Machine-Learned Molecular Surface and Its Application to Implicit Solvent Simulations
- PMID: 34516109
- PMCID: PMC9132718
- DOI: 10.1021/acs.jctc.1c00492
Machine-Learned Molecular Surface and Its Application to Implicit Solvent Simulations
Abstract
Implicit solvent models, such as Poisson-Boltzmann models, play important roles in computational studies of biomolecules. A vital step in almost all implicit solvent models is to determine the solvent-solute interface, and the solvent excluded surface (SES) is the most widely used interface definition in these models. However, classical algorithms used for computing SES are geometry-based, so that they are neither suitable for parallel implementations nor convenient for obtaining surface derivatives. To address the limitations, we explored a machine learning strategy to obtain a level set formulation for the SES. The training process was conducted in three steps, eventually leading to a model with over 95% agreement with the classical SES. Visualization of tested molecular surfaces shows that the machine-learned SES overlaps with the classical SES in almost all situations. Further analyses show that the machine-learned SES is incredibly stable in terms of rotational variation of tested molecules. Our timing analysis shows that the machine-learned SES is roughly 2.5 times as efficient as the classical SES routine implemented in Amber/PBSA on a tested central processing unit (CPU) platform. We expect further performance gain on massively parallel platforms such as graphics processing units (GPUs) given the ease in converting the machine-learned SES to a parallel procedure. We also implemented the machine-learned SES into the Amber/PBSA program to study its performance on reaction field energy calculation. The analysis shows that the two sets of reaction field energies are highly consistent with a 1% deviation on average. Given its level set formulation, we expect the machine-learned SES to be applied in molecular simulations that require either surface derivatives or high efficiency on parallel computing platforms.
Conflict of interest statement
The authors declare no competing financial interest.
Figures
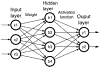
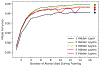
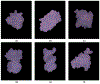
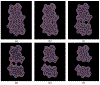
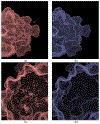
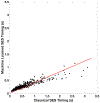
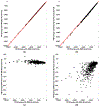
Similar articles
-
On-the-fly Numerical Surface Integration for Finite-Difference Poisson-Boltzmann Methods.J Chem Theory Comput. 2011 Nov 1;7(11):3608-3619. doi: 10.1021/ct200389p. J Chem Theory Comput. 2011. PMID: 24772042 Free PMC article.
-
Optimizing the Poisson Dielectric Boundary with Explicit Solvent Forces and Energies: Lessons Learned with Atom-Centered Dielectric Functions.J Chem Theory Comput. 2007 Jan;3(1):170-83. doi: 10.1021/ct600216k. J Chem Theory Comput. 2007. PMID: 26627162
-
Comparison of the MSMS and NanoShaper molecular surface triangulation codes in the TABI Poisson-Boltzmann solver.J Comput Chem. 2021 Aug 15;42(22):1552-1560. doi: 10.1002/jcc.26692. Epub 2021 May 26. J Comput Chem. 2021. PMID: 34041777
-
Machine Learning of Coarse-Grained Models for Organic Molecules and Polymers: Progress, Opportunities, and Challenges.ACS Omega. 2021 Jan 11;6(3):1758-1772. doi: 10.1021/acsomega.0c05321. eCollection 2021 Jan 26. ACS Omega. 2021. PMID: 33521417 Free PMC article. Review.
-
Securing Machine Learning in the Cloud: A Systematic Review of Cloud Machine Learning Security.Front Big Data. 2020 Nov 12;3:587139. doi: 10.3389/fdata.2020.587139. eCollection 2020. Front Big Data. 2020. PMID: 33693420 Free PMC article. Review.
Cited by
-
A Concise Review of Biomolecule Visualization.Curr Issues Mol Biol. 2024 Feb 2;46(2):1318-1334. doi: 10.3390/cimb46020084. Curr Issues Mol Biol. 2024. PMID: 38392202 Free PMC article. Review.
-
AmberTools.J Chem Inf Model. 2023 Oct 23;63(20):6183-6191. doi: 10.1021/acs.jcim.3c01153. Epub 2023 Oct 8. J Chem Inf Model. 2023. PMID: 37805934 Free PMC article.
-
Improving the Accuracy of Physics-Based Hydration-Free Energy Predictions by Machine Learning the Remaining Error Relative to the Experiment.J Chem Theory Comput. 2024 Jan 9;20(1):396-410. doi: 10.1021/acs.jctc.3c00981. Epub 2023 Dec 27. J Chem Theory Comput. 2024. PMID: 38149593 Free PMC article.
-
Grid-Robust Efficient Neural Interface Model for Universal Molecule Surface Construction from Point Clouds.J Phys Chem Lett. 2023 Oct 12;14(40):9034-9041. doi: 10.1021/acs.jpclett.3c02176. Epub 2023 Oct 2. J Phys Chem Lett. 2023. PMID: 37782231 Free PMC article.
References
-
- Richards FM Areas, volumes, packing, and protein structure. Annu. Rev. Biophys. Bioeng 1977, 6, 151–176. - PubMed
-
- Connolly ML Solvent-accessible surfaces of proteins and nucleic acids. Science 1983, 221, 709–713. - PubMed
-
- Connolly ML Analytical molecular surface calculation. J. Appl. Crystallogr 1983, 16, 548–558.
-
- Gilson MK; Sharp KA; Honig BH Calculating the electrostatic potential of molecules in solution - method and error assessment. J. Comput. Chem 1988, 9, 327–335.
-
- Rocchia W; Sridharan S; Nicholls A; Alexov E; Chiabrera A; Honig B Rapid grid-based construction of the molecular surface and the use of induced surface charge to calculate reaction field energies: Applications to the molecular systems and geometric objects. J. Comput. Chem 2002, 23, 128–137. - PubMed