ATRPred: A machine learning based tool for clinical decision making of anti-TNF treatment in rheumatoid arthritis patients
- PMID: 35788746
- PMCID: PMC9321399
- DOI: 10.1371/journal.pcbi.1010204
ATRPred: A machine learning based tool for clinical decision making of anti-TNF treatment in rheumatoid arthritis patients
Abstract
Rheumatoid arthritis (RA) is a chronic autoimmune condition, characterised by joint pain, damage and disability, which can be addressed in a high proportion of patients by timely use of targeted biologic treatments. However, the patients, non-responsive to the treatments often suffer from refractoriness of the disease, leading to poor quality of life. Additionally, the biologic treatments are expensive. We obtained plasma samples from N = 144 participants with RA, who were about to commence anti-tumour necrosis factor (anti-TNF) therapy. These samples were sent to Olink Proteomics, Uppsala, Sweden, where proximity extension assays of 4 panels, containing 92 proteins each, were performed. A total of n = 89 samples of patients passed the quality control of anti-TNF treatment response data. The preliminary analysis of plasma protein expression values suggested that the RA population could be divided into two distinct molecular sub-groups (endotypes). However, these broad groups did not predict response to anti-TNF treatment, but were significantly different in terms of gender and their disease activity. We then labelled these patients as responders (n = 60) and non-responders (n = 29) based on the change in disease activity score (DAS) after 6 months of anti-TNF treatment and applied machine learning (ML) with a rigorous 5-fold nested cross-validation scheme to filter 17 proteins that were significantly associated with the treatment response. We have developed a ML based classifier ATRPred (anti-TNF treatment response predictor), which can predict anti-TNF treatment response in RA patients with 81% accuracy, 75% sensitivity and 86% specificity. ATRPred may aid clinicians to direct anti-TNF therapy to patients most likely to receive benefit, thus save cost as well as prevent non-responsive patients from refractory consequences. ATRPred is implemented in R.
Conflict of interest statement
I have read the journal’s policy and the authors of this manuscript have the following competing interests: A UK-wide patent application has been filed by the Ulster University; UK Application No. 2208371.1, patent pending. All the aspects of this manuscript are covered in this patent application.
Figures
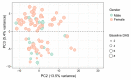
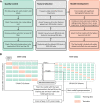
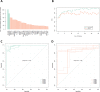
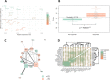
Similar articles
-
Early prediction of clinical response to anti-TNF treatment using multi-omics and machine learning in rheumatoid arthritis.Rheumatology (Oxford). 2022 Apr 11;61(4):1680-1689. doi: 10.1093/rheumatology/keab521. Rheumatology (Oxford). 2022. PMID: 34175943 Free PMC article.
-
Integrative Clinical, Molecular, and Computational Analysis Identify Novel Biomarkers and Differential Profiles of Anti-TNF Response in Rheumatoid Arthritis.Front Immunol. 2021 Mar 23;12:631662. doi: 10.3389/fimmu.2021.631662. eCollection 2021. Front Immunol. 2021. PMID: 33833756 Free PMC article.
-
Blood-based identification of non-responders to anti-TNF therapy in rheumatoid arthritis.BMC Med Genomics. 2015 Jun 3;8:26. doi: 10.1186/s12920-015-0100-6. BMC Med Genomics. 2015. PMID: 26036272 Free PMC article.
-
Economic consequences of sequencing biologics in rheumatoid arthritis: a systematic review.J Med Econ. 2013;16(3):391-6. doi: 10.3111/13696998.2013.763812. Epub 2013 Jan 18. J Med Econ. 2013. PMID: 23298329 Review.
-
Defining response to TNF-inhibitors in rheumatoid arthritis: the negative impact of anti-TNF cycling and the need for a personalized medicine approach to identify primary non-responders.Clin Rheumatol. 2019 Nov;38(11):2967-2976. doi: 10.1007/s10067-019-04684-1. Epub 2019 Sep 13. Clin Rheumatol. 2019. PMID: 31520227 Review.
Cited by
-
Unveiling Artificial Intelligence's Power: Precision, Personalization, and Progress in Rheumatology.J Clin Med. 2024 Oct 31;13(21):6559. doi: 10.3390/jcm13216559. J Clin Med. 2024. PMID: 39518698 Free PMC article. Review.
-
We asked Chat GPT to describe brain fog in chronic pain: What did we learn?Digit Health. 2023 Dec 19;9:20552076231218120. doi: 10.1177/20552076231218120. eCollection 2023 Jan-Dec. Digit Health. 2023. PMID: 39286464 Free PMC article.
-
Artificial Intelligence Driven Biomedical Image Classification for Robust Rheumatoid Arthritis Classification.Biomedicines. 2022 Oct 26;10(11):2714. doi: 10.3390/biomedicines10112714. Biomedicines. 2022. PMID: 36359235 Free PMC article.
References
-
- Caceres V. Common characteristics in RA patients who don’t respond to biologics. The Rheumatologist. 2019. Available from: https://www.the-rheumatologist.org/article/common-characteristics-in-ra-... (Accessed: 12 April 2021).
Publication types
MeSH terms
Substances
Grants and funding
LinkOut - more resources
Full Text Sources
Medical