learning
Abstract
Early recognition of the risk of Alzheimer's disease (AD) onset is a global challenge that requires the development of reliable and affordable screening methods for wide-scale application. Proteomic studies of blood plasma are of particular relevance; however, the currently proposed differentiating markers are poorly consistent. The targeted quantitative multiple reaction monitoring (MRM) assay of the reported candidate biomarkers (CBs) can contribute to the creation of a consistent marker panel. An MRM-MS analysis of 149 nondepleted EDTA-plasma samples (MHRC, Russia) of patients with AD (n = 47), mild cognitive impairment (MCI, n = 36), vascular dementia (n = 8), frontotemporal dementia (n = 15), and an elderly control group (n = 43) was performed using the BAK 125 kit (MRM Proteomics Inc., Canada). Statistical analysis revealed a significant decrease in the levels of afamin, apolipoprotein E, biotinidase, and serum paraoxonase/arylesterase 1 associated with AD. Different training algorithms for machine learning were performed to identify the protein panels and build corresponding classifiers for the AD prognosis. Machine learning revealed 31 proteins that are important for AD differentiation and mostly include reported earlier CBs. The best-performing classifiers reached 80% accuracy, 79.4% sensitivity and 83.6% specificity and were able to assess the risk of developing AD over the next 3 years for patients with MCI. Overall, this study demonstrates the high potential of the MRM approach combined with machine learning to confirm the significance of previously identified CBs and to propose consistent protein marker panels.
Keywords: Alzheimer’s disease; machine learning; mass spectrometry; multiple reaction monitoring; targeted proteomics.
Conflict of interest statement
The authors declare that the research was conducted in the absence of any commercial or financial relationships that could be construed as a potential conflict of interest.
Figures
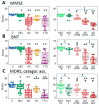
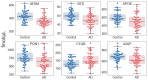
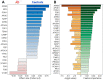
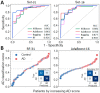
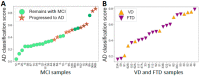
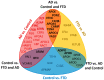
Similar articles
-
A Community-Based Study Identifying Metabolic Biomarkers of Mild Cognitive Impairment and Alzheimer's Disease Using Artificial Intelligence and Machine Learning.J Alzheimers Dis. 2020;78(4):1381-1392. doi: 10.3233/JAD-200305. J Alzheimers Dis. 2020. PMID: 33164929
-
Identification of novel diagnostic panel for mild cognitive impairment and Alzheimer's disease: findings based on urine proteomics and machine learning.Alzheimers Res Ther. 2023 Nov 4;15(1):191. doi: 10.1186/s13195-023-01324-4. Alzheimers Res Ther. 2023. PMID: 37925455 Free PMC article.
-
ApoE4 effects on automated diagnostic classifiers for mild cognitive impairment and Alzheimer's disease.Neuroimage Clin. 2014 Jan 4;4:461-72. doi: 10.1016/j.nicl.2013.12.012. eCollection 2014. Neuroimage Clin. 2014. PMID: 24634832 Free PMC article.
-
Plasma and cerebrospinal fluid amyloid beta for the diagnosis of Alzheimer's disease dementia and other dementias in people with mild cognitive impairment (MCI).Cochrane Database Syst Rev. 2014 Jun 10;2014(6):CD008782. doi: 10.1002/14651858.CD008782.pub4. Cochrane Database Syst Rev. 2014. PMID: 24913723 Free PMC article. Review.
-
Neuropsychiatric Symptoms and Commonly Used Biomarkers of Alzheimer's Disease: A Literature Review from a Machine Learning Perspective.J Alzheimers Dis. 2023;92(4):1131-1146. doi: 10.3233/JAD-221261. J Alzheimers Dis. 2023. PMID: 36872783 Free PMC article. Review.
Cited by
-
First-Trimester Preeclampsia-Induced Disturbance in Maternal Blood Serum Proteome: A Pilot Study.Int J Mol Sci. 2024 Oct 3;25(19):10653. doi: 10.3390/ijms251910653. Int J Mol Sci. 2024. PMID: 39408980 Free PMC article.
-
Absolute Quantitative Targeted Monitoring of Potential Plasma Protein Biomarkers: A Pilot Study on Healthy Individuals.Biomedicines. 2024 Oct 21;12(10):2403. doi: 10.3390/biomedicines12102403. Biomedicines. 2024. PMID: 39457715 Free PMC article.
-
Targeted MRM Quantification of Urinary Proteins in Chronic Kidney Disease Caused by Glomerulopathies.Molecules. 2023 Apr 9;28(8):3323. doi: 10.3390/molecules28083323. Molecules. 2023. PMID: 37110557 Free PMC article.
-
A multi-ethnic proteomic profiling analysis in Alzheimer's disease identifies the disparities in dysregulation of proteins and pathogenesis.PeerJ. 2024 Jul 18;12:e17643. doi: 10.7717/peerj.17643. eCollection 2024. PeerJ. 2024. PMID: 39035156 Free PMC article.
-
Crucial Regulatory Role of Organokines in Relation to Metabolic Changes in Non-Diabetic Obesity.Metabolites. 2023 Feb 14;13(2):270. doi: 10.3390/metabo13020270. Metabolites. 2023. PMID: 36837889 Free PMC article. Review.
References
-
- Mollenhauer B., Bibl M., Wiltfang J., Steinacker P., Ciesielczyk B., Trenkwalder C., Otto M. Total tau protein, phosphorylated tau (181p) protein, β-amyloid1-42, and β-amyloid1-40 in cerebrospinal fluid of patients with dementia with Lewy bodies. Clin. Chem. Lab. Med. 2006;44:192–195. doi: 10.1515/CCLM.2006.035. - DOI - PubMed
-
- Ritchie C., Smailagic N., Ladds E.C., Noel-Storr A.H., Ukoumunne O., Martin S. CSF tau and the CSF tau/ABeta ratio for the diagnosis of Alzheimer’s disease dementia and other dementias in people with mild cognitive impairment (MCI) Cochrane Database Syst. Rev. 2013;3:CD10803. doi: 10.1002/14651858.CD010803. - DOI - PMC - PubMed
MeSH terms
Substances
LinkOut - more resources
Full Text Sources
Medical