Emerging Artificial Intelligence-Empowered mHealth: Scoping Review
- PMID: 35679107
- PMCID: PMC9227797
- DOI: 10.2196/35053
Emerging Artificial Intelligence-Empowered mHealth: Scoping Review
Abstract
Background: Artificial intelligence (AI) has revolutionized health care delivery in recent years. There is an increase in research for advanced AI techniques, such as deep learning, to build predictive models for the early detection of diseases. Such predictive models leverage mobile health (mHealth) data from wearable sensors and smartphones to discover novel ways for detecting and managing chronic diseases and mental health conditions.
Objective: Currently, little is known about the use of AI-powered mHealth (AIM) settings. Therefore, this scoping review aims to map current research on the emerging use of AIM for managing diseases and promoting health. Our objective is to synthesize research in AIM models that have increasingly been used for health care delivery in the last 2 years.
Methods: Using Arksey and O'Malley's 5-point framework for conducting scoping reviews, we reviewed AIM literature from the past 2 years in the fields of biomedical technology, AI, and information systems. We searched 3 databases, PubsOnline at INFORMS, e-journal archive at MIS Quarterly, and Association for Computing Machinery (ACM) Digital Library using keywords such as "mobile healthcare," "wearable medical sensors," "smartphones", and "AI." We included AIM articles and excluded technical articles focused only on AI models. We also used the PRISMA (Preferred Reporting Items for Systematic Reviews and Meta-Analyses) technique for identifying articles that represent a comprehensive view of current research in the AIM domain.
Results: We screened 108 articles focusing on developing AIM models for ensuring better health care delivery, detecting diseases early, and diagnosing chronic health conditions, and 37 articles were eligible for inclusion, with 31 of the 37 articles being published last year (76%). Of the included articles, 9 studied AI models to detect serious mental health issues, such as depression and suicidal tendencies, and chronic health conditions, such as sleep apnea and diabetes. Several articles discussed the application of AIM models for remote patient monitoring and disease management. The considered primary health concerns belonged to 3 categories: mental health, physical health, and health promotion and wellness. Moreover, 14 of the 37 articles used AIM applications to research physical health, representing 38% of the total studies. Finally, 28 out of the 37 (76%) studies used proprietary data sets rather than public data sets. We found a lack of research in addressing chronic mental health issues and a lack of publicly available data sets for AIM research.
Conclusions: The application of AIM models for disease detection and management is a growing research domain. These models provide accurate predictions for enabling preventive care on a broader scale in the health care domain. Given the ever-increasing need for remote disease management during the pandemic, recent AI techniques, such as federated learning and explainable AI, can act as a catalyst for increasing the adoption of AIM and enabling secure data sharing across the health care industry.
Keywords: artificial intelligence; machine learning; mobile health units; review literature as topic; telemedicine.
©Paras Bhatt, Jia Liu, Yanmin Gong, Jing Wang, Yuanxiong Guo. Originally published in JMIR mHealth and uHealth (https://mhealth.jmir.org), 09.06.2022.
Conflict of interest statement
Conflicts of Interest: None declared.
Figures
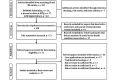
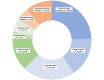
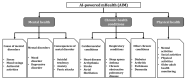
Similar articles
-
Ethics of Procuring and Using Organs or Tissue from Infants and Newborns for Transplantation, Research, or Commercial Purposes: Protocol for a Bioethics Scoping Review.Wellcome Open Res. 2024 Dec 5;9:717. doi: 10.12688/wellcomeopenres.23235.1. eCollection 2024. Wellcome Open Res. 2024. PMID: 39839977 Free PMC article.
-
Applications of Federated Learning in Mobile Health: Scoping Review.J Med Internet Res. 2023 May 1;25:e43006. doi: 10.2196/43006. J Med Internet Res. 2023. PMID: 37126398 Free PMC article.
-
Artificial intelligence technologies and compassion in healthcare: A systematic scoping review.Front Psychol. 2023 Jan 17;13:971044. doi: 10.3389/fpsyg.2022.971044. eCollection 2022. Front Psychol. 2023. PMID: 36733854 Free PMC article.
-
Exploring conceptual and theoretical frameworks for nurse practitioner education: a scoping review protocol.JBI Database System Rev Implement Rep. 2015 Oct;13(10):146-55. doi: 10.11124/jbisrir-2015-2150. JBI Database System Rev Implement Rep. 2015. PMID: 26571290
-
Data Preprocessing Techniques for AI and Machine Learning Readiness: Scoping Review of Wearable Sensor Data in Cancer Care.JMIR Mhealth Uhealth. 2024 Sep 27;12:e59587. doi: 10.2196/59587. JMIR Mhealth Uhealth. 2024. PMID: 38626290 Free PMC article.
Cited by
-
Exploring Digital Biomarkers of Illness Activity in Mood Episodes: Hypotheses Generating and Model Development Study.JMIR Mhealth Uhealth. 2023 May 4;11:e45405. doi: 10.2196/45405. JMIR Mhealth Uhealth. 2023. PMID: 36939345 Free PMC article.
-
Just-in-Time Adaptive Intervention for Stabilizing Sleep Hours of Japanese Workers: Microrandomized Trial.J Med Internet Res. 2024 Jun 11;26:e49669. doi: 10.2196/49669. J Med Internet Res. 2024. PMID: 38861313 Free PMC article. Clinical Trial.
-
Diagnosis and management of subarachnoid haemorrhage.Nat Commun. 2024 Feb 29;15(1):1850. doi: 10.1038/s41467-024-46015-2. Nat Commun. 2024. PMID: 38424037 Free PMC article. Review.
-
The potential impact of ChatGPT in clinical and translational medicine.Clin Transl Med. 2023 Mar;13(3):e1216. doi: 10.1002/ctm2.1216. Clin Transl Med. 2023. PMID: 36856370 Free PMC article. No abstract available.
-
Translating Suicide Safety Planning Components Into the Design of mHealth App Features: Systematic Review.JMIR Ment Health. 2024 Mar 28;11:e52763. doi: 10.2196/52763. JMIR Ment Health. 2024. PMID: 38546711 Free PMC article.
References
-
- Raghupathi W, Raghupathi V. Big data analytics in healthcare: promise and potential. Health Inf Sci Syst. 2014;2:3. doi: 10.1186/2047-2501-2-3. https://hissjournal.biomedcentral.com/articles/10.1186/2047-2501-2-3 14 - DOI - DOI - PMC - PubMed
-
- Valanarasu R. Smart and secure IoT and AI integration framework for hospital environment. JISMAC. 2019 Nov;01(03):172–179. doi: 10.36548/jismac.2019.3.004. - DOI
-
- Barrett M, Boyne J, Brandts J, Brunner-La Rocca H, De Maesschalck L, De Wit K, Dixon L, Eurlings C, Fitzsimons D, Golubnitschaja O, Hageman A, Heemskerk F, Hintzen A, Helms TM, Hill L, Hoedemakers T, Marx N, McDonald K, Mertens M, Müller-Wieland Dirk, Palant A, Piesk J, Pomazanskyi A, Ramaekers J, Ruff P, Schütt Katharina, Shekhawat Y, Ski CF, Thompson DR, Tsirkin A, van der Mierden K, Watson C, Zippel-Schultz B. Artificial intelligence supported patient self-care in chronic heart failure: a paradigm shift from reactive to predictive, preventive and personalised care. EPMA J. 2019 Dec;10(4):445–464. doi: 10.1007/s13167-019-00188-9. http://europepmc.org/abstract/MED/31832118 188 - DOI - PMC - PubMed
-
- Guillodo E, Lemey C, Simonnet M, Walter M, Baca-García Enrique, Masetti V, Moga S, Larsen M, HUGOPSY Network. Ropars J, Berrouiguet S. Clinical applications of mobile health wearable-based sleep monitoring: systematic review. JMIR Mhealth Uhealth. 2020 Apr 01;8(4):e10733. doi: 10.2196/10733. https://mhealth.jmir.org/2020/4/e10733/ v8i4e10733 - DOI - PMC - PubMed
Publication types
MeSH terms
LinkOut - more resources
Full Text Sources
Medical
Miscellaneous