Integration of the Salmonella Typhimurium Methylome and Transcriptome Reveals That DNA Methylation and Transcriptional Regulation Are Largely Decoupled under Virulence-Related Conditions
- PMID: 35658533
- PMCID: PMC9239280
- DOI: 10.1128/mbio.03464-21
Integration of the Salmonella Typhimurium Methylome and Transcriptome Reveals That DNA Methylation and Transcriptional Regulation Are Largely Decoupled under Virulence-Related Conditions
Abstract
Despite being in a golden age of bacterial epigenomics, little work has systematically examined the plasticity and functional impacts of the bacterial DNA methylome. Here, we leveraged single-molecule, real-time sequencing (SMRT-seq) to examine the m6A DNA methylome of two Salmonella enterica serovar Typhimurium strains: 14028s and a ΔmetJ mutant with derepressed methionine metabolism, grown in Luria broth or medium that simulates the intracellular environment. We found that the methylome is remarkably static: >95% of adenosine bases retain their methylation status across conditions. Integration of methylation with transcriptomic data revealed limited correlation between changes in methylation and gene expression. Further, examination of the transcriptome in ΔyhdJ bacteria lacking the m6A methylase with the most dynamic methylation pattern in our data set revealed little evidence of YhdJ-mediated gene regulation. Curiously, despite G(m6A)TC motifs being particularly resistant to change across conditions, incorporating dam mutants into our analyses revealed two examples where changes in methylation and transcription may be linked across conditions. This includes the novel finding that the ΔmetJ motility defect may be partially driven by hypermethylation of the chemotaxis gene tsr. Together, these data redefine the S. Typhimurium epigenome as a highly stable system that has rare but important roles in transcriptional regulation. Incorporating these lessons into future studies will be critical as we progress through the epigenomic era. IMPORTANCE While recent breakthroughs have enabled intense study of bacterial DNA modifications, limitations in current work have potentiated a surprisingly untested narrative that DNA methylation is a common mechanism of the bacterial response to environmental conditions. Essentially, whether epigenetic regulation of bacterial transcription is a common, generalizable phenomenon is a critical unanswered question that we address here. We found that most DNA methylation is static in Salmonella enterica serovar Typhimurium, even when the bacteria are grown under dramatically different conditions that cause broad changes in the transcriptome. Further, even when the methylation of individual bases change, these changes generally do not correlate with changes in gene expression. Finally, we demonstrate methods by which data can be stratified in order to identify coupled changes in methylation and gene expression.
Keywords: DNA methylation; Salmonella; gene regulation; m6A; methylome; transcription.
Conflict of interest statement
The authors declare no conflict of interest.
Figures
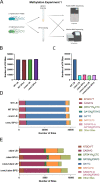
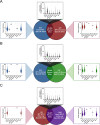
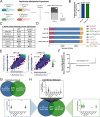
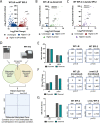
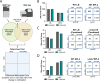
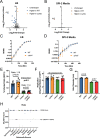
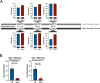
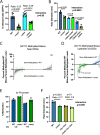
Similar articles
-
Integrative DNA methylome and transcriptome analysis reveals DNA adenine methylation is involved in Salmonella enterica Typhimurium response to oxidative stress.Microbiol Spectr. 2023 Dec 12;11(6):e0247923. doi: 10.1128/spectrum.02479-23. Epub 2023 Oct 26. Microbiol Spectr. 2023. PMID: 37882553 Free PMC article.
-
DNA adenine methylation regulates virulence gene expression in Salmonella enterica serovar Typhimurium.J Bacteriol. 2006 Dec;188(23):8160-8. doi: 10.1128/JB.00847-06. Epub 2006 Sep 22. J Bacteriol. 2006. PMID: 16997949 Free PMC article.
-
Regulation of the Salmonella enterica std fimbrial operon by DNA adenine methylation, SeqA, and HdfR.J Bacteriol. 2008 Nov;190(22):7406-13. doi: 10.1128/JB.01136-08. Epub 2008 Sep 19. J Bacteriol. 2008. PMID: 18805972 Free PMC article.
-
The challenge of relating gene expression to the virulence of Salmonella enterica serovar Typhimurium.Curr Opin Biotechnol. 2011 Apr;22(2):200-10. doi: 10.1016/j.copbio.2011.02.007. Epub 2011 Mar 22. Curr Opin Biotechnol. 2011. PMID: 21388802 Review.
-
The Helicobacter pylori Methylome: Roles in Gene Regulation and Virulence.Curr Top Microbiol Immunol. 2017;400:105-127. doi: 10.1007/978-3-319-50520-6_5. Curr Top Microbiol Immunol. 2017. PMID: 28124151 Review.
Cited by
-
Changes in DNA methylation contribute to rapid adaptation in bacterial plant pathogen evolution.PLoS Biol. 2024 Sep 20;22(9):e3002792. doi: 10.1371/journal.pbio.3002792. eCollection 2024 Sep. PLoS Biol. 2024. PMID: 39302959 Free PMC article.
-
Diverse regulatory pathways modulate bet hedging of competence induction in epigenetically-differentiated phase variants of Streptococcus pneumoniae.Nucleic Acids Res. 2023 Oct 27;51(19):10375-10394. doi: 10.1093/nar/gkad760. Nucleic Acids Res. 2023. PMID: 37757859 Free PMC article.
-
Diverse Roles for a Conserved DNA-Methyltransferase in the Entomopathogenic Bacterium Xenorhabdus.Int J Mol Sci. 2022 Oct 9;23(19):11981. doi: 10.3390/ijms231911981. Int J Mol Sci. 2022. PMID: 36233296 Free PMC article.
-
Genome-wide identification of genes critical for in vivo fitness of multi-drug resistant porcine extraintestinal pathogenic Escherichia coli by transposon-directed insertion site sequencing using a mouse infection model.Virulence. 2023 Dec;14(1):2158708. doi: 10.1080/21505594.2022.2158708. Virulence. 2023. PMID: 36537189 Free PMC article.
References
Publication types
MeSH terms
Substances
Grants and funding
LinkOut - more resources
Full Text Sources
Research Materials