Parkinson's Disease Detection from Resting-State EEG Signals Using Common Spatial Pattern, Entropy, and Machine Learning Techniques
- PMID: 35626189
- PMCID: PMC9139946
- DOI: 10.3390/diagnostics12051033
Parkinson's Disease Detection from Resting-State EEG Signals Using Common Spatial Pattern, Entropy, and Machine Learning Techniques
Abstract
Parkinson's disease (PD) is a very common brain abnormality that affects people all over the world. Early detection of such abnormality is critical in clinical diagnosis in order to prevent disease progression. Electroencephalography (EEG) is one of the most important PD diagnostic tools since this disease is linked to the brain. In this study, novel efficient common spatial pattern-based approaches for detecting Parkinson's disease in two cases, off-medication and on-medication, are proposed. First, the EEG signals are preprocessed to remove major artifacts before spatial filtering using a common spatial pattern. Several features are extracted from spatially filtered signals using different metrics, namely, variance, band power, energy, and several types of entropy. Machine learning techniques, namely, random forest, linear/quadratic discriminant analysis, support vector machine, and k-nearest neighbor, are investigated to classify the extracted features. The impacts of frequency bands, segment length, and reduction number on the results are also investigated in this work. The proposed methods are tested using two EEG datasets: the SanDiego dataset (31 participants, 93 min) and the UNM dataset (54 participants, 54 min). The results show that the proposed methods, particularly the combination of common spatial patterns and log energy entropy, provide competitive results when compared to methods in the literature. The achieved results in terms of classification accuracy, sensitivity, and specificity in the case of off-medication PD detection are around 99%. In the case of on-medication PD, the results range from 95% to 98%. The results also reveal that features extracted from the alpha and beta bands have the highest classification accuracy.
Keywords: Parkinson’s detection; common spatial pattern; discriminant analysis; electroencephalogram; entropy; k-nearest neighbor; machine learning; random forest; support vector machines.
Conflict of interest statement
The authors declare no conflict of interest.
Figures
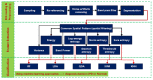
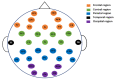
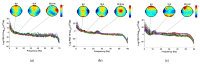
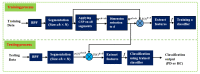
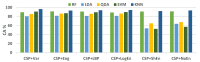
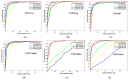
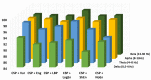
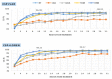
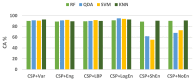
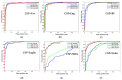
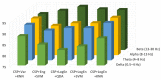
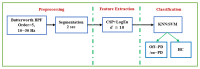
Similar articles
-
Detection of Parkinson's disease from EEG signals using discrete wavelet transform, different entropy measures, and machine learning techniques.Sci Rep. 2022 Dec 29;12(1):22547. doi: 10.1038/s41598-022-26644-7. Sci Rep. 2022. PMID: 36581646 Free PMC article.
-
Diagnosis and classification of Parkinson's disease using ensemble learning and 1D-PDCovNN.Comput Biol Med. 2023 Jul;161:107031. doi: 10.1016/j.compbiomed.2023.107031. Epub 2023 May 17. Comput Biol Med. 2023. PMID: 37211002
-
EEG Signal Analysis for Diagnosing Neurological Disorders Using Discrete Wavelet Transform and Intelligent Techniques.Sensors (Basel). 2020 Apr 28;20(9):2505. doi: 10.3390/s20092505. Sensors (Basel). 2020. PMID: 32354161 Free PMC article.
-
A review of emergent intelligent systems for the detection of Parkinson's disease.Biomed Eng Lett. 2023 Sep 20;13(4):591-612. doi: 10.1007/s13534-023-00319-2. eCollection 2023 Nov. Biomed Eng Lett. 2023. PMID: 37872986 Free PMC article. Review.
-
Imperative Role of Machine Learning Algorithm for Detection of Parkinson's Disease: Review, Challenges and Recommendations.Diagnostics (Basel). 2022 Aug 19;12(8):2003. doi: 10.3390/diagnostics12082003. Diagnostics (Basel). 2022. PMID: 36010353 Free PMC article. Review.
Cited by
-
Detection of Parkinson's disease from EEG signals using discrete wavelet transform, different entropy measures, and machine learning techniques.Sci Rep. 2022 Dec 29;12(1):22547. doi: 10.1038/s41598-022-26644-7. Sci Rep. 2022. PMID: 36581646 Free PMC article.
-
Automatic and Early Detection of Parkinson's Disease by Analyzing Acoustic Signals Using Classification Algorithms Based on Recursive Feature Elimination Method.Diagnostics (Basel). 2023 May 31;13(11):1924. doi: 10.3390/diagnostics13111924. Diagnostics (Basel). 2023. PMID: 37296776 Free PMC article.
-
Parkinson's disease detection from EEG signal employing autoencoder and RBFNN-based hybrid deep learning framework utilizing power spectral density.Digit Health. 2024 Nov 12;10:20552076241297355. doi: 10.1177/20552076241297355. eCollection 2024 Jan-Dec. Digit Health. 2024. PMID: 39539721 Free PMC article.
-
EEG-Based Detection of Mild Cognitive Impairment Using DWT-Based Features and Optimization Methods.Diagnostics (Basel). 2024 Jul 26;14(15):1619. doi: 10.3390/diagnostics14151619. Diagnostics (Basel). 2024. PMID: 39125495 Free PMC article.
-
Evaluation of Parkinson's disease early diagnosis using single-channel EEG features and auditory cognitive assessment.Front Neurol. 2023 Dec 19;14:1273458. doi: 10.3389/fneur.2023.1273458. eCollection 2023. Front Neurol. 2023. PMID: 38174098 Free PMC article.
References
-
- National Institutes of Health (U.S.) Stem Cells: Scientific Progress and Future Research Directions. University Press of the Pacific; Honolulu, HI, USA: 2005.
-
- World Health Organization . Neurological Disorders: Public Health Challenges. WHO Press; Geneva, Switzerland: 2006. p. 177.
LinkOut - more resources
Full Text Sources
Other Literature Sources