Aberrant newborn T cell and microbiota developmental trajectories predict respiratory compromise during infancy
- PMID: 35310935
- PMCID: PMC8931366
- DOI: 10.1016/j.isci.2022.104007
Aberrant newborn T cell and microbiota developmental trajectories predict respiratory compromise during infancy
Abstract
Neonatal immune-microbiota co-development is poorly understood, yet age-appropriate recognition of - and response to - pathogens and commensal microbiota is critical to health. In this longitudinal study of 148 preterm and 119 full-term infants from birth through one year of age, we found that postmenstrual age or weeks from conception is a central factor influencing T cell and mucosal microbiota development. Numerous features of the T cell and microbiota functional development remain unexplained; however, by either age metric and are instead shaped by discrete perinatal and postnatal events. Most strikingly, we establish that prenatal antibiotics or infection disrupt the normal T cell population developmental trajectory, influencing subsequent respiratory microbial colonization and predicting respiratory morbidity. In this way, early exposures predict the postnatal immune-microbiota axis trajectory, placing infants at later risk for respiratory morbidity in early childhood.
Keywords: Immunology; Microbiome.
© 2022 The Author(s).
Conflict of interest statement
The authors declare no competing interests.
Figures
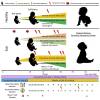
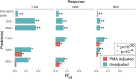
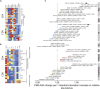
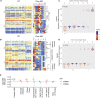
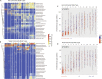
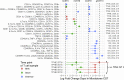
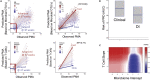
Similar articles
-
Bacteroides abundance drives birth mode dependent infant gut microbiota developmental trajectories.Front Microbiol. 2022 Oct 6;13:953475. doi: 10.3389/fmicb.2022.953475. eCollection 2022. Front Microbiol. 2022. PMID: 36274732 Free PMC article.
-
Antibiotic exposure prevents acquisition of beneficial metabolic functions in the preterm infant gut microbiome.Microbiome. 2022 Jul 7;10(1):103. doi: 10.1186/s40168-022-01300-4. Microbiome. 2022. PMID: 35794664 Free PMC article.
-
Perinatal Antibiotic Exposure Affects the Transmission between Maternal and Neonatal Microbiota and Is Associated with Early-Onset Sepsis.mSphere. 2020 Feb 19;5(1):e00984-19. doi: 10.1128/mSphere.00984-19. mSphere. 2020. PMID: 32075882 Free PMC article.
-
Nasal Microbiome and Its Interaction with the Host in Childhood Asthma.Cells. 2022 Oct 7;11(19):3155. doi: 10.3390/cells11193155. Cells. 2022. PMID: 36231116 Free PMC article. Review.
-
Factors influencing gastrointestinal tract and microbiota immune interaction in preterm infants.Pediatr Res. 2015 Jun;77(6):726-31. doi: 10.1038/pr.2015.54. Epub 2015 Mar 11. Pediatr Res. 2015. PMID: 25760550 Review.
Cited by
-
Microbiota-derived inosine programs protective CD8+ T cell responses against influenza in newborns.bioRxiv [Preprint]. 2024 Apr 13:2024.04.09.588427. doi: 10.1101/2024.04.09.588427. bioRxiv. 2024. PMID: 38645130 Free PMC article. Preprint.
-
Preterm Infants' Airway Microbiome: A Scoping Review of the Current Evidence.Nutrients. 2024 Feb 6;16(4):465. doi: 10.3390/nu16040465. Nutrients. 2024. PMID: 38398790 Free PMC article. Review.
-
Molecular EPISTOP, a comprehensive multi-omic analysis of blood from Tuberous Sclerosis Complex infants age birth to two years.Nat Commun. 2023 Nov 23;14(1):7664. doi: 10.1038/s41467-023-42855-6. Nat Commun. 2023. PMID: 37996417 Free PMC article.
-
Birth Mode Does Not Determine the Presence of Shared Bacterial Strains between the Maternal Vaginal Microbiome and the Infant Stool Microbiome.Microbiol Spectr. 2023 Aug 17;11(4):e0061423. doi: 10.1128/spectrum.00614-23. Epub 2023 Jun 20. Microbiol Spectr. 2023. PMID: 37338388 Free PMC article.
-
Leveraging Microbial Symbiosis to Modulate Bronchopulmonary Dysplasia.Am J Respir Cell Mol Biol. 2023 Mar;68(3):235-236. doi: 10.1165/rcmb.2022-0415ED. Am J Respir Cell Mol Biol. 2023. PMID: 36346615 Free PMC article. No abstract available.
References
-
- Bischl B.L., Lang M., Kotthoff L., Schiffner J., Richter J., Studerus E., Casalicchio G., Jones Z. mlr: machine learning in R. J. Mach. Learn. Res. 2000;1:1–48.
-
- Bisgaard H., Li N., Bonnelykke K., Chawes B.L., Skov T., Paludan-Muller G., Stokholm J., Smith B., Krogfelt K.A. Reduced diversity of the intestinal microbiota during infancy is associated with increased risk of allergic disease at school age. J. Allergy Clin. Immunol. 2011;128:646–652.e1-5. - PubMed
Grants and funding
LinkOut - more resources
Full Text Sources