A Deep Learning and XGBoost-Based Method for Predicting Protein-Protein Interaction Sites
- PMID: 34764983
- PMCID: PMC8576272
- DOI: 10.3389/fgene.2021.752732
A Deep Learning and XGBoost-Based Method for Predicting Protein-Protein Interaction Sites
Abstract
Knowledge about protein-protein interactions is beneficial in understanding cellular mechanisms. Protein-protein interactions are usually determined according to their protein-protein interaction sites. Due to the limitations of current techniques, it is still a challenging task to detect protein-protein interaction sites. In this article, we presented a method based on deep learning and XGBoost (called DeepPPISP-XGB) for predicting protein-protein interaction sites. The deep learning model served as a feature extractor to remove redundant information from protein sequences. The Extreme Gradient Boosting algorithm was used to construct a classifier for predicting protein-protein interaction sites. The DeepPPISP-XGB achieved the following results: area under the receiver operating characteristic curve of 0.681, a recall of 0.624, and area under the precision-recall curve of 0.339, being competitive with the state-of-the-art methods. We also validated the positive role of global features in predicting protein-protein interaction sites.
Keywords: deep learning; extreme gradient boosting; machine learning; protein functions; protein-protein interaction.
Copyright © 2021 Wang, Zhang, Yu and Huang.
Conflict of interest statement
The authors declare that the research was conducted in the absence of any commercial or financial relationships that could be construed as a potential conflict of interest.
Figures
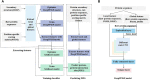
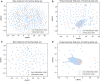
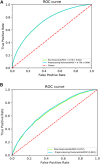
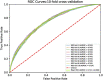
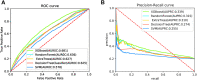
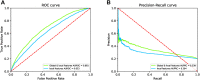
Similar articles
-
A machine learning-based model for predicting distant metastasis in patients with rectal cancer.Front Oncol. 2023 Aug 15;13:1235121. doi: 10.3389/fonc.2023.1235121. eCollection 2023. Front Oncol. 2023. PMID: 37655097 Free PMC article.
-
Prediction of protein-protein interaction sites through eXtreme gradient boosting with kernel principal component analysis.Comput Biol Med. 2021 Jul;134:104516. doi: 10.1016/j.compbiomed.2021.104516. Epub 2021 Jun 1. Comput Biol Med. 2021. PMID: 34119922
-
Ultrasound deep learning radiomics and clinical machine learning models to predict low nuclear grade, ER, PR, and HER2 receptor status in pure ductal carcinoma in situ.Gland Surg. 2024 Apr 29;13(4):512-527. doi: 10.21037/gs-23-417. Epub 2024 Apr 11. Gland Surg. 2024. PMID: 38720675 Free PMC article.
-
Predicting Measles Outbreaks in the United States: Evaluation of Machine Learning Approaches.JMIR Form Res. 2023 Apr 4;7:e42832. doi: 10.2196/42832. JMIR Form Res. 2023. PMID: 37014694 Free PMC article.
-
Interpretable machine learning model to predict surgical difficulty in laparoscopic resection for rectal cancer.Front Oncol. 2024 Feb 6;14:1337219. doi: 10.3389/fonc.2024.1337219. eCollection 2024. Front Oncol. 2024. PMID: 38380369 Free PMC article. Review.
Cited by
-
Fast end-to-end surface interpretation of SARS-CoV-2 variants by differentiable molecular surface interaction fingerprinting method.Comput Struct Biotechnol J. 2023 Sep 28;21:4816-4824. doi: 10.1016/j.csbj.2023.09.033. eCollection 2023. Comput Struct Biotechnol J. 2023. PMID: 37841329 Free PMC article.
-
Recent Advances in Deep Learning for Protein-Protein Interaction Analysis: A Comprehensive Review.Molecules. 2023 Jul 2;28(13):5169. doi: 10.3390/molecules28135169. Molecules. 2023. PMID: 37446831 Free PMC article. Review.
-
Identification of novel interacts partners of ADAR1 enzyme mediating the oncogenic process in aggressive breast cancer.Sci Rep. 2023 May 23;13(1):8341. doi: 10.1038/s41598-023-35517-6. Sci Rep. 2023. PMID: 37221310 Free PMC article.
References
-
- Bagchi A. (2015). Use of Machine Learning Features to Detect Protein-Protein Interaction Sites at the Molecular Level. Inf. Syst. Des. Intell. Appl., 49–54. Springer. 10.1007/978-81-322-2247-7_6 - DOI
LinkOut - more resources
Full Text Sources