Coronavirus disease (COVID-19) detection using X-ray images and enhanced DenseNet
- PMID: 34191925
- PMCID: PMC8225990
- DOI: 10.1016/j.asoc.2021.107645
Coronavirus disease (COVID-19) detection using X-ray images and enhanced DenseNet
Abstract
The 2019 novel coronavirus (COVID-19) originating from China, has spread rapidly among people living in other countries. According to the World Health Organization (WHO), by the end of January, more than 104 million people have been affected by COVID-19, including more than 2 million deaths. The number of COVID-19 test kits available in hospitals is reduced due to the increase in regular cases. Therefore, an automatic detection system should be introduced as a fast, alternative diagnostic to prevent COVID-19 from spreading among humans. For this purpose, three different BiT models: DenseNet, InceptionV3, and Inception-ResNetV4 have been proposed in this analysis for the diagnosis of patients infected with coronavirus pneumonia using X-ray radiographs in the chest. These three models give and examine Receiver Operating Characteristic (ROC) analyses and uncertainty matrices, using 5-fold cross-validation. We have performed the simulations which have visualized that the pre-trained DenseNet model has the best classification efficiency with 92% among two other models proposed (83.47% accuracy for inception V3 and 85.57% accuracy for Inception-ResNetV4).
Keywords: Biomedical imaging; COVID-19; Convolutional neural network; Deep learning; DenseNet; ResNet.
© 2021 Elsevier B.V. All rights reserved.
Conflict of interest statement
The authors declare that they have no known competing financial interests or personal relationships that could have appeared to influence the work reported in this paper.
Figures
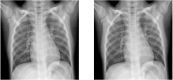
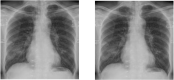
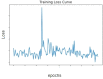
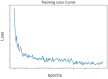
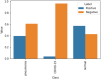
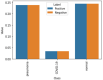
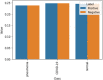
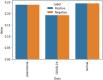
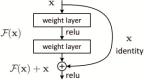
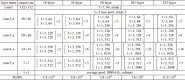
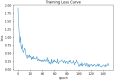
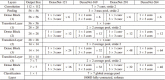
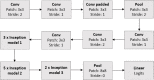
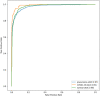
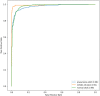
Similar articles
-
Diagnosis of Coronavirus Disease From Chest X-Ray Images Using DenseNet-169 Architecture.SN Comput Sci. 2023;4(3):214. doi: 10.1007/s42979-022-01627-7. Epub 2023 Feb 17. SN Comput Sci. 2023. PMID: 36811126 Free PMC article. Review.
-
Automatic detection of coronavirus disease (COVID-19) using X-ray images and deep convolutional neural networks.Pattern Anal Appl. 2021;24(3):1207-1220. doi: 10.1007/s10044-021-00984-y. Epub 2021 May 9. Pattern Anal Appl. 2021. PMID: 33994847 Free PMC article.
-
An automatic approach based on CNN architecture to detect Covid-19 disease from chest X-ray images.Appl Intell (Dordr). 2021;51(5):2864-2889. doi: 10.1007/s10489-020-02010-w. Epub 2020 Nov 27. Appl Intell (Dordr). 2021. PMID: 34764572 Free PMC article.
-
A radiomics-boosted deep-learning model for COVID-19 and non-COVID-19 pneumonia classification using chest x-ray images.Med Phys. 2022 May;49(5):3213-3222. doi: 10.1002/mp.15582. Epub 2022 Mar 15. Med Phys. 2022. PMID: 35263458 Free PMC article.
-
ADOPT: automatic deep learning and optimization-based approach for detection of novel coronavirus COVID-19 disease using X-ray images.J Biomol Struct Dyn. 2022 Aug;40(13):5836-5847. doi: 10.1080/07391102.2021.1875049. Epub 2021 Jan 21. J Biomol Struct Dyn. 2022. PMID: 33475019 Free PMC article.
Cited by
-
Exploring the Deep-Learning Techniques in Detecting the Presence of Coronavirus in the Chest X-Ray Images: A Comprehensive Review.Arch Comput Methods Eng. 2022;29(7):5381-5395. doi: 10.1007/s11831-022-09768-x. Epub 2022 May 23. Arch Comput Methods Eng. 2022. PMID: 35645554 Free PMC article. Review.
-
A high-accuracy lightweight network model for X-ray image diagnosis: A case study of COVID detection.PLoS One. 2024 Jun 18;19(6):e0303049. doi: 10.1371/journal.pone.0303049. eCollection 2024. PLoS One. 2024. PMID: 38889106 Free PMC article.
-
Densely attention mechanism based network for COVID-19 detection in chest X-rays.Sci Rep. 2023 Jan 6;13(1):261. doi: 10.1038/s41598-022-27266-9. Sci Rep. 2023. PMID: 36609667 Free PMC article.
-
Diagnosis of Coronavirus Disease From Chest X-Ray Images Using DenseNet-169 Architecture.SN Comput Sci. 2023;4(3):214. doi: 10.1007/s42979-022-01627-7. Epub 2023 Feb 17. SN Comput Sci. 2023. PMID: 36811126 Free PMC article. Review.
-
A fuzzy fine-tuned model for COVID-19 diagnosis.Comput Biol Med. 2023 Feb;153:106483. doi: 10.1016/j.compbiomed.2022.106483. Epub 2023 Jan 4. Comput Biol Med. 2023. PMID: 36621192 Free PMC article.
References
-
- Yan L., Zhang H.T., Xiao Y., Wang M., Guo Y., Sun C., Tang X., Jing L., Li S., Zhang M., Xiao Y., Cao H., Chen Y., Ren T., Jin J., Wang F., Xiao Y., Huang S., Tan X., Huang N., Jiao B., Zhang Y., Luo A., Cao Z., Xu H., Yuan Y. 2020. Prediction of criticality in patients with severe Covid-19 infection using three clinical features: a machine learning-based prognostic model with clinical data in wuhan. medRxiv 2020.02.27.20028027.
-
- Stoecklin S.B., Rolland P., Silue Y., Mailles A., Campese C., Simondon A., Mechain M., Meurice L., Nguyen M., Bassi C., Yamani E., Behillil S., Ismael S., Nguyen D., Malvy D., Lescure F.X., Georges S., Lazarus C., Tabäı A., Stempfelet M., Enouf V., Coignard B., Levy-Bruhl D., and Team, I. First cases of coron- avirus disease 2019 (COVID-19) in France: surveillance, investiga- tions and control measures, 2020. Eurosurveillance. 2020;25(6) - PMC - PubMed
-
- Huang C., Wang Y., Li X., Ren L., Zhao J., Hu Y., Zhang L., Fan G., Xu J., Gu X., Cheng Z., Yu T., Xia J., Wei Y., Wu W., Xie X., Yin W., Li H., Liu M., Xiao Y., Gao H., Guo L., Xie J., Wang G., Jiang R., Gao Z., Jin Q., Wang J., Cao B. Clinical features of patients infected with 2019 novel coronavirus in Wuhan, China. Lancet. 2020;395(10223):497–506. - PMC - PubMed
LinkOut - more resources
Full Text Sources