Differential Expression Analysis of Single-Cell RNA-Seq Data: Current Statistical Approaches and Outstanding Challenges
- PMID: 35885218
- PMCID: PMC9315519
- DOI: 10.3390/e24070995
Differential Expression Analysis of Single-Cell RNA-Seq Data: Current Statistical Approaches and Outstanding Challenges
Abstract
With the advent of single-cell RNA-sequencing (scRNA-seq), it is possible to measure the expression dynamics of genes at the single-cell level. Through scRNA-seq, a huge amount of expression data for several thousand(s) of genes over million(s) of cells are generated in a single experiment. Differential expression analysis is the primary downstream analysis of such data to identify gene markers for cell type detection and also provide inputs to other secondary analyses. Many statistical approaches for differential expression analysis have been reported in the literature. Therefore, we critically discuss the underlying statistical principles of the approaches and distinctly divide them into six major classes, i.e., generalized linear, generalized additive, Hurdle, mixture models, two-class parametric, and non-parametric approaches. We also succinctly discuss the limitations that are specific to each class of approaches, and how they are addressed by other subsequent classes of approach. A number of challenges are identified in this study that must be addressed to develop the next class of innovative approaches. Furthermore, we also emphasize the methodological challenges involved in differential expression analysis of scRNA-seq data that researchers must address to draw maximum benefit from this recent single-cell technology. This study will serve as a guide to genome researchers and experimental biologists to objectively select options for their analysis.
Keywords: challenges; classification; differential expression analysis; scRNA-seq; statistical approaches.
Conflict of interest statement
The authors declare no conflict of interest.
Figures
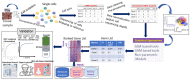
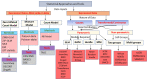
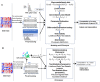
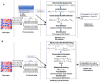
Similar articles
-
Single-Cell RNA Sequencing Analysis: A Step-by-Step Overview.Methods Mol Biol. 2021;2284:343-365. doi: 10.1007/978-1-0716-1307-8_19. Methods Mol Biol. 2021. PMID: 33835452
-
Detection of high variability in gene expression from single-cell RNA-seq profiling.BMC Genomics. 2016 Aug 22;17 Suppl 7(Suppl 7):508. doi: 10.1186/s12864-016-2897-6. BMC Genomics. 2016. PMID: 27556924 Free PMC article.
-
Improvements Achieved by Multiple Imputation for Single-Cell RNA-Seq Data in Clustering Analysis and Differential Expression Analysis.J Comput Biol. 2022 Jul;29(7):634-649. doi: 10.1089/cmb.2021.0597. Epub 2022 May 16. J Comput Biol. 2022. PMID: 35575729
-
Single-cell RNA sequencing in breast cancer: Understanding tumor heterogeneity and paving roads to individualized therapy.Cancer Commun (Lond). 2020 Aug;40(8):329-344. doi: 10.1002/cac2.12078. Epub 2020 Jul 12. Cancer Commun (Lond). 2020. PMID: 32654419 Free PMC article. Review.
-
Machine learning and statistical methods for clustering single-cell RNA-sequencing data.Brief Bioinform. 2020 Jul 15;21(4):1209-1223. doi: 10.1093/bib/bbz063. Brief Bioinform. 2020. PMID: 31243426 Review.
Cited by
-
Theoretical framework for the difference of two negative binomial distributions and its application in comparative analysis of sequencing data.Genome Res. 2024 Oct 29;34(10):1636-1650. doi: 10.1101/gr.278843.123. Genome Res. 2024. PMID: 39406498 Free PMC article.
-
Statistically principled feature selection for single cell transcriptomics.bioRxiv [Preprint]. 2024 Oct 15:2024.10.11.617709. doi: 10.1101/2024.10.11.617709. bioRxiv. 2024. PMID: 39463971 Free PMC article. Preprint.
-
A Novel Algorithm for Feature Selection Using Penalized Regression with Applications to Single-Cell RNA Sequencing Data.Biology (Basel). 2022 Oct 12;11(10):1495. doi: 10.3390/biology11101495. Biology (Basel). 2022. PMID: 36290397 Free PMC article.
-
Comparative study on differential expression analysis methods for single-cell RNA sequencing data with small biological replicates: Based on single-cell transcriptional data of PBMCs from COVID-19 severe patients.PLoS One. 2024 Mar 27;19(3):e0299358. doi: 10.1371/journal.pone.0299358. eCollection 2024. PLoS One. 2024. PMID: 38536877 Free PMC article.
-
scIALM: A method for sparse scRNA-seq expression matrix imputation using the Inexact Augmented Lagrange Multiplier with low error.Comput Struct Biotechnol J. 2024 Jan 2;23:549-558. doi: 10.1016/j.csbj.2023.12.027. eCollection 2024 Dec. Comput Struct Biotechnol J. 2024. PMID: 38274995 Free PMC article.
References
-
- Macosko E.Z., Basu A., Satija R., Nemesh J., Shekhar K., Goldman M., Tirosh I., Bialas A.R., Kamitaki N., Martersteck E.M., et al. Highly Parallel Genome-wide Expression Profiling of Individual Cells Using Nanoliter Droplets. Cell. 2015;161:1202–1214. doi: 10.1016/j.cell.2015.05.002. - DOI - PMC - PubMed
Publication types
Grants and funding
- P30 ES030283/ES/NIEHS NIH HHS/United States
- AGEDIASRISIL202101800189/ICAR-Indian Agricultural Statistics Research Institute
- CRG/2021/004960/Science and Engineering Research Board
- 5P20GM113226, PI: McClain; 1P42ES023716, PI: Srivastava; 5P30GM127607-02, PI: Jones; 1P20GM125504-01, PI: Lamont; 2U54HL120163, PI: Bhatnagar/Robertson; 1P20GM135004, PI: Yan; 1R35ES0238373-01, PI: Cave; 1R01ES029846, PI: Bhatnagar; 1R01ES027778-01A1, PI:/NH/NIH HHS/United States
LinkOut - more resources
Full Text Sources