DNA Methylation Modification Map to Predict Tumor Molecular Subtypes and Efficacy of Immunotherapy in Bladder Cancer
- PMID: 34926451
- PMCID: PMC8678484
- DOI: 10.3389/fcell.2021.760369
DNA Methylation Modification Map to Predict Tumor Molecular Subtypes and Efficacy of Immunotherapy in Bladder Cancer
Abstract
Background: Considering the heterogeneity and complexity of epigenetic regulation in bladder cancer, the underlying mechanisms of global DNA methylation modification in the immune microenvironment must be investigated to predict the prognosis outcomes and clinical response to immunotherapy. Methods: We systematically assessed the DNA methylation modes of 985 integrated bladder cancer samples with the unsupervised clustering algorithm. Subsequently, these DNA methylation modes were analyzed for their correlations with features of the immune microenvironment. The principal analysis algorithm was performed to calculate the DMRscores of each samples for qualification analysis. Findings: Three DNA methylation modes were revealed among 985 bladder cancer samples, and these modes are related to diverse clinical outcomes and several immune microenvironment phenotypes, e.g., immune-desert, immune-inflamed, and immune-excluded ones. Then patients were classified into high- and low-DMRscore subgroups according to the DMRscore, which was calculated based on the expression of DNA methylation related genes (DMRGs). Patients with the low-DMRscore subgroup presented a prominent survival advantage that was significantly correlated to the immune-inflamed phenotype. Further analysis revealed that patients with low DMRscores exhibited less TP53 wild mutation, lower cancer stage and molecular subtypes were mainly papillary subtypes. In addition, an independent immunotherapy cohort confirmed that DMRscore could serve as a signature to predict prognosis outcomes and immune responses. Conclusion: Global DNA methylation modes can be used to predict the immunophenotypes, aggressiveness, and immune responses of bladder cancer. DNA methylation status assessments will strengthen our insights into the features of the immune microenvironment and promote the development of more effective treatment strategies.
Keywords: DNA methylation regulators; bladder cancer; immunotherapy; prognostic model; tumor microenvironment.
Copyright © 2021 Ye, Liang, Hu, Hu, Liu, Cheng, Ou, Xu and Jiang.
Conflict of interest statement
The authors declare that the research was conducted in the absence of any commercial or financial relationships that could be construed as a potential conflict of interest.
Figures
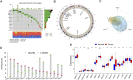
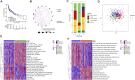
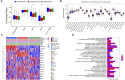
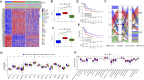
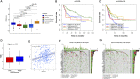
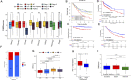
Similar articles
-
DNA methylation regulator-mediated modification pattern defines tumor microenvironment immune infiltration landscape in colon cancer.Front Genet. 2022 Oct 6;13:1008644. doi: 10.3389/fgene.2022.1008644. eCollection 2022. Front Genet. 2022. PMID: 36276973 Free PMC article.
-
m6A regulator-mediated methylation modification patterns and tumor microenvironment infiltration characterization in gastric cancer.Mol Cancer. 2020 Mar 12;19(1):53. doi: 10.1186/s12943-020-01170-0. Mol Cancer. 2020. PMID: 32164750 Free PMC article.
-
m6A regulator-based methylation modification patterns characterized by distinct tumor microenvironment immune profiles in colon cancer.Theranostics. 2021 Jan 1;11(5):2201-2217. doi: 10.7150/thno.52717. eCollection 2021. Theranostics. 2021. PMID: 33500720 Free PMC article.
-
The interaction between DNA methylation and tumor immune microenvironment: from the laboratory to clinical applications.Clin Epigenetics. 2024 Feb 8;16(1):24. doi: 10.1186/s13148-024-01633-x. Clin Epigenetics. 2024. PMID: 38331927 Free PMC article. Review.
-
Tumor battlefield within inflamed, excluded or desert immune phenotypes: the mechanisms and strategies.Exp Hematol Oncol. 2024 Aug 6;13(1):80. doi: 10.1186/s40164-024-00543-1. Exp Hematol Oncol. 2024. PMID: 39107856 Free PMC article. Review.
Cited by
-
The Simultaneous Use of Bladder Epicheck® and Urinary Cytology Can Improve the Sensitivity and Specificity of Diagnostic Follow-Up of Urothelial Lesions: Up-to-Date Data from a Multi-Institutional Cohort.Diseases. 2024 Sep 18;12(9):219. doi: 10.3390/diseases12090219. Diseases. 2024. PMID: 39329888 Free PMC article.
-
Epigenetic and Immunological Features of Bladder Cancer.Int J Mol Sci. 2023 Jun 7;24(12):9854. doi: 10.3390/ijms24129854. Int J Mol Sci. 2023. PMID: 37373000 Free PMC article. Review.
-
A novel cervix carcinoma biomarker: Pathological-epigenomics, integrated analysis of MethylMix algorithm and pathology for predicting response to cancer immunotherapy.Front Oncol. 2022 Nov 2;12:1053800. doi: 10.3389/fonc.2022.1053800. eCollection 2022. Front Oncol. 2022. PMID: 36408176 Free PMC article.
-
Preoperative Systemic Inflammatory Markers as a Significant Prognostic Factor After TURBT in Patients with Non-Muscle-Invasive Bladder Cancer.J Inflamm Res. 2023 Jan 21;16:283-296. doi: 10.2147/JIR.S393511. eCollection 2023. J Inflamm Res. 2023. PMID: 36713048 Free PMC article.
-
Biomarkers and experimental models for cancer immunology investigation.MedComm (2020). 2023 Dec 2;4(6):e437. doi: 10.1002/mco2.437. eCollection 2023 Dec. MedComm (2020). 2023. PMID: 38045830 Free PMC article. Review.
References
LinkOut - more resources
Full Text Sources
Research Materials
Miscellaneous