Plasma from patients with bacterial sepsis or severe COVID-19 induces suppressive myeloid cell production from hematopoietic progenitors in vitro
- PMID: 34103408
- PMCID: PMC8432955
- DOI: 10.1126/scitranslmed.abe9599
Plasma from patients with bacterial sepsis or severe COVID-19 induces suppressive myeloid cell production from hematopoietic progenitors in vitro
Abstract
Bacterial sepsis and severe COVID-19 share similar clinical manifestations and are both associated with dysregulation of the myeloid cell compartment. We previously reported an expanded CD14+ monocyte state, MS1, in patients with bacterial sepsis and validated expansion of this cell subpopulation in publicly available transcriptomics data. Here, using published datasets, we show that the gene expression program associated with MS1 correlated with sepsis severity and was up-regulated in monocytes from patients with severe COVID-19. To examine the ontogeny and function of MS1 cells, we developed a cellular model for inducing CD14+ MS1 monocytes from healthy bone marrow hematopoietic stem and progenitor cells (HSPCs). We found that plasma from patients with bacterial sepsis or COVID-19 induced myelopoiesis in HSPCs in vitro and expression of the MS1 gene program in monocytes and neutrophils that differentiated from these HSPCs. Furthermore, we found that plasma concentrations of IL-6, and to a lesser extent IL-10, correlated with increased myeloid cell output from HSPCs in vitro and enhanced expression of the MS1 gene program. We validated the requirement for these two cytokines to induce the MS1 gene program through CRISPR-Cas9 editing of their receptors in HSPCs. Using this cellular model system, we demonstrated that induced MS1 cells were broadly immunosuppressive and showed decreased responsiveness to stimulation with a synthetic RNA analog. Our in vitro study suggests a potential role for systemic cytokines in inducing myelopoiesis during severe bacterial or SARS-CoV-2 infection.
Copyright © 2021 The Authors, some rights reserved; exclusive licensee American Association for the Advancement of Science. No claim to original U.S. Government Works. Distributed under a Creative Commons Attribution License 4.0 (CC BY).
Figures
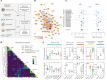
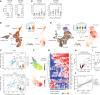

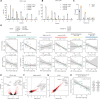
Update of
-
Induction of a regulatory myeloid program in bacterial sepsis and severe COVID-19.bioRxiv [Preprint]. 2020 Sep 2:2020.09.02.280180. doi: 10.1101/2020.09.02.280180. bioRxiv. 2020. Update in: Sci Transl Med. 2021 Jun 16;13(598):eabe9599. doi: 10.1126/scitranslmed.abe9599 PMID: 32908980 Free PMC article. Updated. Preprint.
Similar articles
-
Induction of a regulatory myeloid program in bacterial sepsis and severe COVID-19.bioRxiv [Preprint]. 2020 Sep 2:2020.09.02.280180. doi: 10.1101/2020.09.02.280180. bioRxiv. 2020. Update in: Sci Transl Med. 2021 Jun 16;13(598):eabe9599. doi: 10.1126/scitranslmed.abe9599 PMID: 32908980 Free PMC article. Updated. Preprint.
-
Monocyte state 1 (MS1) cells in critically ill patients with sepsis or non-infectious conditions: association with disease course and host response.Crit Care. 2024 Mar 19;28(1):88. doi: 10.1186/s13054-024-04868-5. Crit Care. 2024. PMID: 38504349 Free PMC article.
-
Hematopoietic stem and progenitor cells improve survival from sepsis by boosting immunomodulatory cells.Elife. 2022 Feb 15;11:e74561. doi: 10.7554/eLife.74561. Elife. 2022. PMID: 35166205 Free PMC article.
-
Regulation of myelopoiesis by proinflammatory cytokines in infectious diseases.Cell Mol Life Sci. 2018 Apr;75(8):1363-1376. doi: 10.1007/s00018-017-2724-5. Epub 2017 Dec 7. Cell Mol Life Sci. 2018. PMID: 29218601 Free PMC article. Review.
-
The Hematopoietic Stem/Progenitor Cell Response to Hemorrhage, Injury, and Sepsis: A Review of Pathophysiology.Shock. 2021 Jul 1;56(1):30-41. doi: 10.1097/SHK.0000000000001699. Shock. 2021. PMID: 33234838 Free PMC article. Review.
Cited by
-
Epigenetic and transcriptomic reprogramming in monocytes of severe COVID-19 patients reflects alterations in myeloid differentiation and the influence of inflammatory cytokines.Genome Med. 2022 Nov 29;14(1):134. doi: 10.1186/s13073-022-01137-4. Genome Med. 2022. PMID: 36443794 Free PMC article.
-
Altered and allele-specific open chromatin landscape reveals epigenetic and genetic regulators of innate immunity in COVID-19.Cell Genom. 2023 Feb 8;3(2):100232. doi: 10.1016/j.xgen.2022.100232. Epub 2022 Dec 2. Cell Genom. 2023. PMID: 36474914 Free PMC article.
-
Neutrophil Profiles of Pediatric COVID-19 and Multisystem Inflammatory Syndrome in Children.bioRxiv [Preprint]. 2021 Dec 20:2021.12.18.473308. doi: 10.1101/2021.12.18.473308. bioRxiv. 2021. Update in: Cell Rep Med. 2022 Dec 20;3(12):100848. doi: 10.1016/j.xcrm.2022.100848 PMID: 34981052 Free PMC article. Updated. Preprint.
-
Respiratory and systemic monocytes, dendritic cells, and myeloid-derived suppressor cells in COVID-19: Implications for disease severity.J Intern Med. 2023 Feb;293(2):130-143. doi: 10.1111/joim.13559. Epub 2022 Aug 31. J Intern Med. 2023. PMID: 35996885 Free PMC article. Review.
-
Long-term effects of Omicron BA.2 breakthrough infection on immunity-metabolism balance: a 6-month prospective study.Nat Commun. 2024 Mar 19;15(1):2444. doi: 10.1038/s41467-024-46692-z. Nat Commun. 2024. PMID: 38503738 Free PMC article.
References
-
- Rudd K. E., Johnson S. C., Agesa K. M., Shackelford K. A., Tsoi D., Kievlan D. R., Colombara D. V., Ikuta K. S., Kissoon N., Finfer S., Fleischmann-Struzek C., Machado F. R., Reinhart K. K., Rowan K., Seymour C. W., Watson R. S., West T. E., Marinho F., Hay S. I., Lozano R., Lopez A. D., Angus D. C., Murray C. J. L., Naghavi M., Global, regional, and national sepsis incidence and mortality, 1990-2017: Analysis for the Global Burden of Disease Study. Lancet 395, 200–211 (2020). - PMC - PubMed
-
- Filbin M. R., Lynch J., Gillingham T. D., Thorsen J. E., Pasakarnis C. L., Nepal S., Matsushima M., Rhee C., Heldt T., Reisner A. T., Presenting symptoms independently predict mortality in septic shock: Importance of a previously unmeasured confounder. Crit. Care Med. 46, 1592–1599 (2018). - PubMed
-
- van der Poll T., van de Veerdonk F. L., Scicluna B. P., Netea M. G., The immunopathology of sepsis and potential therapeutic targets. Nat. Rev. Immunol. 17, 407–420 (2017). - PubMed
-
- Alhazzani W., Møller M. H., Arabi Y. M., Loeb M., Gong M. N., Fan E., Oczkowski S., Levy M. M., Derde L., Dzierba A., Du B., Aboodi M., Wunsch H., Cecconi M., Koh Y., Chertow D. S., Maitland K., Alshamsi F., Belley-Cote E., Greco M., Laundy M., Morgan J. S., Kesecioglu J., McGeer A., Mermel L., Mammen M. J., Alexander P. E., Arrington A., Centofanti J. E., Citerio G., Baw B., Memish Z. A., Hammond N., Hayden F. G., Evans L., Rhodes A., Surviving sepsis campaign: Guidelines on the management of critically ill adults with coronavirus disease 2019 (COVID-19). Crit. Care Med. 48, e440–e469 (2020). - PMC - PubMed
Publication types
MeSH terms
Grants and funding
LinkOut - more resources
Full Text Sources
Medical
Research Materials
Miscellaneous