Identification and Validation of Immune-Related Gene for Predicting Prognosis and Therapeutic Response in Ovarian Cancer
- PMID: 34880862
- PMCID: PMC8645858
- DOI: 10.3389/fimmu.2021.763791
Identification and Validation of Immune-Related Gene for Predicting Prognosis and Therapeutic Response in Ovarian Cancer
Abstract
Ovarian cancer (OC) is a devastating malignancy with a poor prognosis. The complex tumor immune microenvironment results in only a small number of patients benefiting from immunotherapy. To explore the different factors that lead to immune invasion and determine prognosis and response to immune checkpoint inhibitors (ICIs), we established a prognostic risk scoring model (PRSM) with differential expression of immune-related genes (IRGs) to identify key prognostic IRGs. Patients were divided into high-risk and low-risk groups according to their immune and stromal scores. We used a bioinformatics method to identify four key IRGs that had differences in expression between the two groups and affected prognosis. We evaluated the sensitivity of treatment from three aspects, namely chemotherapy, targeted inhibitors (TIs), and immunotherapy, to evaluate the value of prediction models and key prognostic IRGs in the clinical treatment of OC. Univariate and multivariate Cox regression analyses revealed that these four key IRGs were independent prognostic factors of overall survival in OC patients. In the high-risk group comprising four genes, macrophage M0 cells, macrophage M2 cells, and regulatory T cells, observed to be associated with poor overall survival in our study, were higher. The high-risk group had a high immunophenoscore, indicating a better response to ICIs. Taken together, we constructed a PRSM and identified four key prognostic IRGs for predicting survival and response to ICIs. Finally, the expression of these key genes in OC was evaluated using RT-qPCR. Thus, these genes provide a novel predictive biomarker for immunotherapy and immunomodulation.
Keywords: immune checkpoint inhibitors (ICI); immune-related genes (IRGs); ovarian cancer; prognosis; tumor immune microenvironment.
Copyright © 2021 Zhang, Guo, Zhang, Wang, Lou, Cui and Yang.
Conflict of interest statement
The authors declare that the research was conducted in the absence of any commercial or financial relationships that could be construed as a potential conflict of interest.
Figures
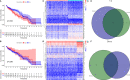
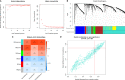
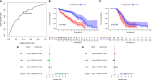
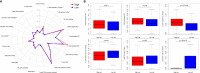
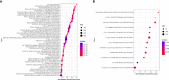
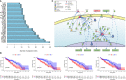
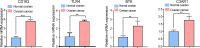
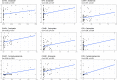
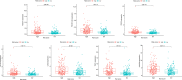
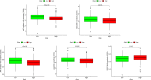
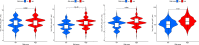
Similar articles
-
Single-sample gene set enrichment analysis reveals the clinical implications of immune-related genes in ovarian cancer.Front Mol Biosci. 2024 Aug 5;11:1426274. doi: 10.3389/fmolb.2024.1426274. eCollection 2024. Front Mol Biosci. 2024. PMID: 39161779 Free PMC article.
-
Prognostic Immune-Related Analysis Based on Differentially Expressed Genes in Left- and Right-Sided Colon Adenocarcinoma.Front Oncol. 2021 Mar 8;11:640196. doi: 10.3389/fonc.2021.640196. eCollection 2021. Front Oncol. 2021. PMID: 33763372 Free PMC article.
-
Immune-Related Genes' Prognostic, Therapeutic and Diagnostic Value in Ovarian Cancer Immune-Related Gene Biomarker in Ovarian Cancer.Cancer Control. 2023 Jan-Dec;30:10732748231168756. doi: 10.1177/10732748231168756. Cancer Control. 2023. PMID: 37078136 Free PMC article.
-
Current Understanding on Why Ovarian Cancer Is Resistant to Immune Checkpoint Inhibitors.Int J Mol Sci. 2023 Jun 29;24(13):10859. doi: 10.3390/ijms241310859. Int J Mol Sci. 2023. PMID: 37446039 Free PMC article. Review.
-
Efficacy of immune checkpoint inhibitor monotherapy or combined with other small molecule-targeted agents in ovarian cancer.Expert Rev Mol Med. 2023 Jan 24;25:e6. doi: 10.1017/erm.2023.3. Expert Rev Mol Med. 2023. PMID: 36691778 Review.
References
Publication types
MeSH terms
Substances
LinkOut - more resources
Full Text Sources
Medical