Homology Characteristics of EEG and EMG for Lower Limb Voluntary Movement Intention
- PMID: 34220479
- PMCID: PMC8249921
- DOI: 10.3389/fnbot.2021.642607
Homology Characteristics of EEG and EMG for Lower Limb Voluntary Movement Intention
Abstract
In the field of lower limb exoskeletons, besides its electromechanical system design and control, attention has been paid to realizing the linkage of exoskeleton robots to humans via electroencephalography (EEG) and electromyography (EMG). However, even the state of the art performance of lower limb voluntary movement intention decoding still faces many obstacles. In the following work, focusing on the perspective of the inner mechanism, a homology characteristic of EEG and EMG for lower limb voluntary movement intention was conducted. A mathematical model of EEG and EMG was built based on its mechanism, which consists of a neural mass model (NMM), neuromuscular junction model, EMG generation model, decoding model, and musculoskeletal biomechanical model. The mechanism analysis and simulation results demonstrated that EEG and EMG signals were both excited by the same movement intention with a response time difference. To assess the efficiency of the proposed model, a synchronous acquisition system for EEG and EMG was constructed to analyze the homology and response time difference from EEG and EMG signals in the limb movement intention. An effective method of wavelet coherence was used to analyze the internal correlation between EEG and EMG signals in the same limb movement intention. To further prove the effectiveness of the hypothesis in this paper, six subjects were involved in the experiments. The experimental results demonstrated that there was a strong EEG-EMG coherence at 1 Hz around movement onset, and the phase of EEG was leading the EMG. Both the simulation and experimental results revealed that EEG and EMG are homologous, and the response time of the EEG signals are earlier than EMG signals during the limb movement intention. This work can provide a theoretical basis for the feasibility of EEG-based pre-perception and fusion perception of EEG and EMG in human movement detection.
Keywords: EEG; EMG; coherence; homology analysis; numerical simulation.
Copyright © 2021 Zhang, Li, Lu and Yin.
Conflict of interest statement
The authors declare that the research was conducted in the absence of any commercial or financial relationships that could be construed as a potential conflict of interest.
Figures
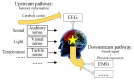
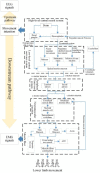
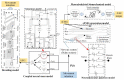
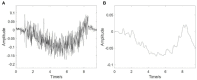
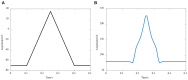
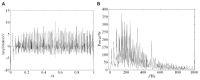
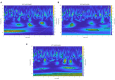
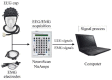
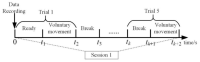
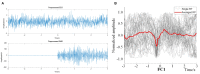
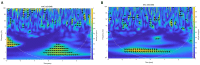
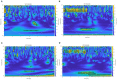
Similar articles
-
Electroencephalogram and surface electromyogram fusion-based precise detection of lower limb voluntary movement using convolution neural network-long short-term memory model.Front Neurosci. 2022 Sep 23;16:954387. doi: 10.3389/fnins.2022.954387. eCollection 2022. Front Neurosci. 2022. PMID: 36213740 Free PMC article.
-
Cross-domain prediction approach of human lower limb voluntary movement intention for exoskeleton robot based on EEG signals.Front Bioeng Biotechnol. 2024 Aug 23;12:1448903. doi: 10.3389/fbioe.2024.1448903. eCollection 2024. Front Bioeng Biotechnol. 2024. PMID: 39246298 Free PMC article.
-
Hierarchical Decoding Model of Upper Limb Movement Intention From EEG Signals Based on Attention State Estimation.IEEE Trans Neural Syst Rehabil Eng. 2021;29:2008-2016. doi: 10.1109/TNSRE.2021.3115490. Epub 2021 Oct 1. IEEE Trans Neural Syst Rehabil Eng. 2021. PMID: 34559657
-
Combined Use of EMG and EEG Techniques for Neuromotor Assessment in Rehabilitative Applications: A Systematic Review.Sensors (Basel). 2021 Oct 22;21(21):7014. doi: 10.3390/s21217014. Sensors (Basel). 2021. PMID: 34770320 Free PMC article. Review.
-
Measuring human locomotor control using EMG and EEG: Current knowledge, limitations and future considerations.Eur J Sport Sci. 2016;16(4):416-26. doi: 10.1080/17461391.2015.1068869. Epub 2015 Aug 4. Eur J Sport Sci. 2016. PMID: 26238032 Review.
Cited by
-
Estimation of Knee Joint Angle from Surface EMG Using Multiple Kernels Relevance Vector Regression.Sensors (Basel). 2023 May 20;23(10):4934. doi: 10.3390/s23104934. Sensors (Basel). 2023. PMID: 37430848 Free PMC article.
-
Electroencephalogram and surface electromyogram fusion-based precise detection of lower limb voluntary movement using convolution neural network-long short-term memory model.Front Neurosci. 2022 Sep 23;16:954387. doi: 10.3389/fnins.2022.954387. eCollection 2022. Front Neurosci. 2022. PMID: 36213740 Free PMC article.
-
Realizing the Application of EEG Modeling in BCI Classification: Based on a Conditional GAN Converter.Front Neurosci. 2021 Nov 11;15:727394. doi: 10.3389/fnins.2021.727394. eCollection 2021. Front Neurosci. 2021. PMID: 34867150 Free PMC article.
-
EEG generation mechanism of lower limb active movement intention and its virtual reality induction enhancement: a preliminary study.Front Neurosci. 2024 Jan 30;17:1305850. doi: 10.3389/fnins.2023.1305850. eCollection 2023. Front Neurosci. 2024. PMID: 38352938 Free PMC article.
-
Review of adaptive control for stroke lower limb exoskeleton rehabilitation robot based on motion intention recognition.Front Neurorobot. 2023 Jul 3;17:1186175. doi: 10.3389/fnbot.2023.1186175. eCollection 2023. Front Neurorobot. 2023. PMID: 37465413 Free PMC article. Review.
References
-
- Guan B., Zhang H., Li Z. (2013). Basic Principles of Cellular Electrophysiology and Patch Clamp Techniques. Beijing: Science Press.
-
- Hanzhe L., Xiaodong Z., Rui L., Zhufeng L., Kuncai Y., Gui Y. (2019a). A preperception method for voluntary movement intention of lower limb using readiness potential. J. Xi'an Jiaotong Univer. 53, 16–23. 10.7652/xjtuxb201910003 - DOI
-
- Hanzhe L., Xiaodong Z., Zhufeng L., Rui L. (2019b). “Detection of Readiness Potential for Lower Limb Voluntary Movement Based on EMG Onset”, in The 9th IEEE International Conference on CYBER Technology in Automation, Control, and Intelligent Systems (IEEE-CYBER 2019) (Suzhou).
LinkOut - more resources
Full Text Sources