Comparison of five supervised feature selection algorithms leading to top features and gene signatures from multi-omics data in cancer
- PMID: 35484501
- PMCID: PMC9052461
- DOI: 10.1186/s12859-022-04678-y
Comparison of five supervised feature selection algorithms leading to top features and gene signatures from multi-omics data in cancer
Abstract
Background: As many complex omics data have been generated during the last two decades, dimensionality reduction problem has been a challenging issue in better mining such data. The omics data typically consists of many features. Accordingly, many feature selection algorithms have been developed. The performance of those feature selection methods often varies by specific data, making the discovery and interpretation of results challenging.
Methods and results: In this study, we performed a comprehensive comparative study of five widely used supervised feature selection methods (mRMR, INMIFS, DFS, SVM-RFE-CBR and VWMRmR) for multi-omics datasets. Specifically, we used five representative datasets: gene expression (Exp), exon expression (ExpExon), DNA methylation (hMethyl27), copy number variation (Gistic2), and pathway activity dataset (Paradigm IPLs) from a multi-omics study of acute myeloid leukemia (LAML) from The Cancer Genome Atlas (TCGA). The different feature subsets selected by the aforesaid five different feature selection algorithms are assessed using three evaluation criteria: (1) classification accuracy (Acc), (2) representation entropy (RE) and (3) redundancy rate (RR). Four different classifiers, viz., C4.5, NaiveBayes, KNN, and AdaBoost, were used to measure the classification accuary (Acc) for each selected feature subset. The VWMRmR algorithm obtains the best Acc for three datasets (ExpExon, hMethyl27 and Paradigm IPLs). The VWMRmR algorithm offers the best RR (obtained using normalized mutual information) for three datasets (Exp, Gistic2 and Paradigm IPLs), while it gives the best RR (obtained using Pearson correlation coefficient) for two datasets (Gistic2 and Paradigm IPLs). It also obtains the best RE for three datasets (Exp, Gistic2 and Paradigm IPLs). Overall, the VWMRmR algorithm yields best performance for all three evaluation criteria for majority of the datasets. In addition, we identified signature genes using supervised learning collected from the overlapped top feature set among five feature selection methods. We obtained a 7-gene signature (ZMIZ1, ENG, FGFR1, PAWR, KRT17, MPO and LAT2) for EXP, a 9-gene signature for ExpExon, a 7-gene signature for hMethyl27, one single-gene signature (PIK3CG) for Gistic2 and a 3-gene signature for Paradigm IPLs.
Conclusion: We performed a comprehensive comparison of the performance evaluation of five well-known feature selection methods for mining features from various high-dimensional datasets. We identified signature genes using supervised learning for the specific omic data for the disease. The study will help incorporate higher order dependencies among features.
Keywords: Classifier; Feature selection; Multi-omics data; Redundancy rate; Representation entropy.
© 2022. The Author(s).
Conflict of interest statement
The authors declare that they have no competing interests.
Figures
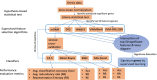
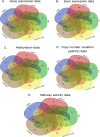
Similar articles
-
Unsupervised Feature Selection Using an Integrated Strategy of Hierarchical Clustering With Singular Value Decomposition: An Integrative Biomarker Discovery Method With Application to Acute Myeloid Leukemia.IEEE/ACM Trans Comput Biol Bioinform. 2022 May-Jun;19(3):1354-1364. doi: 10.1109/TCBB.2021.3110989. Epub 2022 Jun 3. IEEE/ACM Trans Comput Biol Bioinform. 2022. PMID: 34495838
-
Min-redundancy and max-relevance multi-view feature selection for predicting ovarian cancer survival using multi-omics data.BMC Med Genomics. 2018 Sep 14;11(Suppl 3):71. doi: 10.1186/s12920-018-0388-0. BMC Med Genomics. 2018. PMID: 30255801 Free PMC article.
-
Computer-assisted lip diagnosis on Traditional Chinese Medicine using multi-class support vector machines.BMC Complement Altern Med. 2012 Aug 16;12:127. doi: 10.1186/1472-6882-12-127. BMC Complement Altern Med. 2012. PMID: 22898352 Free PMC article.
-
Prediction Performance of Feature Selectors and Classifiers on Highly Dimensional Transcriptomic Data for Prediction of Weight Loss in Filipino Americans at Risk for Type 2 Diabetes.Biol Res Nurs. 2023 Jul;25(3):393-403. doi: 10.1177/10998004221147513. Epub 2023 Jan 4. Biol Res Nurs. 2023. PMID: 36600204 Free PMC article. Review.
-
Survey on Exact kNN Queries over High-Dimensional Data Space.Sensors (Basel). 2023 Jan 5;23(2):629. doi: 10.3390/s23020629. Sensors (Basel). 2023. PMID: 36679422 Free PMC article. Review.
Cited by
-
Review of feature selection approaches based on grouping of features.PeerJ. 2023 Jul 17;11:e15666. doi: 10.7717/peerj.15666. eCollection 2023. PeerJ. 2023. PMID: 37483989 Free PMC article. Review.
-
GradWise: A Novel Application of a Rank-Based Weighted Hybrid Filter and Embedded Feature Selection Method for Glioma Grading with Clinical and Molecular Characteristics.Cancers (Basel). 2023 Sep 19;15(18):4628. doi: 10.3390/cancers15184628. Cancers (Basel). 2023. PMID: 37760597 Free PMC article.
-
ZMIZ1 Regulates Proliferation, Autophagy and Apoptosis of Colon Cancer Cells by Mediating Ubiquitin-Proteasome Degradation of SIRT1.Biochem Genet. 2024 Aug;62(4):3245-3259. doi: 10.1007/s10528-023-10573-9. Epub 2024 Jan 12. Biochem Genet. 2024. PMID: 38214831 Free PMC article.
-
Logistic PCA explains differences between genome-scale metabolic models in terms of metabolic pathways.PLoS Comput Biol. 2024 Jun 24;20(6):e1012236. doi: 10.1371/journal.pcbi.1012236. eCollection 2024 Jun. PLoS Comput Biol. 2024. PMID: 38913731 Free PMC article.
-
Analyzing Wav2Vec 1.0 Embeddings for Cross-Database Parkinson's Disease Detection and Speech Features Extraction.Sensors (Basel). 2024 Aug 26;24(17):5520. doi: 10.3390/s24175520. Sensors (Basel). 2024. PMID: 39275431 Free PMC article.
References
-
- Maulik U, Bandyopadhyay S, Wang JTL. Computational intelligence and pattern analysis in biological informatics. Singapore: Wiley; 2010.
-
- Blum AL, Langley P. Selection of relevant features and examples in machine learning. Artif Intell. 1997;97(1–2):245–271. doi: 10.1016/S0004-3702(97)00063-5. - DOI
MeSH terms
Grants and funding
LinkOut - more resources
Full Text Sources
Research Materials
Miscellaneous