A Physics-Guided Neural Network for Predicting Protein-Ligand Binding Free Energy: From Host-Guest Systems to the PDBbind Database
- PMID: 35883475
- PMCID: PMC9312865
- DOI: 10.3390/biom12070919
A Physics-Guided Neural Network for Predicting Protein-Ligand Binding Free Energy: From Host-Guest Systems to the PDBbind Database
Abstract
Calculation of protein-ligand binding affinity is a cornerstone of drug discovery. Classic implicit solvent models, which have been widely used to accomplish this task, lack accuracy compared to experimental references. Emerging data-driven models, on the other hand, are often accurate yet not fully interpretable and also likely to be overfitted. In this research, we explore the application of Theory-Guided Data Science in studying protein-ligand binding. A hybrid model is introduced by integrating Graph Convolutional Network (data-driven model) with the GBNSR6 implicit solvent (physics-based model). The proposed physics-data model is tested on a dataset of 368 complexes from the PDBbind refined set and 72 host-guest systems. Results demonstrate that the proposed Physics-Guided Neural Network can successfully improve the "accuracy" of the pure data-driven model. In addition, the "interpretability" and "transferability" of our model have boosted compared to the purely data-driven model. Further analyses include evaluating model robustness and understanding relationships between the physical features.
Keywords: binding free energy; graph convolutional network; implicit solvent model.
Conflict of interest statement
The authors declare no conflict of interest.
Figures
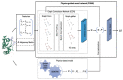
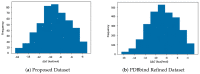
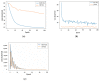
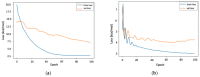
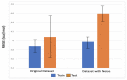
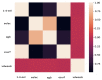
Similar articles
-
Calculation of protein-ligand binding entropies using a rule-based molecular fingerprint.Biophys J. 2024 Sep 3;123(17):2839-2848. doi: 10.1016/j.bpj.2024.03.017. Epub 2024 Mar 13. Biophys J. 2024. PMID: 38481102
-
Tuning Potential Functions to Host-Guest Binding Data.J Chem Theory Comput. 2024 Jan 9;20(1):239-252. doi: 10.1021/acs.jctc.3c01050. Epub 2023 Dec 26. J Chem Theory Comput. 2024. PMID: 38147689 Free PMC article.
-
Accuracy comparison of several common implicit solvent models and their implementations in the context of protein-ligand binding.J Mol Graph Model. 2017 Mar;72:70-80. doi: 10.1016/j.jmgm.2016.12.011. Epub 2016 Dec 21. J Mol Graph Model. 2017. PMID: 28064081 Free PMC article.
-
Overview of the SAMPL5 host-guest challenge: Are we doing better?J Comput Aided Mol Des. 2017 Jan;31(1):1-19. doi: 10.1007/s10822-016-9974-4. Epub 2016 Sep 22. J Comput Aided Mol Des. 2017. PMID: 27658802 Free PMC article. Review.
-
Pose Classification Using Three-Dimensional Atomic Structure-Based Neural Networks Applied to Ion Channel-Ligand Docking.J Chem Inf Model. 2022 May 23;62(10):2301-2315. doi: 10.1021/acs.jcim.1c01510. Epub 2022 Apr 21. J Chem Inf Model. 2022. PMID: 35447030 Free PMC article. Review.
Cited by
-
Improving the Accuracy of Physics-Based Hydration-Free Energy Predictions by Machine Learning the Remaining Error Relative to the Experiment.J Chem Theory Comput. 2024 Jan 9;20(1):396-410. doi: 10.1021/acs.jctc.3c00981. Epub 2023 Dec 27. J Chem Theory Comput. 2024. PMID: 38149593 Free PMC article.
-
Calculation of protein-ligand binding entropies using a rule-based molecular fingerprint.Biophys J. 2024 Sep 3;123(17):2839-2848. doi: 10.1016/j.bpj.2024.03.017. Epub 2024 Mar 13. Biophys J. 2024. PMID: 38481102
-
Editorial: Special Issue "Protein Modeling and Simulation: Selected Articles from the Computational Structural Bioinformatics Workshop 2021".Biomolecules. 2023 Feb 22;13(3):408. doi: 10.3390/biom13030408. Biomolecules. 2023. PMID: 36979343 Free PMC article.
-
PNBACE: an ensemble algorithm to predict the effects of mutations on protein-nucleic acid binding affinity.BMC Biol. 2024 Sep 11;22(1):203. doi: 10.1186/s12915-024-02006-9. BMC Biol. 2024. PMID: 39256728 Free PMC article.
-
Physics-Guided Deep Generative Model for New Ligand Discovery.ACM BCB. 2023 Sep;2023:10.1145/3584371.3613067. doi: 10.1145/3584371.3613067. Epub 2023 Oct 4. ACM BCB. 2023. PMID: 38706556 Free PMC article.
References
Publication types
MeSH terms
Substances
Grants and funding
LinkOut - more resources
Full Text Sources