Screening and identification of angiogenesis-related genes as potential novel prognostic biomarkers of hepatocellular carcinoma through bioinformatics analysis
- PMID: 34252885
- PMCID: PMC8312452
- DOI: 10.18632/aging.203260
Screening and identification of angiogenesis-related genes as potential novel prognostic biomarkers of hepatocellular carcinoma through bioinformatics analysis
Abstract
Hepatocellular carcinoma (HCC) is a malignant tumor with high morbidity and mortality, which makes the prognostic prediction challenging. Angiogenesis appears to be of critical importance in the progression and metastasis of HCC. Some of the angiogenesis-related genes promote this process, while other anti-angiogenesis genes suppress tumor growth and metastasis. Therefore, the comprehensive prognostic value of multiple angiogenesis-related genes in HCC needs to be further clarified. In this study, the mRNA expression profile of HCC patients and the corresponding clinical data were acquired from multiple public databases. Univariate Cox regression analysis was utilized to screen out differentially expressed angiogenesis-related genes with prognostic value. A multigene signature was established with the least absolute shrinkage and selection operator Cox regression in the Cancer Genome Atlas cohort, and validated through an independent cohort. The results suggested that a total of 16 differentially expressed genes (DEGs) were associated with overall survival (OS) and a 7-gene signature was constructed. The risk score of each patient was calculated using this signature, the median value of which was used to divide these patients into a high-risk group and a low-risk group. Compared with the low-risk group, the patients in the high-risk group had a poor prognosis. The risk score was an independent predictor for OS through multivariate Cox regression analysis. Then, unsupervised learning was used to verify the validity of this 7-gene signature. A nomogram by further integrating clinical information and the prognostic signature was utilized to predict prognostic risk and individual OS. Functional enrichment analyses demonstrated that these DEGs were enriched in the pathways of cell proliferation and mitosis, and the immune cell infiltration was significantly different between the two risk groups. In summary, a novel angiogenesis-related genes signature could be used to predict the prognosis of HCC and for targeted therapy.
Keywords: angiogenesis; bioinformatics analysis; gene signature; hepatocellular carcinoma; prognosis.
Conflict of interest statement
Figures
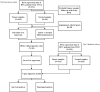
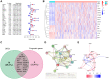
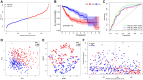
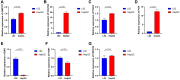
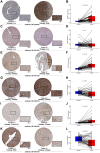
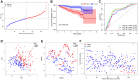
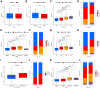
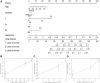
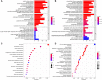
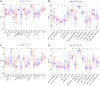
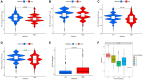
Similar articles
-
Construction and Validation of a Prognostic Gene-Based Model for Overall Survival Prediction in Hepatocellular Carcinoma Using an Integrated Statistical and Bioinformatic Approach.Int J Mol Sci. 2021 Feb 5;22(4):1632. doi: 10.3390/ijms22041632. Int J Mol Sci. 2021. PMID: 33562824 Free PMC article.
-
The pyroptosis-related gene signature predicts prognosis and indicates immune activity in hepatocellular carcinoma.Mol Med. 2022 Feb 5;28(1):16. doi: 10.1186/s10020-022-00445-0. Mol Med. 2022. PMID: 35123387 Free PMC article.
-
Comprehensive analyses of competing endogenous RNA networks reveal potential biomarkers for predicting hepatocellular carcinoma recurrence.BMC Cancer. 2021 Apr 20;21(1):436. doi: 10.1186/s12885-021-08173-0. BMC Cancer. 2021. PMID: 33879119 Free PMC article.
-
Mining Prognostic Biomarkers of Hepatocellular Carcinoma Based on Immune-Associated Genes.DNA Cell Biol. 2020 Apr;39(4):499-512. doi: 10.1089/dna.2019.5099. Epub 2020 Feb 18. DNA Cell Biol. 2020. PMID: 32069130 Review.
-
The prognostic molecular markers in hepatocellular carcinoma.World J Gastroenterol. 2002 Jun;8(3):385-92. doi: 10.3748/wjg.v8.i3.385. World J Gastroenterol. 2002. PMID: 12046056 Free PMC article. Review.
Cited by
-
Dissecting a hypoxia-related angiogenic gene signature for predicting prognosis and immune status in hepatocellular carcinoma.Front Oncol. 2022 Aug 30;12:978050. doi: 10.3389/fonc.2022.978050. eCollection 2022. Front Oncol. 2022. PMID: 36110938 Free PMC article.
-
Prognostic Hypoxia-Angiogenesis-Related Gene Signature in Hepatocellular Carcinoma, in Which HILPDA Contributes to Tumor Progression.J Inflamm Res. 2024 Aug 27;17:5663-5683. doi: 10.2147/JIR.S476388. eCollection 2024. J Inflamm Res. 2024. PMID: 39219818 Free PMC article.
-
Downregulation of Low-density lipoprotein receptor-related protein 1B (LRP1B) inhibits the progression of hepatocellular carcinoma cells by activating the endoplasmic reticulum stress signaling pathway.Bioengineered. 2022 Apr;13(4):9467-9481. doi: 10.1080/21655979.2022.2060778. Bioengineered. 2022. PMID: 35389768 Free PMC article.
-
Identification of VEGFs-related gene signature for predicting microangiogenesis and hepatocellular carcinoma prognosis.Aging (Albany NY). 2024 Jun 13;16(12):10321-10347. doi: 10.18632/aging.205931. Epub 2024 Jun 13. Aging (Albany NY). 2024. PMID: 38874512 Free PMC article.
-
Pyroptosis-Related Risk Signature Exhibits Distinct Prognostic, Immune, and Therapeutic Landscapes in Hepatocellular Carcinoma.Front Genet. 2022 Mar 9;13:823443. doi: 10.3389/fgene.2022.823443. eCollection 2022. Front Genet. 2022. PMID: 35368686 Free PMC article.
References
-
- Torrecilla S, Sia D, Harrington AN, Zhang Z, Cabellos L, Cornella H, Moeini A, Camprecios G, Leow WQ, Fiel MI, Hao K, Bassaganyas L, Mahajan M, et al.. Trunk mutational events present minimal intra- and inter-tumoral heterogeneity in hepatocellular carcinoma. J Hepatol. 2017; 67:1222–31. 10.1016/j.jhep.2017.08.013 - DOI - PubMed
Publication types
MeSH terms
Substances
LinkOut - more resources
Full Text Sources
Medical