Artificial intelligence in the prediction of protein-ligand interactions: recent advances and future directions
- PMID: 34849575
- PMCID: PMC8690157
- DOI: 10.1093/bib/bbab476
Artificial intelligence in the prediction of protein-ligand interactions: recent advances and future directions
Abstract
New drug production, from target identification to marketing approval, takes over 12 years and can cost around $2.6 billion. Furthermore, the COVID-19 pandemic has unveiled the urgent need for more powerful computational methods for drug discovery. Here, we review the computational approaches to predicting protein-ligand interactions in the context of drug discovery, focusing on methods using artificial intelligence (AI). We begin with a brief introduction to proteins (targets), ligands (e.g. drugs) and their interactions for nonexperts. Next, we review databases that are commonly used in the domain of protein-ligand interactions. Finally, we survey and analyze the machine learning (ML) approaches implemented to predict protein-ligand binding sites, ligand-binding affinity and binding pose (conformation) including both classical ML algorithms and recent deep learning methods. After exploring the correlation between these three aspects of protein-ligand interaction, it has been proposed that they should be studied in unison. We anticipate that our review will aid exploration and development of more accurate ML-based prediction strategies for studying protein-ligand interactions.
Keywords: binding affinity; binding pose; binding site; deep learning; drug discovery; machine learning; protein–ligand interaction.
© The Author(s) 2021. Published by Oxford University Press.
Figures
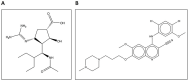
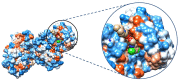
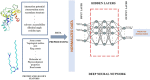
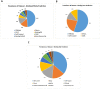
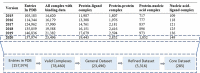
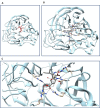
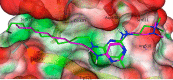
Similar articles
-
Computational anti-COVID-19 drug design: progress and challenges.Brief Bioinform. 2022 Jan 17;23(1):bbab484. doi: 10.1093/bib/bbab484. Brief Bioinform. 2022. PMID: 34850817 Free PMC article. Review.
-
Streamlining Computational Fragment-Based Drug Discovery through Evolutionary Optimization Informed by Ligand-Based Virtual Prescreening.J Chem Inf Model. 2024 May 13;64(9):3826-3840. doi: 10.1021/acs.jcim.4c00234. Epub 2024 May 2. J Chem Inf Model. 2024. PMID: 38696451 Free PMC article.
-
Knowing and combating the enemy: a brief review on SARS-CoV-2 and computational approaches applied to the discovery of drug candidates.Biosci Rep. 2021 Mar 26;41(3):BSR20202616. doi: 10.1042/BSR20202616. Biosci Rep. 2021. PMID: 33624754 Free PMC article. Review.
-
Advances in Magnetic Microbead Affinity Selection Screening: Discovery of Natural Ligands to the SARS-CoV-2 Spike Protein.J Am Soc Mass Spectrom. 2022 Jan 5;33(1):181-188. doi: 10.1021/jasms.1c00318. Epub 2021 Dec 23. J Am Soc Mass Spectrom. 2022. PMID: 34939787 Free PMC article.
-
Machine Learning for Sequence and Structure-Based Protein-Ligand Interaction Prediction.J Chem Inf Model. 2024 Mar 11;64(5):1456-1472. doi: 10.1021/acs.jcim.3c01841. Epub 2024 Feb 22. J Chem Inf Model. 2024. PMID: 38385768 Review.
Cited by
-
Revolutionizing Medicinal Chemistry: The Application of Artificial Intelligence (AI) in Early Drug Discovery.Pharmaceuticals (Basel). 2023 Sep 6;16(9):1259. doi: 10.3390/ph16091259. Pharmaceuticals (Basel). 2023. PMID: 37765069 Free PMC article. Review.
-
Benchmarking Cross-Docking Strategies for Structure-Informed Machine Learning in Kinase Drug Discovery.bioRxiv [Preprint]. 2023 Sep 14:2023.09.11.557138. doi: 10.1101/2023.09.11.557138. bioRxiv. 2023. Update in: J Chem Inf Model. 2024 Dec 9;64(23):8848-8858. doi: 10.1021/acs.jcim.4c00905. PMID: 37745489 Free PMC article. Updated. Preprint.
-
Drug-Online: an online platform for drug-target interaction, affinity, and binding sites identification using deep learning.BMC Bioinformatics. 2024 Apr 20;25(1):156. doi: 10.1186/s12859-024-05783-w. BMC Bioinformatics. 2024. PMID: 38641811 Free PMC article.
-
Cryo2StructData: A Large Labeled Cryo-EM Density Map Dataset for AI-based Modeling of Protein Structures.bioRxiv [Preprint]. 2024 Jan 2:2023.06.14.545024. doi: 10.1101/2023.06.14.545024. bioRxiv. 2024. Update in: Sci Data. 2024 May 6;11(1):458. doi: 10.1038/s41597-024-03299-9. PMID: 37398020 Free PMC article. Updated. Preprint.
-
Artificial Intelligence (AI) Applications in Drug Discovery and Drug Delivery: Revolutionizing Personalized Medicine.Pharmaceutics. 2024 Oct 14;16(10):1328. doi: 10.3390/pharmaceutics16101328. Pharmaceutics. 2024. PMID: 39458657 Free PMC article. Review.
References
Publication types
MeSH terms
Substances
Grants and funding
LinkOut - more resources
Full Text Sources
Medical