Enhancing PCORnet Clinical Research Network data completeness by integrating multistate insurance claims with electronic health records in a cloud environment aligned with CMS security and privacy requirements
- PMID: 34897506
- PMCID: PMC8922172
- DOI: 10.1093/jamia/ocab269
Enhancing PCORnet Clinical Research Network data completeness by integrating multistate insurance claims with electronic health records in a cloud environment aligned with CMS security and privacy requirements
Abstract
Objective: The Greater Plains Collaborative (GPC) and other PCORnet Clinical Data Research Networks capture healthcare utilization within their health systems. Here, we describe a reusable environment (GPC Reusable Observable Unified Study Environment [GROUSE]) that integrates hospital and electronic health records (EHRs) data with state-wide Medicare and Medicaid claims and assess how claims and clinical data complement each other to identify obesity and related comorbidities in a patient sample.
Materials and methods: EHR, billing, and tumor registry data from 7 healthcare systems were integrated with Center for Medicare (2011-2016) and Medicaid (2011-2012) services insurance claims to create deidentified databases in Informatics for Integrating Biology & the Bedside and PCORnet Common Data Model formats. We describe technical details of how this federally compliant, cloud-based data environment was built. As a use case, trends in obesity rates for different age groups are reported, along with the relative contribution of claims and EHR data-to-data completeness and detecting common comorbidities.
Results: GROUSE contained 73 billion observations from 24 million unique patients (12.9 million Medicare; 13.9 million Medicaid; 6.6 million GPC patients) with 1 674 134 patients crosswalked and 983 450 patients with body mass index (BMI) linked to claims. Diagnosis codes from EHR and claims sources underreport obesity by 2.56 times compared with body mass index measures. However, common comorbidities such as diabetes and sleep apnea diagnoses were more often available from claims diagnoses codes (1.6 and 1.4 times, respectively).
Conclusion: GROUSE provides a unified EHR-claims environment to address health system and federal privacy concerns, which enables investigators to generalize analyses across health systems integrated with multistate insurance claims.
Keywords: Amazon Web Services private cloud; Centers for Medicare and Medicaid Services; PCORnet; Patient-Centered Outcomes Research Institute; cloud computing; electronic health records; obesity.
© The Author(s) 2021. Published by Oxford University Press on behalf of the American Medical Informatics Association. All rights reserved. For permissions, please email: journals.permissions@oup.com.
Figures
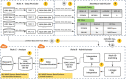
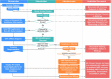
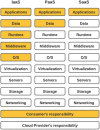
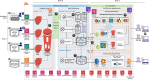
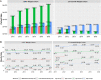
Similar articles
-
Using pooled electronic health records data to conduct pharmacoepidemiology safety studies: Challenges and lessons learned.Pharmacoepidemiol Drug Saf. 2023 Sep;32(9):969-977. doi: 10.1002/pds.5627. Epub 2023 Apr 15. Pharmacoepidemiol Drug Saf. 2023. PMID: 37005701 Free PMC article.
-
Development and Validation of a Claims-Based Model to Predict Categories of Obesity.Am J Epidemiol. 2024 Jan 8;193(1):203-213. doi: 10.1093/aje/kwad178. Am J Epidemiol. 2024. PMID: 37650647 Free PMC article.
-
Comparison of EHR Data-Completeness in Patients with Different Types of Medical Insurance Coverage in the United States.Clin Pharmacol Ther. 2023 Nov;114(5):1116-1125. doi: 10.1002/cpt.3027. Epub 2023 Sep 1. Clin Pharmacol Ther. 2023. PMID: 37597260 Free PMC article.
-
Developing Methods to Link Patient Records across Data Sets That Preserve Patient Privacy [Internet].Washington (DC): Patient-Centered Outcomes Research Institute (PCORI); 2020 Jun. Washington (DC): Patient-Centered Outcomes Research Institute (PCORI); 2020 Jun. PMID: 37535796 Free Books & Documents. Review.
-
Database and Registry Research in Orthopaedic Surgery: Part I: Claims-Based Data.J Bone Joint Surg Am. 2015 Aug 5;97(15):1278-87. doi: 10.2106/JBJS.N.01260. J Bone Joint Surg Am. 2015. PMID: 26246263 Review.
Cited by
-
Leveraging multi-site electronic health data for characterization of subtypes: a pilot study of dementia in the N3C Clinical Tenant.JAMIA Open. 2024 Aug 6;7(3):ooae076. doi: 10.1093/jamiaopen/ooae076. eCollection 2024 Oct. JAMIA Open. 2024. PMID: 39132679 Free PMC article.
-
Fitness-for-use of Retrospective Multicenter Electronic Health Records to Conduct Outcome Analysis for Pediatric Ulcerative Colitis.Medicine (Baltimore). 2024 Mar 15;103(11):e37395. doi: 10.1097/MD.0000000000037395. Medicine (Baltimore). 2024. PMID: 38489703 Free PMC article.
-
Association of prior treatment with nitrogen-containing bisphosphonates on outcomes of COVID-19 positive patients.Osteoporos Int. 2024 Jan;35(1):181-187. doi: 10.1007/s00198-023-06912-6. Epub 2023 Sep 13. Osteoporos Int. 2024. PMID: 37700010
-
Common data model for sickle cell disease surveillance: considerations and implications.JAMIA Open. 2023 May 27;6(2):ooad036. doi: 10.1093/jamiaopen/ooad036. eCollection 2023 Jul. JAMIA Open. 2023. PMID: 37252051 Free PMC article.
-
Use Cases Requiring Privacy-Preserving Record Linkage in Paediatric Oncology.Cancers (Basel). 2024 Jul 29;16(15):2696. doi: 10.3390/cancers16152696. Cancers (Basel). 2024. PMID: 39123424 Free PMC article.
References
-
- The Greater Plains Collaborative (GPC). Secondary The Greater Plains Collaborative. 2015. http://www.gpcnetwork.org/ Accessed July 1, 2021.
-
- NIH. National Institutes of Health, National Center for Advancing Translational Sciences, Clinical and Translational Science Award (CTSA) Program. Secondary National Institutes of Health, National Center for Advancing Translational Sciences, Clinical and Translational Science Award (CTSA) Program. 2013. https://ncats.nih.gov/ctsa.
-
- PCORI. Patient-Centered Outcomes Research Institute Cooperative Agreement Funding Announcement: Improving Infrastructure for Conducting Patient-Centered Outcomes Research. The National Patient-Centered Clinical Research Network: Clinical Data Research Networks (CDRN)—Phase One. Secondary Patient-Centered Outcomes Research Institute Cooperative Agreement Funding Announcement: Improving Infrastructure for Conducting Patient-Centered Outcomes Research. The National Patient-Centered Clinical Research Network: Clinical Data Research Networks (CDRN)—Phase One. 2013. https://www.pcori.org/sites/default/files/PCORI-PFA-CDRN-071713.pdf.
Publication types
MeSH terms
Grants and funding
LinkOut - more resources
Full Text Sources
Research Materials