Exome sequencing and analysis of 454,787 UK Biobank participants
- PMID: 34662886
- PMCID: PMC8596853
- DOI: 10.1038/s41586-021-04103-z
Exome sequencing and analysis of 454,787 UK Biobank participants
Abstract
A major goal in human genetics is to use natural variation to understand the phenotypic consequences of altering each protein-coding gene in the genome. Here we used exome sequencing1 to explore protein-altering variants and their consequences in 454,787 participants in the UK Biobank study2. We identified 12 million coding variants, including around 1 million loss-of-function and around 1.8 million deleterious missense variants. When these were tested for association with 3,994 health-related traits, we found 564 genes with trait associations at P ≤ 2.18 × 10-11. Rare variant associations were enriched in loci from genome-wide association studies (GWAS), but most (91%) were independent of common variant signals. We discovered several risk-increasing associations with traits related to liver disease, eye disease and cancer, among others, as well as risk-lowering associations for hypertension (SLC9A3R2), diabetes (MAP3K15, FAM234A) and asthma (SLC27A3). Six genes were associated with brain imaging phenotypes, including two involved in neural development (GBE1, PLD1). Of the signals available and powered for replication in an independent cohort, 81% were confirmed; furthermore, association signals were generally consistent across individuals of European, Asian and African ancestry. We illustrate the ability of exome sequencing to identify gene-trait associations, elucidate gene function and pinpoint effector genes that underlie GWAS signals at scale.
© 2021. The Author(s).
Conflict of interest statement
J.D.B., A.H.L., A.M., D.S., J. Mbatchou, C.E.G., D.L., A.E.L., S.B., A.Y., N.B., M.D.K., A.D., S.L., C.B., X.B., A.H., E.M., L.G., K.W., J.A.K., V.R., J. Mighty, M.J., L.M., G.C., E.J., L.H., W.J.S., A.R.S., L.A.L., J.D.O., M.N.C., J.G.R., G.Y., H.M.K., J. Marchini, A.B., G.R.A. and M.A.R.F. are current employees and/or stockholders of Regeneron Genetics Center or Regeneron Pharmaceuticals.
Figures
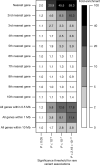
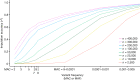
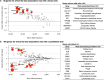
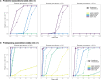
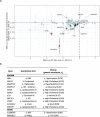
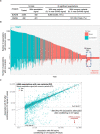
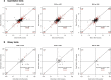
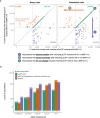
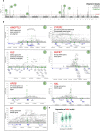
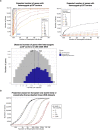
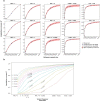
Comment in
-
A massive effort links protein-coding gene variants to health.Nature. 2021 Nov;599(7886):561-563. doi: 10.1038/d41586-021-02873-0. Nature. 2021. PMID: 34697483 No abstract available.
Similar articles
-
Rare variant contribution to human disease in 281,104 UK Biobank exomes.Nature. 2021 Sep;597(7877):527-532. doi: 10.1038/s41586-021-03855-y. Epub 2021 Aug 10. Nature. 2021. PMID: 34375979 Free PMC article.
-
Efficient identification of trait-associated loss-of-function variants in the UK Biobank cohort by exome-sequencing based genotype imputation.Genet Epidemiol. 2023 Mar;47(2):121-134. doi: 10.1002/gepi.22511. Epub 2022 Dec 9. Genet Epidemiol. 2023. PMID: 36490288
-
Rare and Coding Region Genetic Variants Associated With Risk of Ischemic Stroke: The NHLBI Exome Sequence Project.JAMA Neurol. 2015 Jul;72(7):781-8. doi: 10.1001/jamaneurol.2015.0582. JAMA Neurol. 2015. PMID: 25961151 Free PMC article.
-
Advancing human genetics research and drug discovery through exome sequencing of the UK Biobank.Nat Genet. 2021 Jul;53(7):942-948. doi: 10.1038/s41588-021-00885-0. Epub 2021 Jun 28. Nat Genet. 2021. PMID: 34183854 Review.
-
Molecular genetic studies of complex phenotypes.Transl Res. 2012 Feb;159(2):64-79. doi: 10.1016/j.trsl.2011.08.001. Epub 2011 Aug 31. Transl Res. 2012. PMID: 22243791 Free PMC article. Review.
Cited by
-
Whole exome sequencing analysis identifies genes for alcohol consumption.Nat Commun. 2024 Jul 10;15(1):5777. doi: 10.1038/s41467-024-50132-3. Nat Commun. 2024. PMID: 38982111 Free PMC article.
-
Resolving intra-repeat variation in medically relevant VNTRs from short-read sequencing data using the cardiovascular risk gene LPA as a model.Genome Biol. 2024 Jun 26;25(1):167. doi: 10.1186/s13059-024-03316-5. Genome Biol. 2024. PMID: 38926899 Free PMC article.
-
A cost-effective sequencing method for genetic studies combining high-depth whole exome and low-depth whole genome.NPJ Genom Med. 2024 Feb 7;9(1):8. doi: 10.1038/s41525-024-00390-3. NPJ Genom Med. 2024. PMID: 38326393 Free PMC article.
-
Performing highly parallelized and reproducible GWAS analysis on biobank-scale data.NAR Genom Bioinform. 2024 Feb 7;6(1):lqae015. doi: 10.1093/nargab/lqae015. eCollection 2024 Mar. NAR Genom Bioinform. 2024. PMID: 38327871 Free PMC article.
-
Prevalence, Cardiac Phenotype, and Outcomes of Transthyretin Variants in the UK Biobank Population.JAMA Cardiol. 2024 Nov 1;9(11):964-972. doi: 10.1001/jamacardio.2024.2190. JAMA Cardiol. 2024. PMID: 39196575
References
-
- Szustakowski JD, et al. Advancing human genetics research and drug discovery through exome sequencing of the UK Biobank. Nat. Genet. 2021;53:942–948. - PubMed
Publication types
MeSH terms
Grants and funding
LinkOut - more resources
Full Text Sources
Other Literature Sources
Research Materials
Miscellaneous