Unusual mammalian usage of TGA stop codons reveals that sequence conservation need not imply purifying selection
- PMID: 35550630
- PMCID: PMC9129041
- DOI: 10.1371/journal.pbio.3001588
Unusual mammalian usage of TGA stop codons reveals that sequence conservation need not imply purifying selection
Abstract
The assumption that conservation of sequence implies the action of purifying selection is central to diverse methodologies to infer functional importance. GC-biased gene conversion (gBGC), a meiotic mismatch repair bias strongly favouring GC over AT, can in principle mimic the action of selection, this being thought to be especially important in mammals. As mutation is GC→AT biased, to demonstrate that gBGC does indeed cause false signals requires evidence that an AT-rich residue is selectively optimal compared to its more GC-rich allele, while showing also that the GC-rich alternative is conserved. We propose that mammalian stop codon evolution provides a robust test case. Although in most taxa TAA is the optimal stop codon, TGA is both abundant and conserved in mammalian genomes. We show that this mammalian exceptionalism is well explained by gBGC mimicking purifying selection and that TAA is the selectively optimal codon. Supportive of gBGC, we observe (i) TGA usage trends are consistent at the focal stop codon and elsewhere (in UTR sequences); (ii) that higher TGA usage and higher TAA→TGA substitution rates are predicted by a high recombination rate; and (iii) across species the difference in TAA <-> TGA substitution rates between GC-rich and GC-poor genes is largest in genomes that possess higher between-gene GC variation. TAA optimality is supported both by enrichment in highly expressed genes and trends associated with effective population size. High TGA usage and high TAA→TGA rates in mammals are thus consistent with gBGC's predicted ability to "drive" deleterious mutations and supports the hypothesis that sequence conservation need not be indicative of purifying selection. A general trend for GC-rich trinucleotides to reside at frequencies far above their mutational equilibrium in high recombining domains supports the generality of these results.
Conflict of interest statement
The authors have declared that no competing interests exist.
Figures
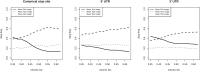
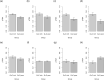
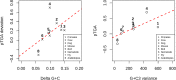
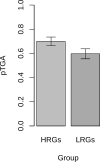
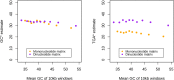
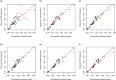
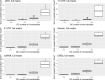
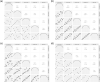
Similar articles
-
Stop Codon Usage as a Window into Genome Evolution: Mutation, Selection, Biased Gene Conversion and the TAG Paradox.Genome Biol Evol. 2022 Aug 3;14(8):evac115. doi: 10.1093/gbe/evac115. Genome Biol Evol. 2022. PMID: 35867377 Free PMC article.
-
GC-biased gene conversion and selection affect GC content in the Oryza genus (rice).Mol Biol Evol. 2011 Sep;28(9):2695-706. doi: 10.1093/molbev/msr104. Epub 2011 Apr 18. Mol Biol Evol. 2011. PMID: 21504892
-
Stop codons in bacteria are not selectively equivalent.Biol Direct. 2012 Sep 13;7:30. doi: 10.1186/1745-6150-7-30. Biol Direct. 2012. PMID: 22974057 Free PMC article.
-
Biased gene conversion and the evolution of mammalian genomic landscapes.Annu Rev Genomics Hum Genet. 2009;10:285-311. doi: 10.1146/annurev-genom-082908-150001. Annu Rev Genomics Hum Genet. 2009. PMID: 19630562 Review.
-
Codon usage and codon pair patterns in non-grass monocot genomes.Ann Bot. 2017 Nov 28;120(6):893-909. doi: 10.1093/aob/mcx112. Ann Bot. 2017. PMID: 29155926 Free PMC article. Review.
Cited by
-
Selection on synonymous sites: the unwanted transcript hypothesis.Nat Rev Genet. 2024 Jun;25(6):431-448. doi: 10.1038/s41576-023-00686-7. Epub 2024 Jan 31. Nat Rev Genet. 2024. PMID: 38297070 Review.
-
Stop Codon Usage as a Window into Genome Evolution: Mutation, Selection, Biased Gene Conversion and the TAG Paradox.Genome Biol Evol. 2022 Aug 3;14(8):evac115. doi: 10.1093/gbe/evac115. Genome Biol Evol. 2022. PMID: 35867377 Free PMC article.
-
Mitochondrial genomes of Macropsini (Hemiptera: Cicadellidae: Eurymelinae): Structural features, codon usage patterns, and phylogenetic implications.Ecol Evol. 2024 Sep 10;14(9):e70268. doi: 10.1002/ece3.70268. eCollection 2024 Sep. Ecol Evol. 2024. PMID: 39263460 Free PMC article.
-
Genes for highly abundant proteins in Escherichia coli avoid 5' codons that promote ribosomal initiation.PLoS Comput Biol. 2023 Oct 25;19(10):e1011581. doi: 10.1371/journal.pcbi.1011581. eCollection 2023 Oct. PLoS Comput Biol. 2023. PMID: 37878567 Free PMC article.
-
The Patterns of Codon Usage between Chordates and Arthropods are Different but Co-evolving with Mutational Biases.Mol Biol Evol. 2024 May 3;41(5):msae080. doi: 10.1093/molbev/msae080. Mol Biol Evol. 2024. PMID: 38667829 Free PMC article.
References
Publication types
MeSH terms
Substances
Grants and funding
LinkOut - more resources
Full Text Sources
Research Materials
Miscellaneous