A Methodological Framework to Discover Pharmacogenomic Interactions Based on Random Forests
- PMID: 34207374
- PMCID: PMC8235396
- DOI: 10.3390/genes12060933
A Methodological Framework to Discover Pharmacogenomic Interactions Based on Random Forests
Abstract
The identification of genomic alterations in tumor tissues, including somatic mutations, deletions, and gene amplifications, produces large amounts of data, which can be correlated with a diversity of therapeutic responses. We aimed to provide a methodological framework to discover pharmacogenomic interactions based on Random Forests. We matched two databases from the Cancer Cell Line Encyclopaedia (CCLE) project, and the Genomics of Drug Sensitivity in Cancer (GDSC) project. For a total of 648 shared cell lines, we considered 48,270 gene alterations from CCLE as input features and the area under the dose-response curve (AUC) for 265 drugs from GDSC as the outcomes. A three-step reduction to 501 alterations was performed, selecting known driver genes and excluding very frequent/infrequent alterations and redundant ones. For each model, we used the concordance correlation coefficient (CCC) for assessing the predictive performance, and permutation importance for assessing the contribution of each alteration. In a reasonable computational time (56 min), we identified 12 compounds whose response was at least fairly sensitive (CCC > 20) to the alteration profiles. Some diversities were found in the sets of influential alterations, providing clues to discover significant drug-gene interactions. The proposed methodological framework can be helpful for mining pharmacogenomic interactions.
Keywords: Random Forests; cancer; cell lines; drug response; genomic alterations; pharmacogenomic interactions.
Conflict of interest statement
The authors declare no conflict of interest.
Figures
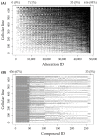
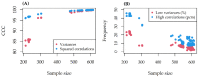
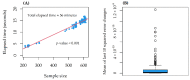
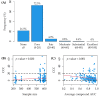
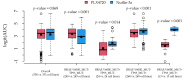
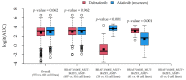
Similar articles
-
Improved anticancer drug response prediction in cell lines using matrix factorization with similarity regularization.BMC Cancer. 2017 Aug 2;17(1):513. doi: 10.1186/s12885-017-3500-5. BMC Cancer. 2017. PMID: 28768489 Free PMC article.
-
Encircling the regions of the pharmacogenomic landscape that determine drug response.Genome Med. 2019 Mar 26;11(1):17. doi: 10.1186/s13073-019-0626-x. Genome Med. 2019. PMID: 30914058 Free PMC article.
-
Integrating heterogeneous drug sensitivity data from cancer pharmacogenomic studies.Oncotarget. 2016 Aug 9;7(32):51619-51625. doi: 10.18632/oncotarget.10010. Oncotarget. 2016. PMID: 27322211 Free PMC article.
-
Evaluating the consistency of large-scale pharmacogenomic studies.Brief Bioinform. 2019 Sep 27;20(5):1734-1753. doi: 10.1093/bib/bby046. Brief Bioinform. 2019. PMID: 31846027 Free PMC article. Review.
-
Predictive genomics: a cancer hallmark network framework for predicting tumor clinical phenotypes using genome sequencing data.Semin Cancer Biol. 2015 Feb;30:4-12. doi: 10.1016/j.semcancer.2014.04.002. Epub 2014 Apr 18. Semin Cancer Biol. 2015. PMID: 24747696 Review.
Cited by
-
Interpretable Machine Learning Models to Predict the Resistance of Breast Cancer Patients to Doxorubicin from Their microRNA Profiles.Adv Sci (Weinh). 2022 Aug;9(24):e2201501. doi: 10.1002/advs.202201501. Epub 2022 Jul 3. Adv Sci (Weinh). 2022. PMID: 35785523 Free PMC article.
-
Pharmacogenomics: Challenges and Future.Genes (Basel). 2024 May 30;15(6):714. doi: 10.3390/genes15060714. Genes (Basel). 2024. PMID: 38927650 Free PMC article.
References
-
- Barretina J., Caponigro G., Stransky N., Venkatesan K., Margolin A.A., Kim S., Wilson C.J., Lehár J., Kryukov G.V., Sonkin D., et al. The Cancer Cell Line Encyclopedia Enables Predictive Modelling of Anticancer Drug Sensitivity. Nature. 2012;483:603–607. doi: 10.1038/nature11003. - DOI - PMC - PubMed
-
- Yang W., Soares J., Greninger P., Edelman E.J., Lightfoot H., Forbes S., Bindal N., Beare D., Smith J.A., Thompson I.R., et al. Genomics of Drug Sensitivity in Cancer (GDSC): A Resource for Therapeutic Biomarker Discovery in Cancer Cells. Nucleic Acids Res. 2013;41:D955–D961. doi: 10.1093/nar/gks1111. - DOI - PMC - PubMed
MeSH terms
LinkOut - more resources
Full Text Sources