On Deep Landscape Exploration of COVID-19 Patients Cells and Severity Markers
- PMID: 34603282
- PMCID: PMC8481922
- DOI: 10.3389/fimmu.2021.705646
On Deep Landscape Exploration of COVID-19 Patients Cells and Severity Markers
Abstract
COVID-19 is a disease with a spectrum of clinical responses ranging from moderate to critical. To study and control its effects, a large number of researchers are focused on two substantial aims. On the one hand, the discovery of diverse biomarkers to classify and potentially anticipate the disease severity of patients. These biomarkers could serve as a medical criterion to prioritize attention to those patients with higher prone to severe responses. On the other hand, understanding how the immune system orchestrates its responses in this spectrum of disease severities is a fundamental issue required to design new and optimized therapeutic strategies. In this work, using single-cell RNAseq of bronchoalveolar lavage fluid of nine patients with COVID-19 and three healthy controls, we contribute to both aspects. First, we presented computational supervised machine-learning models with high accuracy in classifying the disease severity (moderate and severe) in patients with COVID-19 starting from single-cell data from bronchoalveolar lavage fluid. Second, we identified regulatory mechanisms from the heterogeneous cell populations in the lungs microenvironment that correlated with different clinical responses. Given the results, patients with moderate COVID-19 symptoms showed an activation/inactivation profile for their analyzed cells leading to a sequential and innocuous immune response. In comparison, severe patients might be promoting cytotoxic and pro-inflammatory responses in a systemic fashion involving epithelial and immune cells without the possibility to develop viral clearance and immune memory. Consequently, we present an in-depth landscape analysis of how transcriptional factors and pathways from these heterogeneous populations can regulate their expression to promote or restrain an effective immune response directly linked to the patients prognosis.
Keywords: COVID-19; cell heterogeneity; immune landscape; immune system; machine-learning; single-cell analysis.
Copyright © 2021 Vázquez-Jiménez, Avila-Ponce De León, Matadamas-Guzman, Muciño-Olmos, Martínez-López, Escobedo-Tapia and Resendis-Antonio.
Conflict of interest statement
The authors declare that the research was conducted in the absence of any commercial or financial relationships that could be construed as a potential conflict of interest.
Figures
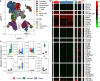
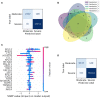
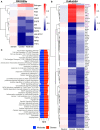
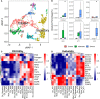
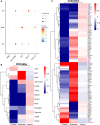
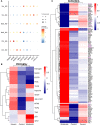
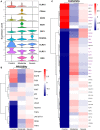
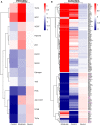
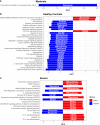
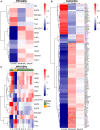
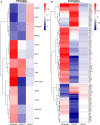
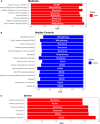
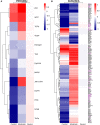
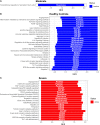
Similar articles
-
SARS-CoV-2 activates lung epithelial cell proinflammatory signaling and leads to immune dysregulation in COVID-19 patients.EBioMedicine. 2021 Aug;70:103500. doi: 10.1016/j.ebiom.2021.103500. Epub 2021 Jul 23. EBioMedicine. 2021. PMID: 34311326 Free PMC article.
-
Single-Cell Transcriptome Analysis Highlights a Role for Neutrophils and Inflammatory Macrophages in the Pathogenesis of Severe COVID-19.Cells. 2020 Oct 29;9(11):2374. doi: 10.3390/cells9112374. Cells. 2020. PMID: 33138195 Free PMC article.
-
Single-cell analysis reveals cell communication triggered by macrophages associated with the reduction and exhaustion of CD8+ T cells in COVID-19.Cell Commun Signal. 2021 Jul 8;19(1):73. doi: 10.1186/s12964-021-00754-7. Cell Commun Signal. 2021. PMID: 34238338 Free PMC article.
-
[Deep lung--cellular reaction to HIV].Rev Port Pneumol. 2007 Mar-Apr;13(2):175-212. Rev Port Pneumol. 2007. PMID: 17492233 Review. Portuguese.
-
How could we forget immunometabolism in SARS-CoV2 infection or COVID-19?Int Rev Immunol. 2021;40(1-2):72-107. doi: 10.1080/08830185.2020.1840567. Epub 2020 Nov 6. Int Rev Immunol. 2021. PMID: 33155525 Review.
Cited by
-
Uncoding the interdependency of tumor microenvironment and macrophage polarization: insights from a continuous network approach.Front Immunol. 2023 May 22;14:1150890. doi: 10.3389/fimmu.2023.1150890. eCollection 2023. Front Immunol. 2023. PMID: 37283734 Free PMC article.
-
Single-cell RNA-seq methods to interrogate virus-host interactions.Semin Immunopathol. 2023 Jan;45(1):71-89. doi: 10.1007/s00281-022-00972-2. Epub 2022 Nov 21. Semin Immunopathol. 2023. PMID: 36414692 Free PMC article. Review.
-
Macrophage Boolean networks in the time of SARS-CoV-2.Front Immunol. 2022 Oct 17;13:997434. doi: 10.3389/fimmu.2022.997434. eCollection 2022. Front Immunol. 2022. PMID: 36325317 Free PMC article. No abstract available.
-
The Spike Protein of SARS-CoV-2 Is Adapting Because of Selective Pressures.Vaccines (Basel). 2022 May 28;10(6):864. doi: 10.3390/vaccines10060864. Vaccines (Basel). 2022. PMID: 35746472 Free PMC article.
-
Identification of Gene Markers Associated with COVID-19 Severity and Recovery in Different Immune Cell Subtypes.Biology (Basel). 2023 Jul 2;12(7):947. doi: 10.3390/biology12070947. Biology (Basel). 2023. PMID: 37508378 Free PMC article.
References
MeSH terms
Substances
LinkOut - more resources
Full Text Sources
Medical