Alternative polyadenylation associated with prognosis and therapy in colorectal cancer
- PMID: 35487956
- PMCID: PMC9054804
- DOI: 10.1038/s41598-022-11089-9
Alternative polyadenylation associated with prognosis and therapy in colorectal cancer
Abstract
Colorectal cancer (CRC) is among the most widely spread cancers globally. Aberrant alternative polyadenylation (APA) plays a role in cancer onset and its progression. Consequently, this study focused on highlighting the role of APA events and signals in the prognosis of patients with CRC. The APA events, RNA sequencing (RNA-seq), somatic mutations, copy number variants (CNVs), and clinical information of the CRC cohort were obtained from The Cancer Genome Atlas (TCGA) database and UCSC (University of California-Santa Cruz) Xena database. The whole set was sorted into two sets: a training set and a test set in a ratio of 7:3. 197 prognosis-related APA events were collected by performing univariate Cox regression signature in patients with CRC. Subsequently, a signature for APA events was established by least absolute shrinkage and selection operator (LASSO) and multivariate Cox analysis. The risk scores were measured for individual patients on the basis of the signature and patients were sorted into two groups; the high-risk group and the low-risk group as per their median risk scores. Kaplan-Meier curves, principal component analysis (PCA), and time-dependent receiver operator characteristic (ROC) curves revealed that the signature was able to predict patient prognosis effectively and further validation was provided in the test set and the whole set. The high-risk and low-risk groups displayed various distributions of mutations and CNVs. Tumor mutation burden (TMB) alone and in combination with the signature predicted the prognosis of CRC patients, but the gene frequencies of TMBs and CNVs did not change in the low- and high-risk groups. Moreover, immunotherapy and chemotherapy treatments showed different responses to PD-1 inhibitors and multiple chemotherapeutic agents in the low and high-risk groups based on the tumor immune dysfunction and exclusion (TIDE) and genomics of drugs sensitivity in cancer (GDSC) databases. This study may help in understanding the potential roles of APA in CRC, and the signature for prognosis-related APA events can work as a potential predictor for survival and treatment in patients with CRC.
© 2022. The Author(s).
Conflict of interest statement
The authors declare no competing interests.
Figures
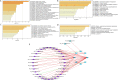
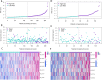
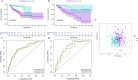
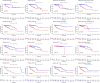
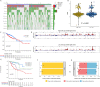
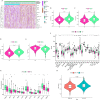
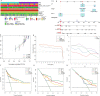
Similar articles
-
A mitophagy-related gene signature associated with prognosis and immune microenvironment in colorectal cancer.Sci Rep. 2022 Nov 4;12(1):18688. doi: 10.1038/s41598-022-23463-8. Sci Rep. 2022. PMID: 36333388 Free PMC article.
-
Establishment of a circular RNA regulatory stemness-related gene pair signature for predicting prognosis and therapeutic response in colorectal cancer.Front Immunol. 2022 Jul 25;13:934124. doi: 10.3389/fimmu.2022.934124. eCollection 2022. Front Immunol. 2022. PMID: 35958575 Free PMC article.
-
Identification and validation of an immune prognostic signature in colorectal cancer.Int Immunopharmacol. 2020 Nov;88:106868. doi: 10.1016/j.intimp.2020.106868. Epub 2020 Aug 6. Int Immunopharmacol. 2020. PMID: 32771948
-
A CLRN3-Based CD8+ T-Related Gene Signature Predicts Prognosis and Immunotherapy Response in Colorectal Cancer.Biomolecules. 2024 Jul 24;14(8):891. doi: 10.3390/biom14080891. Biomolecules. 2024. PMID: 39199281 Free PMC article.
-
A new signature based on alternative polyadenylation for prognostic prediction and therapeutic responses in low-grade glioma.Aging (Albany NY). 2022 Jan 18;14(2):826-844. doi: 10.18632/aging.203844. Epub 2022 Jan 18. Aging (Albany NY). 2022. PMID: 35042833 Free PMC article.
Cited by
-
Multiplexed screening reveals how cancer-specific alternative polyadenylation shapes tumor growth in vivo.Nat Commun. 2024 Feb 1;15(1):959. doi: 10.1038/s41467-024-44931-x. Nat Commun. 2024. PMID: 38302465 Free PMC article.
-
Comprehensive Analysis of Alternative Polyadenylation Events Associated with the Tumor Immune Microenvironment in Colon Adenocarcinoma.Curr Genomics. 2023 Jun 23;24(1):48-61. doi: 10.2174/1389202924666230503122134. Curr Genomics. 2023. PMID: 37920728 Free PMC article.
-
Molecular signature to predict quality of life and survival with glioblastoma using Multiview omics model.PLoS One. 2023 Nov 16;18(11):e0287448. doi: 10.1371/journal.pone.0287448. eCollection 2023. PLoS One. 2023. PMID: 37972206 Free PMC article.
-
Post-transcriptional control drives Aurora kinase A expression in human cancers.PLoS One. 2024 Nov 11;19(11):e0310625. doi: 10.1371/journal.pone.0310625. eCollection 2024. PLoS One. 2024. PMID: 39527514 Free PMC article.
References
Publication types
MeSH terms
LinkOut - more resources
Full Text Sources
Medical