Signatures of plasticity, metastasis, and immunosuppression in an atlas of human small cell lung cancer
- PMID: 34653364
- PMCID: PMC8628860
- DOI: 10.1016/j.ccell.2021.09.008
Signatures of plasticity, metastasis, and immunosuppression in an atlas of human small cell lung cancer
Abstract
Small cell lung cancer (SCLC) is an aggressive malignancy that includes subtypes defined by differential expression of ASCL1, NEUROD1, and POU2F3 (SCLC-A, -N, and -P, respectively). To define the heterogeneity of tumors and their associated microenvironments across subtypes, we sequenced 155,098 transcriptomes from 21 human biospecimens, including 54,523 SCLC transcriptomes. We observe greater tumor diversity in SCLC than lung adenocarcinoma, driven by canonical, intermediate, and admixed subtypes. We discover a PLCG2-high SCLC phenotype with stem-like, pro-metastatic features that recurs across subtypes and predicts worse overall survival. SCLC exhibits greater immune sequestration and less immune infiltration than lung adenocarcinoma, and SCLC-N shows less immune infiltrate and greater T cell dysfunction than SCLC-A. We identify a profibrotic, immunosuppressive monocyte/macrophage population in SCLC tumors that is particularly associated with the recurrent, PLCG2-high subpopulation.
Keywords: PLCG2; SCLC; metastasis; myeloid; scRNA-seq; single cell; tumor atlas.
Copyright © 2021 Elsevier Inc. All rights reserved.
Conflict of interest statement
Declaration of interests J.M.C. reports an advisory role in VantAI. A.Q.-V. reports honoraria from AstraZeneca. M.O. reports advisory roles for PharMar, Novartis, and Targeted Oncology, and reports honoraria from Bristol-Myers Squibb and Merck Sharp & Dohme. C.M.R. has consulted regarding oncology drug development with AbbVie, Amgen, Ascentage, AstraZeneca, Bicycle, Celgene, Daiichi Sankyo, Genentech/Roche, Ipsen, Jazz, Lilly, Pfizer, PharmaMar, Syros, and Vavotek. C.M.R. serves on the scientific advisory boards of Bridge Medicines, Earli, and Harpoon Therapeutics.
Figures
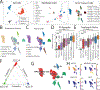
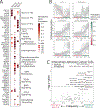
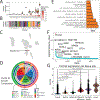
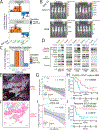
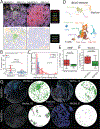
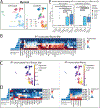
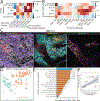
Similar articles
-
Expression patterns and prognostic relevance of subtype-specific transcription factors in surgically resected small-cell lung cancer: an international multicenter study.J Pathol. 2022 Aug;257(5):674-686. doi: 10.1002/path.5922. Epub 2022 May 25. J Pathol. 2022. PMID: 35489038 Free PMC article.
-
Clinical characteristics and patient outcomes of molecular subtypes of small cell lung cancer (SCLC).World J Surg Oncol. 2022 Feb 27;20(1):54. doi: 10.1186/s12957-022-02528-y. World J Surg Oncol. 2022. PMID: 35220975 Free PMC article.
-
Comparison of ASCL1, NEUROD1, and POU2F3 expression in surgically resected specimens, paired tissue microarrays, and lymph node metastases in small cell lung carcinoma.Histopathology. 2023 May;82(6):860-869. doi: 10.1111/his.14872. Epub 2023 Feb 14. Histopathology. 2023. PMID: 36715573
-
Small cell lung cancer: Subtypes and therapeutic implications.Semin Cancer Biol. 2022 Nov;86(Pt 2):543-554. doi: 10.1016/j.semcancer.2022.04.001. Epub 2022 Apr 6. Semin Cancer Biol. 2022. PMID: 35398266 Review.
-
Small cell lung cancer profiling: an updated synthesis of subtypes, vulnerabilities, and plasticity.Trends Cancer. 2024 Oct;10(10):935-946. doi: 10.1016/j.trecan.2024.07.008. Epub 2024 Aug 19. Trends Cancer. 2024. PMID: 39164163 Review.
Cited by
-
Pulmonary cancers across different histotypes share hybrid tuft cell/ionocyte-like molecular features and potentially druggable vulnerabilities.Cell Death Dis. 2022 Nov 19;13(11):979. doi: 10.1038/s41419-022-05428-x. Cell Death Dis. 2022. PMID: 36402755 Free PMC article.
-
Exportin 1 inhibition prevents neuroendocrine transformation through SOX2 down-regulation in lung and prostate cancers.Sci Transl Med. 2023 Aug 2;15(707):eadf7006. doi: 10.1126/scitranslmed.adf7006. Epub 2023 Aug 2. Sci Transl Med. 2023. PMID: 37531417 Free PMC article.
-
C-Myc-induced hypersialylation of small cell lung cancer facilitates pro-tumoral phenotypes of macrophages.iScience. 2023 Aug 29;26(10):107771. doi: 10.1016/j.isci.2023.107771. eCollection 2023 Oct 20. iScience. 2023. PMID: 37731607 Free PMC article.
-
Single-cell landscape of primary central nervous system diffuse large B-cell lymphoma.Cell Discov. 2023 Jun 12;9(1):55. doi: 10.1038/s41421-023-00559-7. Cell Discov. 2023. PMID: 37308475 Free PMC article.
-
Conserved transcriptional connectivity of regulatory T cells in the tumor microenvironment informs new combination cancer therapy strategies.Nat Immunol. 2023 Jun;24(6):1020-1035. doi: 10.1038/s41590-023-01504-2. Epub 2023 May 1. Nat Immunol. 2023. PMID: 37127830 Free PMC article.
References
-
- Patel Anoop P,*1, 2, 3, 4 Itay Tirosh,*3 Trombetta John J., 3Alex K. Shalek, 3 Gillespie Shawn M., 2, 3, 4 Wakimoto Hiroaki, 1 Cahill Daniel P., 1 Nahed Brian V., 1 Curry William T., 1 Martuza Robert L., 1 Louis David N., 2 Rozenblatt-Rosen Orit, 3 Mari, 4†‡, and Human (2014). R es e a rc h | r e po r ts. Science (80-. ). 344, 1396–1402. - PMC - PubMed
Publication types
MeSH terms
Substances
Grants and funding
LinkOut - more resources
Full Text Sources
Other Literature Sources
Medical