A universal framework for detecting cis-regulatory diversity in DNA regions
- PMID: 34285090
- PMCID: PMC8415372
- DOI: 10.1101/gr.274563.120
A universal framework for detecting cis-regulatory diversity in DNA regions
Abstract
High-throughput sequencing-based assays measure different biochemical activities pertaining to gene regulation, genome-wide. These activities include transcription factor (TF)-DNA binding, enhancer activity, open chromatin, and more. A major goal is to understand underlying sequence components, or motifs, that can explain the measured activity. It is usually not one motif but a combination of motifs bound by cooperatively acting proteins that confers activity to such regions. Furthermore, regions can be diverse, governed by different combinations of TFs/motifs. Current approaches do not take into account this issue of combinatorial diversity. We present a new statistical framework, cisDIVERSITY, which models regions as diverse modules characterized by combinations of motifs while simultaneously learning the motifs themselves. Because cisDIVERSITY does not rely on knowledge of motifs, modules, cell type, or organism, it is general enough to be applied to regions reported by most high-throughput assays. For example, in enhancer predictions resulting from different assays-GRO-cap, STARR-seq, and those measuring chromatin structure-cisDIVERSITY discovers distinct modules and combinations of TF binding sites, some specific to the assay. From protein-DNA binding data, cisDIVERSITY identifies potential cofactors of the profiled TF, whereas from ATAC-seq data, it identifies tissue-specific regulatory modules. Finally, analysis of single-cell ATAC-seq data suggests that regions open in one cell-state encode information about future states, with certain modules staying open and others closing down in the next time point.
© 2021 Biswas and Narlikar; Published by Cold Spring Harbor Laboratory Press.
Figures
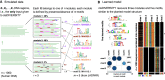
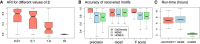
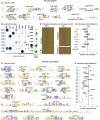
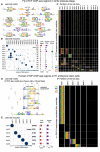
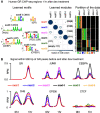
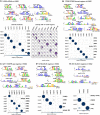
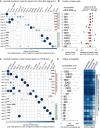
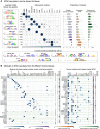
Similar articles
-
Information content differentiates enhancers from silencers in mouse photoreceptors.Elife. 2021 Sep 6;10:e67403. doi: 10.7554/eLife.67403. Elife. 2021. PMID: 34486522 Free PMC article.
-
Sequence and chromatin determinants of cell-type-specific transcription factor binding.Genome Res. 2012 Sep;22(9):1723-34. doi: 10.1101/gr.127712.111. Genome Res. 2012. PMID: 22955984 Free PMC article.
-
Sequence features and chromatin structure around the genomic regions bound by 119 human transcription factors.Genome Res. 2012 Sep;22(9):1798-812. doi: 10.1101/gr.139105.112. Genome Res. 2012. PMID: 22955990 Free PMC article.
-
Organizing combinatorial transcription factor recruitment at cis-regulatory modules.Transcription. 2018;9(4):233-239. doi: 10.1080/21541264.2017.1394424. Epub 2017 Nov 28. Transcription. 2018. PMID: 29105538 Free PMC article. Review.
-
DNA sequence motif: a jack of all trades for ChIP-Seq data.Adv Protein Chem Struct Biol. 2013;91:135-71. doi: 10.1016/B978-0-12-411637-5.00005-6. Adv Protein Chem Struct Biol. 2013. PMID: 23790213 Review.
Cited by
-
How to proceed after "negative" exome: A review on genetic diagnostics, limitations, challenges, and emerging new multiomics techniques.J Inherit Metab Dis. 2022 Jul;45(4):663-681. doi: 10.1002/jimd.12507. Epub 2022 May 22. J Inherit Metab Dis. 2022. PMID: 35506430 Free PMC article. Review.
-
Resolving diverse protein-DNA footprints from exonuclease-based ChIP experiments.Bioinformatics. 2021 Jul 12;37(Suppl_1):i367-i375. doi: 10.1093/bioinformatics/btab274. Bioinformatics. 2021. PMID: 34252930 Free PMC article.
References
-
- Bailey TL, Elkan C. 1994. Fitting a mixture model by expectation maximization to discover motifs in biopolymers. Proc Int Conf Intell Syst Mol Biol 2:28–36. - PubMed
Publication types
MeSH terms
Substances
LinkOut - more resources
Full Text Sources
Miscellaneous