Immune Response in Severe and Non-Severe Coronavirus Disease 2019 (COVID-19) Infection: A Mechanistic Landscape
- PMID: 34721400
- PMCID: PMC8548832
- DOI: 10.3389/fimmu.2021.738073
Immune Response in Severe and Non-Severe Coronavirus Disease 2019 (COVID-19) Infection: A Mechanistic Landscape
Abstract
The mechanisms underlying the immune remodeling and severity response in coronavirus disease 2019 (COVID-19) are yet to be fully elucidated. Our comprehensive integrative analyses of single-cell RNA sequencing (scRNAseq) data from four published studies, in patients with mild/moderate and severe infections, indicate a robust expansion and mobilization of the innate immune response and highlight mechanisms by which low-density neutrophils and megakaryocytes play a crucial role in the cross talk between lymphoid and myeloid lineages. We also document a marked reduction of several lymphoid cell types, particularly natural killer cells, mucosal-associated invariant T (MAIT) cells, and gamma-delta T (γδT) cells, and a robust expansion and extensive heterogeneity within plasmablasts, especially in severe COVID-19 patients. We confirm the changes in cellular abundances for certain immune cell types within a new patient cohort. While the cellular heterogeneity in COVID-19 extends across cells in both lineages, we consistently observe certain subsets respond more potently to interferon type I (IFN-I) and display increased cellular abundances across the spectrum of severity, as compared with healthy subjects. However, we identify these expanded subsets to have a more muted response to IFN-I within severe disease compared to non-severe disease. Our analyses further highlight an increased aggregation potential of the myeloid subsets, particularly monocytes, in COVID-19. Finally, we provide detailed mechanistic insights into the interaction between lymphoid and myeloid lineages, which contributes to the multisystemic phenotype of COVID-19, distinguishing severe from non-severe responses.
Keywords: COVID-19; MDSC; aggregation; cDC2; immune-remodeling; low-density neutrophils; megakaryocyte; plasmablast.
Copyright © 2021 Mukund, Nayak, Ashokkumar, Rao, Almeda, Betancourt-Garcia, Sindhi and Subramaniam.
Conflict of interest statement
Phenotyping of the independent cohort was performed at Plexision, a University of Pittsburgh spinoff in which RS and the University hold equity. RS and CA are currently employed at Plexision. The remaining authors declare that the research was conducted in the absence of any commercial or financial relationships that could be construed as a potential conflict of interest.
Figures

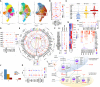
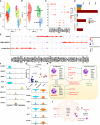
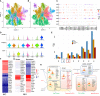
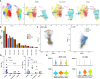
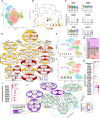
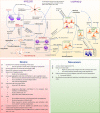
Similar articles
-
Immunopathology of Hyperinflammation in COVID-19.Am J Pathol. 2021 Jan;191(1):4-17. doi: 10.1016/j.ajpath.2020.08.009. Epub 2020 Sep 11. Am J Pathol. 2021. PMID: 32919977 Free PMC article. Review.
-
Identification of Distinct Immune Cell Subsets Associated With Asymptomatic Infection, Disease Severity, and Viral Persistence in COVID-19 Patients.Front Immunol. 2022 Feb 22;13:812514. doi: 10.3389/fimmu.2022.812514. eCollection 2022. Front Immunol. 2022. PMID: 35281000 Free PMC article.
-
Suppressive Monocytes Impair MAIT Cells Response via IL-10 in Patients with Severe COVID-19.J Immunol. 2021 Oct 1;207(7):1848-1856. doi: 10.4049/jimmunol.2100228. Epub 2021 Aug 27. J Immunol. 2021. PMID: 34452933
-
Mucosal-Associated Invariant T (MAIT) Cells Are Highly Activated and Functionally Impaired in COVID-19 Patients.Viruses. 2021 Feb 3;13(2):241. doi: 10.3390/v13020241. Viruses. 2021. PMID: 33546489 Free PMC article.
-
Natural killer cells and unconventional T cells in COVID-19.Curr Opin Virol. 2021 Aug;49:176-182. doi: 10.1016/j.coviro.2021.06.005. Epub 2021 Jun 19. Curr Opin Virol. 2021. PMID: 34217135 Free PMC article. Review.
Cited by
-
Mucosal immunity in COVID-19: a comprehensive review.Front Immunol. 2024 Aug 14;15:1433452. doi: 10.3389/fimmu.2024.1433452. eCollection 2024. Front Immunol. 2024. PMID: 39206184 Free PMC article. Review.
-
Emerging Trends in the Application of Extracellular Vesicles as Novel Oral Delivery Vehicles for Therapeutics in Inflammatory Diseases.Int J Nanomedicine. 2024 Aug 21;19:8573-8601. doi: 10.2147/IJN.S475532. eCollection 2024. Int J Nanomedicine. 2024. PMID: 39185348 Free PMC article. Review.
-
The role of dendritic cells in respiratory viral infection.Eur Respir Rev. 2024 May 29;33(172):230250. doi: 10.1183/16000617.0250-2023. Print 2024 Apr 30. Eur Respir Rev. 2024. PMID: 38811032 Free PMC article. Review.
-
Differential detection workflows for multi-sample single-cell RNA-seq data.bioRxiv [Preprint]. 2023 Dec 19:2023.12.17.572043. doi: 10.1101/2023.12.17.572043. bioRxiv. 2023. PMID: 38187695 Free PMC article. Preprint.
-
Convalescent Adaptive Immunity Is Highly Heterogenous after SARS-CoV-2 Infection.J Clin Med. 2023 Nov 16;12(22):7136. doi: 10.3390/jcm12227136. J Clin Med. 2023. PMID: 38002748 Free PMC article.
References
Publication types
MeSH terms
Substances
Grants and funding
LinkOut - more resources
Full Text Sources
Other Literature Sources
Medical
Miscellaneous