A graph neural network model to estimate cell-wise metabolic flux using single-cell RNA-seq data
- PMID: 34301623
- PMCID: PMC8494226
- DOI: 10.1101/gr.271205.120
A graph neural network model to estimate cell-wise metabolic flux using single-cell RNA-seq data
Abstract
The metabolic heterogeneity and metabolic interplay between cells are known as significant contributors to disease treatment resistance. However, with the lack of a mature high-throughput single-cell metabolomics technology, we are yet to establish systematic understanding of the intra-tissue metabolic heterogeneity and cooperative mechanisms. To mitigate this knowledge gap, we developed a novel computational method, namely, single-cell flux estimation analysis (scFEA), to infer the cell-wise fluxome from single-cell RNA-sequencing (scRNA-seq) data. scFEA is empowered by a systematically reconstructed human metabolic map as a factor graph, a novel probabilistic model to leverage the flux balance constraints on scRNA-seq data, and a novel graph neural network-based optimization solver. The intricate information cascade from transcriptome to metabolome was captured using multilayer neural networks to capitulate the nonlinear dependency between enzymatic gene expressions and reaction rates. We experimentally validated scFEA by generating an scRNA-seq data set with matched metabolomics data on cells of perturbed oxygen and genetic conditions. Application of scFEA on this data set showed the consistency between predicted flux and the observed variation of metabolite abundance in the matched metabolomics data. We also applied scFEA on five publicly available scRNA-seq and spatial transcriptomics data sets and identified context- and cell group-specific metabolic variations. The cell-wise fluxome predicted by scFEA empowers a series of downstream analyses including identification of metabolic modules or cell groups that share common metabolic variations, sensitivity evaluation of enzymes with regards to their impact on the whole metabolic flux, and inference of cell-tissue and cell-cell metabolic communications.
© 2021 Alghamdi et al.; Published by Cold Spring Harbor Laboratory Press.
Figures
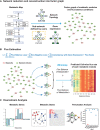
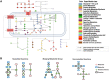
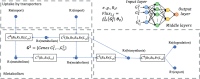
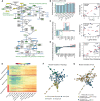
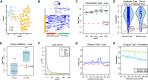
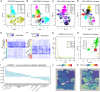
Similar articles
-
Combining denoising of RNA-seq data and flux balance analysis for cluster analysis of single cells.BMC Bioinformatics. 2022 Oct 25;23(Suppl 6):445. doi: 10.1186/s12859-022-04967-6. BMC Bioinformatics. 2022. PMID: 36284276 Free PMC article.
-
FLUXestimator: a webserver for predicting metabolic flux and variations using transcriptomics data.Nucleic Acids Res. 2023 Jul 5;51(W1):W180-W190. doi: 10.1093/nar/gkad444. Nucleic Acids Res. 2023. PMID: 37216602 Free PMC article.
-
Single-cell RNA-seq data analysis based on directed graph neural network.Methods. 2023 Mar;211:48-60. doi: 10.1016/j.ymeth.2023.02.008. Epub 2023 Feb 16. Methods. 2023. PMID: 36804214
-
Machine learning and statistical methods for clustering single-cell RNA-sequencing data.Brief Bioinform. 2020 Jul 15;21(4):1209-1223. doi: 10.1093/bib/bbz063. Brief Bioinform. 2020. PMID: 31243426 Review.
-
Mapping and Validation of scRNA-Seq-Derived Cell-Cell Communication Networks in the Tumor Microenvironment.Front Immunol. 2022 Apr 28;13:885267. doi: 10.3389/fimmu.2022.885267. eCollection 2022. Front Immunol. 2022. PMID: 35572582 Free PMC article. Review.
Cited by
-
Systems-based approaches to study immunometabolism.Cell Mol Immunol. 2022 Mar;19(3):409-420. doi: 10.1038/s41423-021-00783-9. Epub 2022 Feb 4. Cell Mol Immunol. 2022. PMID: 35121805 Free PMC article. Review.
-
Preleukemic single-cell landscapes reveal mutation-specific mechanisms and gene programs predictive of AML patient outcomes.Cell Genom. 2023 Oct 27;3(12):100426. doi: 10.1016/j.xgen.2023.100426. eCollection 2023 Dec 13. Cell Genom. 2023. PMID: 38116120 Free PMC article.
-
Metabolic rewiring and communication in cancer immunity.Cell Chem Biol. 2024 May 16;31(5):862-883. doi: 10.1016/j.chembiol.2024.02.001. Epub 2024 Feb 29. Cell Chem Biol. 2024. PMID: 38428418 Free PMC article. Review.
-
Single-cell and spatial analyses reveal the association between gene expression of glutamine synthetase with the immunosuppressive phenotype of APOE+CTSZ+TAM in cancers.Mol Oncol. 2023 Apr;17(4):611-628. doi: 10.1002/1878-0261.13373. Epub 2023 Jan 21. Mol Oncol. 2023. PMID: 36587392 Free PMC article.
-
Targeting neoadjuvant chemotherapy-induced metabolic reprogramming in pancreatic cancer promotes anti-tumor immunity and chemo-response.Cell Rep Med. 2023 Oct 17;4(10):101234. doi: 10.1016/j.xcrm.2023.101234. Cell Rep Med. 2023. PMID: 37852179 Free PMC article.
References
-
- Ali A, Abouleila Y, Shimizu Y, Hiyama E, Emara S, Mashaghi A, Hankemeier T. 2019. Single-cell metabolomics by mass spectrometry: advances, challenges, and future applications. Trends Analyt Chem 120: 115436. 10.1016/j.trac.2019.02.033 - DOI
Publication types
MeSH terms
Grants and funding
LinkOut - more resources
Full Text Sources
Miscellaneous