A hierarchical study for urban statistical indicators on the prevalence of COVID-19 in Chinese city clusters based on multiple linear regression (MLR) and polynomial best subset regression (PBSR) analysis
- PMID: 35121784
- PMCID: PMC8817036
- DOI: 10.1038/s41598-022-05859-8
A hierarchical study for urban statistical indicators on the prevalence of COVID-19 in Chinese city clusters based on multiple linear regression (MLR) and polynomial best subset regression (PBSR) analysis
Abstract
With evidence-based measures, COVID-19 can be effectively controlled by advanced data analysis and prediction. However, while valuable insights are available, there is a shortage of robust and rigorous research on what factors shape COVID-19 transmissions at the city cluster level. Therefore, to bridge the research gap, we adopted a data-driven hierarchical modeling approach to identify the most influential factors in shaping COVID-19 transmissions across different Chinese cities and clusters. The data used in this study are from Chinese officials, and hierarchical modeling conclusions drawn from the analysis are systematic, multifaceted, and comprehensive. To further improve research rigor, the study utilizes SPSS, Python and RStudio to conduct multiple linear regression and polynomial best subset regression (PBSR) analysis for the hierarchical modeling. The regression model utilizes the magnitude of various relative factors in nine Chinese city clusters, including 45 cities at a different level of clusters, to examine these aspects from the city cluster scale, exploring the correlation between various factors of the cities. These initial 12 factors are comprised of 'Urban population ratio', 'Retail sales of consumer goods', 'Number of tourists', 'Tourism Income', 'Ratio of the elderly population (> 60 year old) in this city', 'population density', 'Mobility scale (move in/inbound) during the spring festival', 'Ratio of Population and Health facilities', 'Jobless rate (%)', 'The straight-line distance from original epicenter Wuhan to this city', 'urban per capita GDP', and 'the prevalence of the COVID-19'. The study's results provide rigorously-tested and evidence-based insights on most instrumental factors that shape COVID-19 transmissions across cities and regions in China. Overall, the study findings found that per capita GDP and population mobility rates were the most affected factors in the prevalence of COVID-19 in a city, which could inform health experts and government officials to design and develop evidence-based and effective public health policies that could curb the spread of the COVID-19 pandemic.
© 2022. The Author(s).
Conflict of interest statement
The authors declare no competing interests.
Figures
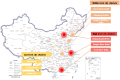
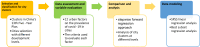


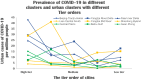
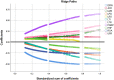
Similar articles
-
Associations between changes in population mobility in response to the COVID-19 pandemic and socioeconomic factors at the city level in China and country level worldwide: a retrospective, observational study.Lancet Digit Health. 2021 Jun;3(6):e349-e359. doi: 10.1016/S2589-7500(21)00059-5. Lancet Digit Health. 2021. PMID: 34045001 Free PMC article.
-
The impact of lockdown in Wuhan on residents confidence in controlling COVID-19 outbreak at the destination cities.Front Public Health. 2022 Aug 15;10:902455. doi: 10.3389/fpubh.2022.902455. eCollection 2022. Front Public Health. 2022. PMID: 36045730 Free PMC article.
-
Understanding the spatial diffusion dynamics of the COVID-19 pandemic in the city system in China.Soc Sci Med. 2022 Jun;302:114988. doi: 10.1016/j.socscimed.2022.114988. Epub 2022 Apr 28. Soc Sci Med. 2022. PMID: 35512611 Free PMC article.
-
Effects of COVID-19 on Urban Population Flow in China.Int J Environ Res Public Health. 2021 Feb 8;18(4):1617. doi: 10.3390/ijerph18041617. Int J Environ Res Public Health. 2021. PMID: 33567714 Free PMC article.
-
City-Scale Agent-Based Simulators for the Study of Non-pharmaceutical Interventions in the Context of the COVID-19 Epidemic: IISc-TIFR COVID-19 City-Scale Simulation Team.J Indian Inst Sci. 2020;100(4):809-847. doi: 10.1007/s41745-020-00211-3. Epub 2020 Nov 12. J Indian Inst Sci. 2020. PMID: 33199946 Free PMC article. Review.
Cited by
-
Discourse on COVID-19 Mass Testing vs. Rapid Testing Processing.Front Public Health. 2022 May 10;10:883490. doi: 10.3389/fpubh.2022.883490. eCollection 2022. Front Public Health. 2022. PMID: 35619817 Free PMC article. No abstract available.
-
Space and social distancing in managing and preventing COVID-19 community spread: An overview.Heliyon. 2023 Mar;9(3):e13879. doi: 10.1016/j.heliyon.2023.e13879. Epub 2023 Feb 20. Heliyon. 2023. PMID: 36845035 Free PMC article.
References
-
- Zhu N, Zhang D, Wang W, Li X, Yang B, Song J, Zhao X, Huang B, Shi W, Lu R, Niu P, Zhan F, Ma X, Wang D, Xu W, Wu G, Gao GF, Tan W, China Novel Coronavirus Investigating and Research Team A novel coronavirus from patients with pneumonia in China, 2019. N. Engl. J. Med. 2020;382(8):727–733. doi: 10.1056/NEJMoa2001017. - DOI - PMC - PubMed
-
- Jebril, N. World Health Organization declared a pandemic public health menace: a systematic review of the coronavirus disease 2019 “COVID-19”. Available at SSRN 3566298, from https://www.psychosocial.com/article/PR290311/25748/ (2020).
Publication types
MeSH terms
Grants and funding
LinkOut - more resources
Full Text Sources
Medical
Miscellaneous