Tool evaluation for the detection of variably sized indels from next generation whole genome and targeted sequencing data
- PMID: 35176018
- PMCID: PMC8916674
- DOI: 10.1371/journal.pcbi.1009269
Tool evaluation for the detection of variably sized indels from next generation whole genome and targeted sequencing data
Abstract
Insertions and deletions (indels) in human genomes are associated with a wide range of phenotypes, including various clinical disorders. High-throughput, next generation sequencing (NGS) technologies enable the detection of short genetic variants, such as single nucleotide variants (SNVs) and indels. However, the variant calling accuracy for indels remains considerably lower than for SNVs. Here we present a comparative study of the performance of variant calling tools for indel calling, evaluated with a wide repertoire of NGS datasets. While there is no single optimal tool to suit all circumstances, our results demonstrate that the choice of variant calling tool greatly impacts the precision and recall of indel calling. Furthermore, to reliably detect indels, it is essential to choose NGS technologies that offer a long read length and high coverage coupled with specific variant calling tools.
Conflict of interest statement
The authors have declared that no competing interests exist.
Figures
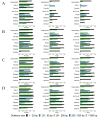
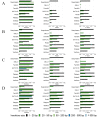
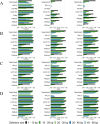
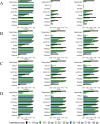
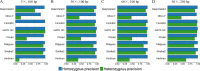
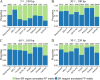
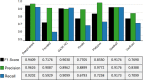
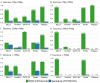
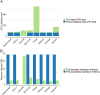
Similar articles
-
Optimized detection of insertions/deletions (INDELs) in whole-exome sequencing data.PLoS One. 2017 Aug 9;12(8):e0182272. doi: 10.1371/journal.pone.0182272. eCollection 2017. PLoS One. 2017. PMID: 28792971 Free PMC article.
-
SNVSniffer: an integrated caller for germline and somatic single-nucleotide and indel mutations.BMC Syst Biol. 2016 Aug 1;10 Suppl 2(Suppl 2):47. doi: 10.1186/s12918-016-0300-5. BMC Syst Biol. 2016. PMID: 27489955 Free PMC article.
-
Comparison of INDEL Calling Tools with Simulation Data and Real Short-Read Data.IEEE/ACM Trans Comput Biol Bioinform. 2019 Sep-Oct;16(5):1635-1644. doi: 10.1109/TCBB.2018.2854793. Epub 2018 Jul 10. IEEE/ACM Trans Comput Biol Bioinform. 2019. PMID: 30004886
-
Best practices for variant calling in clinical sequencing.Genome Med. 2020 Oct 26;12(1):91. doi: 10.1186/s13073-020-00791-w. Genome Med. 2020. PMID: 33106175 Free PMC article. Review.
-
Sequencing technologies and tools for short tandem repeat variation detection.Brief Bioinform. 2015 Mar;16(2):193-204. doi: 10.1093/bib/bbu001. Epub 2014 Feb 6. Brief Bioinform. 2015. PMID: 24504770 Review.
Cited by
-
Integrated approach to generate artificial samples with low tumor fraction for somatic variant calling benchmarking.BMC Bioinformatics. 2024 May 8;25(1):180. doi: 10.1186/s12859-024-05793-8. BMC Bioinformatics. 2024. PMID: 38720249 Free PMC article.
-
Comparative evaluation of SNVs, indels, and structural variations detected with short- and long-read sequencing data.Hum Genome Var. 2024 Apr 17;11(1):18. doi: 10.1038/s41439-024-00276-x. Hum Genome Var. 2024. PMID: 38632226 Free PMC article.
-
Histologic and Genomic Analysis of Conjunctival SCC in African and American Cohorts Reveal UV Light and HPV Signatures and High Tumor Mutation Burden.Invest Ophthalmol Vis Sci. 2024 Apr 1;65(4):24. doi: 10.1167/iovs.65.4.24. Invest Ophthalmol Vis Sci. 2024. PMID: 38597722 Free PMC article.
-
Hereditary Renal Cancer Syndromes.Med Sci (Basel). 2024 Feb 18;12(1):12. doi: 10.3390/medsci12010012. Med Sci (Basel). 2024. PMID: 38390862 Free PMC article. Review.
-
Relevance of mutation-derived neoantigens and non-classical antigens for anticancer therapies.Hum Vaccin Immunother. 2024 Dec 31;20(1):2303799. doi: 10.1080/21645515.2024.2303799. Epub 2024 Feb 12. Hum Vaccin Immunother. 2024. PMID: 38346926 Free PMC article. Review.
References
Publication types
MeSH terms
Grants and funding
LinkOut - more resources
Full Text Sources