Physics-informed deep learning characterizes morphodynamics of Asian soybean rust disease
- PMID: 34741028
- PMCID: PMC8571353
- DOI: 10.1038/s41467-021-26577-1
Physics-informed deep learning characterizes morphodynamics of Asian soybean rust disease
Abstract
Medicines and agricultural biocides are often discovered using large phenotypic screens across hundreds of compounds, where visible effects of whole organisms are compared to gauge efficacy and possible modes of action. However, such analysis is often limited to human-defined and static features. Here, we introduce a novel framework that can characterize shape changes (morphodynamics) for cell-drug interactions directly from images, and use it to interpret perturbed development of Phakopsora pachyrhizi, the Asian soybean rust crop pathogen. We describe population development over a 2D space of shapes (morphospace) using two models with condition-dependent parameters: a top-down Fokker-Planck model of diffusive development over Waddington-type landscapes, and a bottom-up model of tip growth. We discover a variety of landscapes, describing phenotype transitions during growth, and identify possible perturbations in the tip growth machinery that cause this variation. This demonstrates a widely-applicable integration of unsupervised learning and biophysical modeling.
© 2021. The Author(s).
Conflict of interest statement
The authors declare no competing interests.
Figures
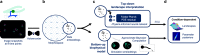
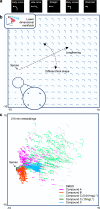
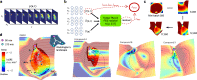
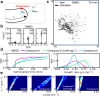
Similar articles
-
Transcriptome-based analyses of phosphite-mediated suppression of rust pathogens Puccinia emaculata and Phakopsora pachyrhizi and functional characterization of selected fungal target genes.Plant J. 2018 Mar;93(5):894-904. doi: 10.1111/tpj.13817. Epub 2018 Feb 6. Plant J. 2018. PMID: 29315949
-
From Select Agent to an Established Pathogen: The Response to Phakopsora pachyrhizi (Soybean Rust) in North America.Phytopathology. 2015 Jul;105(7):905-16. doi: 10.1094/PHYTO-02-15-0054-FI. Epub 2015 Jul 1. Phytopathology. 2015. PMID: 25775102 Review.
-
Correlations between traits in soybean (Glycine max L.) naturally infected with Asian rust (Phakopsora pachyrhizi).Genet Mol Res. 2015 Dec 22;14(4):17718-29. doi: 10.4238/2015.December.21.45. Genet Mol Res. 2015. PMID: 26782417
-
Near-isogenic soybean lines carrying Asian soybean rust resistance genes for practical pathogenicity validation.Sci Rep. 2020 Aug 6;10(1):13270. doi: 10.1038/s41598-020-70188-7. Sci Rep. 2020. PMID: 32764613 Free PMC article.
-
Phakopsora pachyrhizi, the causal agent of Asian soybean rust.Mol Plant Pathol. 2010 Mar;11(2):169-77. doi: 10.1111/j.1364-3703.2009.00589.x. Mol Plant Pathol. 2010. PMID: 20447267 Free PMC article. Review.
Cited by
-
DC2Net: An Asian Soybean Rust Detection Model Based on Hyperspectral Imaging and Deep Learning.Plant Phenomics. 2024 Apr 5;6:0163. doi: 10.34133/plantphenomics.0163. eCollection 2024. Plant Phenomics. 2024. PMID: 38586218 Free PMC article.
-
Plant science in the age of simulation intelligence.Front Plant Sci. 2024 Jan 16;14:1299208. doi: 10.3389/fpls.2023.1299208. eCollection 2023. Front Plant Sci. 2024. PMID: 38293629 Free PMC article.
-
Density physics-informed neural networks reveal sources of cell heterogeneity in signal transduction.Patterns (N Y). 2023 Dec 26;5(2):100899. doi: 10.1016/j.patter.2023.100899. eCollection 2024 Feb 9. Patterns (N Y). 2023. PMID: 38370126 Free PMC article.
-
Unraveling biochemical spatial patterns: Machine learning approaches to the inverse problem of stationary Turing patterns.iScience. 2024 Apr 29;27(6):109822. doi: 10.1016/j.isci.2024.109822. eCollection 2024 Jun 21. iScience. 2024. PMID: 38827409 Free PMC article.
References
Publication types
MeSH terms
Grants and funding
LinkOut - more resources
Full Text Sources