A Machine Learning-Based Aging Measure Among Middle-Aged and Older Chinese Adults: The China Health and Retirement Longitudinal Study
- PMID: 34926482
- PMCID: PMC8671693
- DOI: 10.3389/fmed.2021.698851
A Machine Learning-Based Aging Measure Among Middle-Aged and Older Chinese Adults: The China Health and Retirement Longitudinal Study
Abstract
Objective: Biological age (BA) has been accepted as a more accurate proxy of aging than chronological age (CA). This study aimed to use machine learning (ML) algorithms to estimate BA in the Chinese population. Materials and methods: We used data from 9,771 middle-aged and older Chinese adults (≥45 years) in the 2011/2012 wave of the China Health and Retirement Longitudinal Study and followed until 2018. We used several ML algorithms (e.g., Gradient Boosting Regressor, Random Forest, CatBoost Regressor, and Support Vector Machine) to develop new measures of biological aging (ML-BAs) based on physiological biomarkers. R-squared value and mean absolute error (MAE) were used to determine the optimal performance of these ML-BAs. We used logistic regression models to examine the associations of the best ML-BA and a conventional aging measure-Klemera and Doubal method-BA (KDM-BA) we previously developed-with physical disability and mortality, respectively. Results: The Gradient Boosting Regression model performed the best, resulting in an ML-BA with an R-squared value of 0.270 and an MAE of 6.519. This ML-BA was significantly associated with disability in basic activities of daily living, instrumental activities of daily living, lower extremity mobility, and upper extremity mobility, and mortality, with odds ratios ranging from 1 to 7% (per 1-year increment in ML-BA, all P < 0.001), independent of CA. These associations were generally comparable to that of KDM-BA. Conclusion: This study provides a valid ML-based measure of biological aging for middle-aged and older Chinese adults. These findings support the application of ML in geroscience research and may help facilitate preventive and geroprotector intervention studies.
Keywords: aging measure; biological age; disability; machine learning; mortality.
Copyright © 2021 Cao, Yang, Jin, He, Li, Zheng, Liu and Wu.
Conflict of interest statement
XJ is employed by MindRank AI ltd. The remaining authors declare that the research was conducted in the absence of any commercial or financial relationships that could be construed as a potential conflict of interest.
Figures
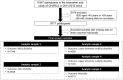
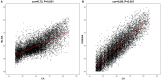
Similar articles
-
Estimating Biological Age in the Singapore Longitudinal Aging Study.J Gerontol A Biol Sci Med Sci. 2020 Sep 25;75(10):1913-1920. doi: 10.1093/gerona/glz146. J Gerontol A Biol Sci Med Sci. 2020. PMID: 31179487
-
Development and Validation of 2 Composite Aging Measures Using Routine Clinical Biomarkers in the Chinese Population: Analyses From 2 Prospective Cohort Studies.J Gerontol A Biol Sci Med Sci. 2021 Aug 13;76(9):1627-1632. doi: 10.1093/gerona/glaa238. J Gerontol A Biol Sci Med Sci. 2021. PMID: 32946548 Free PMC article.
-
Daytime napping, biological aging and cognitive function among middle-aged and older Chinese: insights from the China health and retirement longitudinal study.Front Public Health. 2023 Nov 17;11:1294948. doi: 10.3389/fpubh.2023.1294948. eCollection 2023. Front Public Health. 2023. PMID: 38045976 Free PMC article.
-
Association between Accelerated Biological Aging, Diet, and Gut Microbiome.Microorganisms. 2024 Aug 20;12(8):1719. doi: 10.3390/microorganisms12081719. Microorganisms. 2024. PMID: 39203561 Free PMC article. Review.
-
Progress in biological age research.Front Public Health. 2023 Apr 12;11:1074274. doi: 10.3389/fpubh.2023.1074274. eCollection 2023. Front Public Health. 2023. PMID: 37124811 Free PMC article. Review.
Cited by
-
Prediction of posttraumatic functional recovery in middle-aged and older patients through dynamic ensemble selection modeling.Front Public Health. 2023 Jun 20;11:1164820. doi: 10.3389/fpubh.2023.1164820. eCollection 2023. Front Public Health. 2023. PMID: 37408743 Free PMC article.
-
Physiological Dysregulation Proceeds and Predicts Health Outcomes Similarly in Chinese and Western Populations.J Gerontol A Biol Sci Med Sci. 2024 Jan 1;79(1):glad146. doi: 10.1093/gerona/glad146. J Gerontol A Biol Sci Med Sci. 2024. PMID: 37313838 Free PMC article.
-
A model for predicting physical function upon discharge of hospitalized older adults in Taiwan-a machine learning approach based on both electronic health records and comprehensive geriatric assessment.Front Med (Lausanne). 2023 Jul 21;10:1160013. doi: 10.3389/fmed.2023.1160013. eCollection 2023. Front Med (Lausanne). 2023. PMID: 37547611 Free PMC article.
-
A machine learning-based data mining in medical examination data: a biological features-based biological age prediction model.BMC Bioinformatics. 2022 Oct 3;23(1):411. doi: 10.1186/s12859-022-04966-7. BMC Bioinformatics. 2022. PMID: 36192681 Free PMC article.
-
Biomarkers of aging.Sci China Life Sci. 2023 May;66(5):893-1066. doi: 10.1007/s11427-023-2305-0. Epub 2023 Apr 11. Sci China Life Sci. 2023. PMID: 37076725 Free PMC article. Review.
References
LinkOut - more resources
Full Text Sources