Predicting Short-Term Survival after Gross Total or Near Total Resection in Glioblastomas by Machine Learning-Based Radiomic Analysis of Preoperative MRI
- PMID: 34680199
- PMCID: PMC8533879
- DOI: 10.3390/cancers13205047
Predicting Short-Term Survival after Gross Total or Near Total Resection in Glioblastomas by Machine Learning-Based Radiomic Analysis of Preoperative MRI
Abstract
Radiomics, in combination with artificial intelligence, has emerged as a powerful tool for the development of predictive models in neuro-oncology. Our study aims to find an answer to a clinically relevant question: is there a radiomic profile that can identify glioblastoma (GBM) patients with short-term survival after complete tumor resection? A retrospective study of GBM patients who underwent surgery was conducted in two institutions between January 2019 and January 2020, along with cases from public databases. Cases with gross total or near total tumor resection were included. Preoperative structural multiparametric magnetic resonance imaging (mpMRI) sequences were pre-processed, and a total of 15,720 radiomic features were extracted. After feature reduction, machine learning-based classifiers were used to predict early mortality (<6 months). Additionally, a survival analysis was performed using the random survival forest (RSF) algorithm. A total of 203 patients were enrolled in this study. In the classification task, the naive Bayes classifier obtained the best results in the test data set, with an area under the curve (AUC) of 0.769 and classification accuracy of 80%. The RSF model allowed the stratification of patients into low- and high-risk groups. In the test data set, this model obtained values of C-Index = 0.61, IBS = 0.123 and integrated AUC at six months of 0.761. In this study, we developed a reliable predictive model of short-term survival in GBM by applying open-source and user-friendly computational means. These new tools will assist clinicians in adapting our therapeutic approach considering individual patient characteristics.
Keywords: glioblastoma; machine learning; radiomics; survival; texture analysis.
Conflict of interest statement
The authors declare no conflict of interest.
Figures
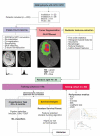
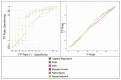
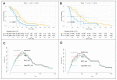
Similar articles
-
The Nomogram of MRI-based Radiomics with Complementary Visual Features by Machine Learning Improves Stratification of Glioblastoma Patients: A Multicenter Study.J Magn Reson Imaging. 2021 Aug;54(2):571-583. doi: 10.1002/jmri.27536. Epub 2021 Feb 8. J Magn Reson Imaging. 2021. PMID: 33559302
-
Magnetic resonance imaging radiomics predicts preoperative axillary lymph node metastasis to support surgical decisions and is associated with tumor microenvironment in invasive breast cancer: A machine learning, multicenter study.EBioMedicine. 2021 Jul;69:103460. doi: 10.1016/j.ebiom.2021.103460. Epub 2021 Jul 4. EBioMedicine. 2021. PMID: 34233259 Free PMC article. Clinical Trial.
-
Comparison of machine learning algorithms to predict clinically significant prostate cancer of the peripheral zone with multiparametric MRI using clinical assessment categories and radiomic features.Eur Radiol. 2020 Dec;30(12):6757-6769. doi: 10.1007/s00330-020-07064-5. Epub 2020 Jul 16. Eur Radiol. 2020. PMID: 32676784 Free PMC article.
-
Artificial intelligence in the radiomic analysis of glioblastomas: A review, taxonomy, and perspective.Front Oncol. 2022 Aug 2;12:924245. doi: 10.3389/fonc.2022.924245. eCollection 2022. Front Oncol. 2022. PMID: 35982952 Free PMC article. Review.
-
Radiomics in the diagnosis of glioblastoma.Pol J Radiol. 2023 Oct 6;88:e461-e466. doi: 10.5114/pjr.2023.132168. eCollection 2023. Pol J Radiol. 2023. PMID: 38020501 Free PMC article. Review.
Cited by
-
Current Evidence, Limitations and Future Challenges of Survival Prediction for Glioblastoma Based on Advanced Noninvasive Methods: A Narrative Review.Medicina (Kaunas). 2022 Nov 29;58(12):1746. doi: 10.3390/medicina58121746. Medicina (Kaunas). 2022. PMID: 36556948 Free PMC article. Review.
-
Forecasting Molecular Features in IDH-Wildtype Gliomas: The State of the Art of Radiomics Applied to Neurosurgery.Cancers (Basel). 2023 Feb 2;15(3):940. doi: 10.3390/cancers15030940. Cancers (Basel). 2023. PMID: 36765898 Free PMC article. Review.
-
Time-to-event overall survival prediction in glioblastoma multiforme patients using magnetic resonance imaging radiomics.Radiol Med. 2023 Dec;128(12):1521-1534. doi: 10.1007/s11547-023-01725-3. Epub 2023 Sep 26. Radiol Med. 2023. PMID: 37751102 Free PMC article.
-
Prediction of Rapid Early Progression and Survival Risk with Pre-Radiation MRI in WHO Grade 4 Glioma Patients.Cancers (Basel). 2023 Sep 19;15(18):4636. doi: 10.3390/cancers15184636. Cancers (Basel). 2023. PMID: 37760604 Free PMC article.
-
Radiomics and Machine Learning in Brain Tumors and Their Habitat: A Systematic Review.Cancers (Basel). 2023 Jul 28;15(15):3845. doi: 10.3390/cancers15153845. Cancers (Basel). 2023. PMID: 37568660 Free PMC article. Review.
References
-
- Stupp R., Hegi M.E., Mason W.P., van den Bent M.J., Taphoorn M.J.B., Janzer R.C., Ludwin S.K., Allgeier A., Fisher B., Belanger K., et al. Effects of radiotherapy with concomitant and adjuvant temozolomide versus radiotherapy alone on survival in glioblastoma in a randomised phase III study: 5-year analysis of the EORTC-NCIC trial. Lancet. Oncol. 2009;10:459–466. doi: 10.1016/S1470-2045(09)70025-7. - DOI - PubMed
-
- Gutman D.A., Cooper L.A.D., Hwang S.N., Holder C.A., Gao J., Aurora T.D., Dunn W.D., Scarpace L., Mikkelsen T., Jain R., et al. MR Imaging predictors of molecular profile and survival: Multi-institutional Study of the TCGA Glioblastoma Data Set. Radiology. 2013;267:560–569. doi: 10.1148/radiol.13120118. - DOI - PMC - PubMed
LinkOut - more resources
Full Text Sources