Integrating Spatiotemporal Epidemiology, Eco-Phylogenetics, and Distributional Ecology to Assess West Nile Disease Risk in Horses
- PMID: 34578392
- PMCID: PMC8473291
- DOI: 10.3390/v13091811
Integrating Spatiotemporal Epidemiology, Eco-Phylogenetics, and Distributional Ecology to Assess West Nile Disease Risk in Horses
Abstract
Mosquito-borne West Nile virus (WNV) is the causative agent of West Nile disease in humans, horses, and some bird species. Since the initial introduction of WNV to the United States (US), approximately 30,000 horses have been impacted by West Nile neurologic disease and hundreds of additional horses are infected each year. Research describing the drivers of West Nile disease in horses is greatly needed to better anticipate the spatial and temporal extent of disease risk, improve disease surveillance, and alleviate future economic impacts to the equine industry and private horse owners. To help meet this need, we integrated techniques from spatiotemporal epidemiology, eco-phylogenetics, and distributional ecology to assess West Nile disease risk in horses throughout the contiguous US. Our integrated approach considered horse abundance and virus exposure, vector and host distributions, and a variety of extrinsic climatic, socio-economic, and environmental risk factors. Birds are WNV reservoir hosts, and therefore we quantified avian host community dynamics across the continental US to show intra-annual variability in host phylogenetic structure and demonstrate host phylodiversity as a mechanism for virus amplification in time and virus dilution in space. We identified drought as a potential amplifier of virus transmission and demonstrated the importance of accounting for spatial non-stationarity when quantifying interaction between disease risk and meteorological influences such as temperature and precipitation. Our results delineated the timing and location of several areas at high risk of West Nile disease and can be used to prioritize vaccination programs and optimize virus surveillance and monitoring.
Keywords: Bayesian; West Nile virus; avian reservoir; disease biogeography; eco-phylogenetics; equine; horses; mosquito; spatial non-stationarity.
Conflict of interest statement
The authors declare no conflict of interest.
Figures
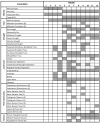
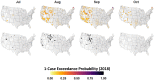
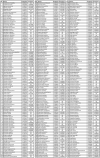
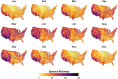
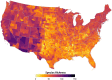
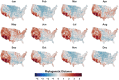
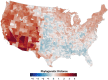
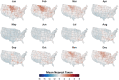
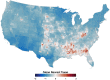
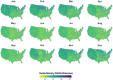
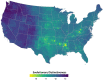
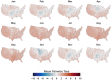
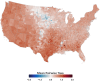
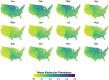
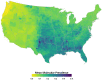
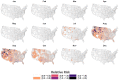
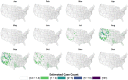
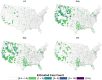
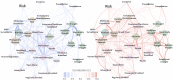
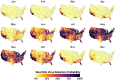
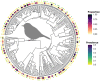
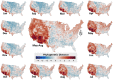
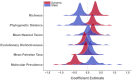
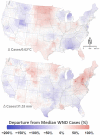
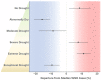
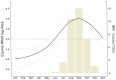
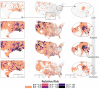
Similar articles
-
An Integrative Eco-Epidemiological Analysis of West Nile Virus Transmission.Ecohealth. 2017 Sep;14(3):474-489. doi: 10.1007/s10393-017-1249-6. Epub 2017 Jun 5. Ecohealth. 2017. PMID: 28584951 Free PMC article.
-
Transmission dynamics and changing epidemiology of West Nile virus.Anim Health Res Rev. 2008 Jun;9(1):71-86. doi: 10.1017/S1466252307001430. Epub 2008 Mar 19. Anim Health Res Rev. 2008. PMID: 18348742 Review.
-
The human and animal health impacts of introduction and spread of an exotic strain of West Nile virus in Australia.Prev Vet Med. 2013 May 1;109(3-4):186-204. doi: 10.1016/j.prevetmed.2012.09.018. Epub 2012 Oct 23. Prev Vet Med. 2013. PMID: 23098914
-
Integrated analysis of human-animal-vector surveillance: West Nile virus infections in Austria, 2015-2016.Emerg Microbes Infect. 2018 Mar 14;7(1):25. doi: 10.1038/s41426-018-0021-5. Emerg Microbes Infect. 2018. PMID: 29535293 Free PMC article.
-
West Nile Disease Epidemiology in North-West Africa: Bibliographical Review.Transbound Emerg Dis. 2016 Dec;63(6):e153-e159. doi: 10.1111/tbed.12341. Epub 2015 Mar 6. Transbound Emerg Dis. 2016. PMID: 25753775 Review.
Cited by
-
Impact of climate change on the global circulation of West Nile virus and adaptation responses: a scoping review.Infect Dis Poverty. 2024 May 24;13(1):38. doi: 10.1186/s40249-024-01207-2. Infect Dis Poverty. 2024. PMID: 38790027 Free PMC article.
-
Modeling the 2014-2015 Vesicular Stomatitis Outbreak in the United States Using an SEIR-SEI Approach.Viruses. 2024 Aug 18;16(8):1315. doi: 10.3390/v16081315. Viruses. 2024. PMID: 39205289 Free PMC article.
-
Interrogating Genomes and Geography to Unravel Multiyear Vesicular Stomatitis Epizootics.Viruses. 2024 Jul 11;16(7):1118. doi: 10.3390/v16071118. Viruses. 2024. PMID: 39066280 Free PMC article.
References
Publication types
MeSH terms
LinkOut - more resources
Full Text Sources
Medical