A combination approach of pseudotime analysis and mathematical modeling for understanding drug-resistant mechanisms
- PMID: 34531471
- PMCID: PMC8445918
- DOI: 10.1038/s41598-021-97887-z
A combination approach of pseudotime analysis and mathematical modeling for understanding drug-resistant mechanisms
Abstract
Cancer cells acquire drug resistance through the following stages: nonresistant, pre-resistant, and resistant. Although the molecular mechanism of drug resistance is well investigated, the process of drug resistance acquisition remains largely unknown. Here we elucidate the molecular mechanisms underlying the process of drug resistance acquisition by sequential analysis of gene expression patterns in tamoxifen-treated breast cancer cells. Single-cell RNA-sequencing indicates that tamoxifen-resistant cells can be subgrouped into two, one showing altered gene expression related to metabolic regulation and another showing high expression levels of adhesion-related molecules and histone-modifying enzymes. Pseudotime analysis showed a cell transition trajectory to the two resistant subgroups that stem from a shared pre-resistant state. An ordinary differential equation model based on the trajectory fitted well with the experimental results of cell growth. Based on the established model, it was predicted and experimentally validated that inhibition of transition to both resistant subtypes would prevent the appearance of tamoxifen resistance.
© 2021. The Author(s).
Conflict of interest statement
The authors declare no competing interests.
Figures
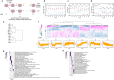
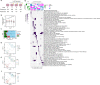
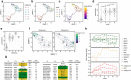
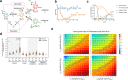
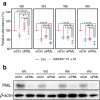
Similar articles
-
Integrative analyses of gene expression and DNA methylation profiles in breast cancer cell line models of tamoxifen-resistance indicate a potential role of cells with stem-like properties.Breast Cancer Res. 2013 Dec 19;15(6):R119. doi: 10.1186/bcr3588. Breast Cancer Res. 2013. PMID: 24355041 Free PMC article.
-
Histone methyltransferases regulate the transcriptional expression of ERα and the proliferation of tamoxifen-resistant breast cancer cells.Breast Cancer Res Treat. 2020 Feb;180(1):45-54. doi: 10.1007/s10549-019-05517-0. Epub 2020 Jan 2. Breast Cancer Res Treat. 2020. PMID: 31897900 Free PMC article.
-
Association of tamoxifen resistance and lipid reprogramming in breast cancer.BMC Cancer. 2018 Aug 24;18(1):850. doi: 10.1186/s12885-018-4757-z. BMC Cancer. 2018. PMID: 30143015 Free PMC article.
-
GPER mediates decreased chemosensitivity via regulation of ABCG2 expression and localization in tamoxifen-resistant breast cancer cells.Mol Cell Endocrinol. 2020 Apr 15;506:110762. doi: 10.1016/j.mce.2020.110762. Epub 2020 Feb 19. Mol Cell Endocrinol. 2020. PMID: 32087276
-
Tamoxifen resistance and epigenetic modifications in breast cancer cell lines.Curr Med Chem. 2007;14(28):3035-45. doi: 10.2174/092986707782794023. Curr Med Chem. 2007. PMID: 18220739 Free PMC article. Review.
Cited by
-
Transplantation of Human Brain-Derived Ischemia-Induced Multipotent Stem Cells Ameliorates Neurological Dysfunction in Mice After Stroke.Stem Cells Transl Med. 2023 Jun 15;12(6):400-414. doi: 10.1093/stcltm/szad031. Stem Cells Transl Med. 2023. PMID: 37221140 Free PMC article.
-
Identifying Key Regulatory Genes in Drug Resistance Acquisition: Modeling Pseudotime Trajectories of Breast Cancer Single-Cell Transcriptome.Cancers (Basel). 2024 May 15;16(10):1884. doi: 10.3390/cancers16101884. Cancers (Basel). 2024. PMID: 38791962 Free PMC article.
-
Proteomic time course of breast cancer cells highlights enhanced sensitivity to Stat3 and Src inhibitors prior to endocrine resistance development.Cancer Gene Ther. 2023 Feb;30(2):324-334. doi: 10.1038/s41417-022-00548-0. Epub 2022 Oct 20. Cancer Gene Ther. 2023. PMID: 36266450 Free PMC article.
References
Publication types
MeSH terms
Substances
LinkOut - more resources
Full Text Sources
Medical