Differential RNA methylation using multivariate statistical methods
- PMID: 34586372
- PMCID: PMC8974314
- DOI: 10.1093/bib/bbab309
Differential RNA methylation using multivariate statistical methods
Abstract
Motivation: m6A methylation is a highly prevalent post-transcriptional modification in eukaryotes. MeRIP-seq or m6A-seq, which comprises immunoprecipitation of methylation fragments , is the most common method for measuring methylation signals. Existing computational tools for analyzing MeRIP-seq data sets and identifying differentially methylated genes/regions are not most optimal. They either ignore the sparsity or dependence structure of the methylation signals within a gene/region. Modeling the methylation signals using univariate distributions could also lead to high type I error rates and low sensitivity. In this paper, we propose using mean vector testing (MVT) procedures for testing differential methylation of RNA at the gene level. MVTs use a distribution-free test statistic with proven ability to control type I error even for extremely small sample sizes. We performed a comprehensive simulation study comparing the MVTs to existing MeRIP-seq data analysis tools. Comparative analysis of existing MeRIP-seq data sets is presented to illustrate the advantage of using MVTs.
Results: Mean vector testing procedures are observed to control type I error rate and achieve high power for detecting differential RNA methylation using m6A-seq data. Results from two data sets indicate that the genes detected identified as having different m6A methylation patterns have high functional relevance to the study conditions.
Availability: The dimer software package for differential RNA methylation analysis is freely available at https://github.com/ouyang-lab/DIMER.
Supplementary information: Supplementary data are available at Briefings in Bioinformatics online.
Keywords: RNA methylation; differential analysis; statistical methods.
© The Author(s) 2021. Published by Oxford University Press. All rights reserved. For Permissions, please email: journals.permissions@oup.com.
Figures
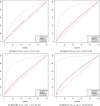
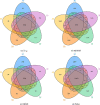
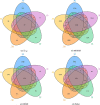
Similar articles
-
Detecting m6A methylation regions from Methylated RNA Immunoprecipitation Sequencing.Bioinformatics. 2021 Sep 29;37(18):2818-2824. doi: 10.1093/bioinformatics/btab181. Bioinformatics. 2021. PMID: 33724304 Free PMC article.
-
A protocol for RNA methylation differential analysis with MeRIP-Seq data and exomePeak R/Bioconductor package.Methods. 2014 Oct 1;69(3):274-81. doi: 10.1016/j.ymeth.2014.06.008. Epub 2014 Jun 27. Methods. 2014. PMID: 24979058 Free PMC article.
-
Differential RNA methylation analysis for MeRIP-seq data under general experimental design.Bioinformatics. 2022 Oct 14;38(20):4705-4712. doi: 10.1093/bioinformatics/btac601. Bioinformatics. 2022. PMID: 36063045 Free PMC article.
-
RADAR: differential analysis of MeRIP-seq data with a random effect model.Genome Biol. 2019 Dec 23;20(1):294. doi: 10.1186/s13059-019-1915-9. Genome Biol. 2019. PMID: 31870409 Free PMC article.
-
The role of m6A methylation in osteosarcoma biological processes and its potential clinical value.Hum Genomics. 2022 Apr 18;16(1):12. doi: 10.1186/s40246-022-00384-1. Hum Genomics. 2022. PMID: 35436972 Free PMC article. Review.
Cited by
-
Analysis approaches for the identification and prediction of N6-methyladenosine sites.Epigenetics. 2023 Dec;18(1):2158284. doi: 10.1080/15592294.2022.2158284. Epub 2022 Dec 23. Epigenetics. 2023. PMID: 36562485 Free PMC article. Review.
References
-
- Altham PME. Two generalizations of the binomial distribution. J R Stat Soc Ser C Appl Stat 1978; 27(2): 162–7.
-
- Chen SX, Qin Y. A two-sample test for high-dimensional data with applications to gene-set testing. Ann Stat 2010; 38(2): 808–35.
Publication types
MeSH terms
Substances
Grants and funding
LinkOut - more resources
Full Text Sources
Miscellaneous