Metagenomic evidence for a polymicrobial signature of sepsis
- PMID: 34477543
- PMCID: PMC8715444
- DOI: 10.1099/mgen.0.000642
Metagenomic evidence for a polymicrobial signature of sepsis
Abstract
Our understanding of the host component of sepsis has made significant progress. However, detailed study of the microorganisms causing sepsis, either as single pathogens or microbial assemblages, has received far less attention. Metagenomic data offer opportunities to characterize the microbial communities found in septic and healthy individuals. In this study we apply gradient-boosted tree classifiers and a novel computational decontamination technique built upon SHapley Additive exPlanations (SHAP) to identify microbial hallmarks which discriminate blood metagenomic samples of septic patients from that of healthy individuals. Classifiers had high performance when using the read assignments to microbial genera [area under the receiver operating characteristic (AUROC=0.995)], including after removal of species 'culture-confirmed' as the cause of sepsis through clinical testing (AUROC=0.915). Models trained on single genera were inferior to those employing a polymicrobial model and we identified multiple co-occurring bacterial genera absent from healthy controls. While prevailing diagnostic paradigms seek to identify single pathogens, our results point to the involvement of a polymicrobial community in sepsis. We demonstrate the importance of the microbial component in characterising sepsis, which may offer new biological insights into the aetiology of sepsis, and ultimately support the development of clinical diagnostic or even prognostic tools.
Keywords: SHAP; bacteraemia; blood metagenomics; contamination; kitome; machine learning; metagenomics; sepsis.
Conflict of interest statement
The authors declare that there are no conflicts of interest.
Figures
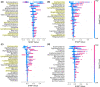
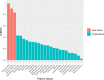
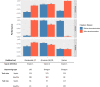
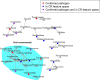
Similar articles
-
Molecular Methodologies for Improved Polymicrobial Sepsis Diagnosis.Int J Mol Sci. 2022 Apr 19;23(9):4484. doi: 10.3390/ijms23094484. Int J Mol Sci. 2022. PMID: 35562877 Free PMC article. Review.
-
The microbiological diagnostic performance of metagenomic next-generation sequencing in patients with sepsis.BMC Infect Dis. 2021 Dec 16;21(1):1257. doi: 10.1186/s12879-021-06934-7. BMC Infect Dis. 2021. PMID: 34915851 Free PMC article.
-
Massive metagenomic data analysis using abundance-based machine learning.Biol Direct. 2019 Aug 1;14(1):12. doi: 10.1186/s13062-019-0242-0. Biol Direct. 2019. PMID: 31370905 Free PMC article.
-
A bacterial sensor taxonomy across earth ecosystems for machine learning applications.mSystems. 2024 Jan 23;9(1):e0002623. doi: 10.1128/msystems.00026-23. Epub 2023 Dec 11. mSystems. 2024. PMID: 38078749 Free PMC article.
-
What Is Metagenomics Teaching Us, and What Is Missed?Annu Rev Microbiol. 2020 Sep 8;74:117-135. doi: 10.1146/annurev-micro-012520-072314. Epub 2020 Jun 30. Annu Rev Microbiol. 2020. PMID: 32603623 Review.
Cited by
-
No evidence for a common blood microbiome based on a population study of 9,770 healthy humans.Nat Microbiol. 2023 May;8(5):973-985. doi: 10.1038/s41564-023-01350-w. Epub 2023 Mar 30. Nat Microbiol. 2023. PMID: 36997797 Free PMC article.
-
No microbial community in the blood of healthy individuals.Nat Microbiol. 2023 May;8(5):769-770. doi: 10.1038/s41564-023-01364-4. Nat Microbiol. 2023. PMID: 37002417 No abstract available.
-
Polymicrobial Infections and Biofilms: Clinical Significance and Eradication Strategies.Antibiotics (Basel). 2022 Dec 1;11(12):1731. doi: 10.3390/antibiotics11121731. Antibiotics (Basel). 2022. PMID: 36551388 Free PMC article. Review.
-
Molecular Methodologies for Improved Polymicrobial Sepsis Diagnosis.Int J Mol Sci. 2022 Apr 19;23(9):4484. doi: 10.3390/ijms23094484. Int J Mol Sci. 2022. PMID: 35562877 Free PMC article. Review.
-
Divergent bacterial landscapes: unraveling geographically driven microbiomes in Atlantic cod.Sci Rep. 2024 Mar 13;14(1):6088. doi: 10.1038/s41598-024-56616-y. Sci Rep. 2024. PMID: 38480867 Free PMC article.
References
Publication types
MeSH terms
LinkOut - more resources
Full Text Sources
Medical