Phenotypic Trade-Offs: Deciphering the Impact of Neurodiversity on Drug Development in Fragile X Syndrome
- PMID: 34733188
- PMCID: PMC8558248
- DOI: 10.3389/fpsyt.2021.730987
Phenotypic Trade-Offs: Deciphering the Impact of Neurodiversity on Drug Development in Fragile X Syndrome
Abstract
Fragile X syndrome (FXS) is the most common single-gene cause of intellectual disability and autism spectrum disorder. Individuals with FXS present with a wide range of severity in multiple phenotypes including cognitive delay, behavioral challenges, sleep issues, epilepsy, and anxiety. These symptoms are also shared by many individuals with other neurodevelopmental disorders (NDDs). Since the discovery of the FXS gene, FMR1, FXS has been the focus of intense preclinical investigation and is placed at the forefront of clinical trials in the field of NDDs. So far, most studies have aimed to translate the rescue of specific phenotypes in animal models, for example, learning, or improving general cognitive or behavioral functioning in individuals with FXS. Trial design, selection of outcome measures, and interpretation of results of recent trials have shown limitations in this type of approach. We propose a new paradigm in which all phenotypes involved in individuals with FXS would be considered and, more importantly, the possible interactions between these phenotypes. This approach would be implemented both at the baseline, meaning when entering a trial or when studying a patient population, and also after the intervention when the study subjects have been exposed to the investigational product. This approach would allow us to further understand potential trade-offs underlying the varying effects of the treatment on different individuals in clinical trials, and to connect the results to individual genetic differences. To better understand the interplay between different phenotypes, we emphasize the need for preclinical studies to investigate various interrelated biological and behavioral outcomes when assessing a specific treatment. In this paper, we present how such a conceptual shift in preclinical design could shed new light on clinical trial results. Future clinical studies should take into account the rich neurodiversity of individuals with FXS specifically and NDDs in general, and incorporate the idea of trade-offs in their designs.
Keywords: autism; clinical trials; fragile X syndrome; intellectual disability; neurodevelopmental disorder; neurodiversity; phenotype-genotype correlation; trade-off.
Copyright © 2021 Bui, Shatto, Cuppens, Droit and Bolduc.
Conflict of interest statement
The authors declare that the research was conducted in the absence of any commercial or financial relationships that could be construed as a potential conflict of interest.
Figures
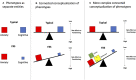
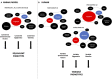
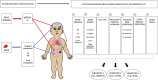
Similar articles
-
Emerging pharmacologic treatment options for fragile X syndrome.Appl Clin Genet. 2015 Apr 7;8:75-93. doi: 10.2147/TACG.S35673. eCollection 2015. Appl Clin Genet. 2015. PMID: 25897255 Free PMC article. Review.
-
Sensory Processing Phenotypes in Fragile X Syndrome.ASN Neuro. 2018 Jan-Dec;10:1759091418801092. doi: 10.1177/1759091418801092. ASN Neuro. 2018. PMID: 30231625 Free PMC article. Review.
-
Mechanism-based treatments in neurodevelopmental disorders: fragile X syndrome.Pediatr Neurol. 2014 Apr;50(4):297-302. doi: 10.1016/j.pediatrneurol.2013.12.001. Epub 2013 Dec 4. Pediatr Neurol. 2014. PMID: 24518745 Review.
-
Melatonin as a Novel Interventional Candidate for Fragile X Syndrome with Autism Spectrum Disorder in Humans.Int J Mol Sci. 2017 Jun 20;18(6):1314. doi: 10.3390/ijms18061314. Int J Mol Sci. 2017. PMID: 28632163 Free PMC article. Review.
-
Double Genetic Hit: Fragile X Syndrome and Partial Deletion of Protein Patched Homolog 1 Antisense as Cause of Severe Autism Spectrum Disorder.J Dev Behav Pediatr. 2020 Dec;41(9):724-728. doi: 10.1097/DBP.0000000000000850. J Dev Behav Pediatr. 2020. PMID: 32947579
Cited by
-
The feasibility and utility of hair follicle sampling to measure FMRP and FMR1 mRNA in children with or without fragile X syndrome: a pilot study.J Neurodev Disord. 2022 Dec 9;14(1):57. doi: 10.1186/s11689-022-09465-7. J Neurodev Disord. 2022. PMID: 36494616 Free PMC article.
References
LinkOut - more resources
Full Text Sources