Dysgu: efficient structural variant calling using short or long reads
- PMID: 35100420
- PMCID: PMC9122538
- DOI: 10.1093/nar/gkac039
Dysgu: efficient structural variant calling using short or long reads
Abstract
Structural variation (SV) plays a fundamental role in genome evolution and can underlie inherited or acquired diseases such as cancer. Long-read sequencing technologies have led to improvements in the characterization of structural variants (SVs), although paired-end sequencing offers better scalability. Here, we present dysgu, which calls SVs or indels using paired-end or long reads. Dysgu detects signals from alignment gaps, discordant and supplementary mappings, and generates consensus contigs, before classifying events using machine learning. Additional SVs are identified by remapping of anomalous sequences. Dysgu outperforms existing state-of-the-art tools using paired-end or long-reads, offering high sensitivity and precision whilst being among the fastest tools to run. We find that combining low coverage paired-end and long-reads is competitive in terms of performance with long-reads at higher coverage values.
© The Author(s) 2022. Published by Oxford University Press on behalf of Nucleic Acids Research.
Figures
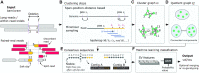
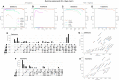
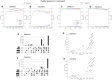
Similar articles
-
SvABA: genome-wide detection of structural variants and indels by local assembly.Genome Res. 2018 Apr;28(4):581-591. doi: 10.1101/gr.221028.117. Epub 2018 Mar 13. Genome Res. 2018. PMID: 29535149 Free PMC article.
-
Precise characterization of somatic complex structural variations from tumor/control paired long-read sequencing data with nanomonsv.Nucleic Acids Res. 2023 Aug 11;51(14):e74. doi: 10.1093/nar/gkad526. Nucleic Acids Res. 2023. PMID: 37336583 Free PMC article.
-
A Comparison of Structural Variant Calling from Short-Read and Nanopore-Based Whole-Genome Sequencing Using Optical Genome Mapping as a Benchmark.Genes (Basel). 2024 Jul 16;15(7):925. doi: 10.3390/genes15070925. Genes (Basel). 2024. PMID: 39062704 Free PMC article.
-
SVJedi: genotyping structural variations with long reads.Bioinformatics. 2020 Nov 1;36(17):4568-4575. doi: 10.1093/bioinformatics/btaa527. Bioinformatics. 2020. PMID: 32437523
-
Automated filtering of genome-wide large deletions through an ensemble deep learning framework.Methods. 2022 Oct;206:77-86. doi: 10.1016/j.ymeth.2022.08.001. Epub 2022 Aug 28. Methods. 2022. PMID: 36038049
Cited by
-
Optimizing Design of Genomics Studies for Clonal Evolution Analysis.bioRxiv [Preprint]. 2024 Mar 15:2024.03.14.585055. doi: 10.1101/2024.03.14.585055. bioRxiv. 2024. Update in: Bioinform Adv. 2024 Dec 02;4(1):vbae193. doi: 10.1093/bioadv/vbae193 PMID: 38559253 Free PMC article. Updated. Preprint.
-
Comprehensive analysis of structural variants in chickens using PacBio sequencing.Front Genet. 2022 Oct 20;13:971588. doi: 10.3389/fgene.2022.971588. eCollection 2022. Front Genet. 2022. PMID: 36338955 Free PMC article.
-
Correspondence on NanoVar's performance outlined by Jiang T. et al. in "Long-read sequencing settings for efficient structural variation detection based on comprehensive evaluation".BMC Bioinformatics. 2023 Sep 20;24(1):350. doi: 10.1186/s12859-023-05484-w. BMC Bioinformatics. 2023. PMID: 37730547 Free PMC article.
-
Genome-wide characterization of structure variations in the Xiang pig for genetic resistance to African swine fever.Virulence. 2024 Dec;15(1):2382762. doi: 10.1080/21505594.2024.2382762. Epub 2024 Aug 2. Virulence. 2024. PMID: 39092797 Free PMC article.
-
When less is more: sketching with minimizers in genomics.Genome Biol. 2024 Oct 14;25(1):270. doi: 10.1186/s13059-024-03414-4. Genome Biol. 2024. PMID: 39402664 Free PMC article. Review.
References
MeSH terms
Grants and funding
LinkOut - more resources
Full Text Sources