Development and validation of artificial intelligence to detect and diagnose liver lesions from ultrasound images
- PMID: 34101764
- PMCID: PMC8186767
- DOI: 10.1371/journal.pone.0252882
Development and validation of artificial intelligence to detect and diagnose liver lesions from ultrasound images
Abstract
Artificial intelligence (AI) using a convolutional neural network (CNN) has demonstrated promising performance in radiological analysis. We aimed to develop and validate a CNN for the detection and diagnosis of focal liver lesions (FLLs) from ultrasonography (USG) still images. The CNN was developed with a supervised training method using 40,397 retrospectively collected images from 3,487 patients, including 20,432 FLLs (hepatocellular carcinomas (HCCs), cysts, hemangiomas, focal fatty sparing, and focal fatty infiltration). AI performance was evaluated using an internal test set of 6,191 images with 845 FLLs, then externally validated using 18,922 images with 1,195 FLLs from two additional hospitals. The internal evaluation yielded an overall detection rate, diagnostic sensitivity and specificity of 87.0% (95%CI: 84.3-89.6), 83.9% (95%CI: 80.3-87.4), and 97.1% (95%CI: 96.5-97.7), respectively. The CNN also performed consistently well on external validation cohorts, with a detection rate, diagnostic sensitivity and specificity of 75.0% (95%CI: 71.7-78.3), 84.9% (95%CI: 81.6-88.2), and 97.1% (95%CI: 96.5-97.6), respectively. For diagnosis of HCC, the CNN yielded sensitivity, specificity, and negative predictive value (NPV) of 73.6% (95%CI: 64.3-82.8), 97.8% (95%CI: 96.7-98.9), and 96.5% (95%CI: 95.0-97.9) on the internal test set; and 81.5% (95%CI: 74.2-88.8), 94.4% (95%CI: 92.8-96.0), and 97.4% (95%CI: 96.2-98.5) on the external validation set, respectively. CNN detected and diagnosed common FLLs in USG images with excellent specificity and NPV for HCC. Further development of an AI system for real-time detection and characterization of FLLs in USG is warranted.
Conflict of interest statement
The authors have declared that no competing interests exist.
Figures
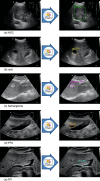
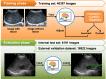
Similar articles
-
Artificial intelligence for ultrasonographic detection and diagnosis of hepatocellular carcinoma and cholangiocarcinoma.Sci Rep. 2024 Sep 4;14(1):20617. doi: 10.1038/s41598-024-71657-z. Sci Rep. 2024. PMID: 39232086 Free PMC article.
-
Application of artificial intelligence using a novel EUS-based convolutional neural network model to identify and distinguish benign and malignant hepatic masses.Gastrointest Endosc. 2021 May;93(5):1121-1130.e1. doi: 10.1016/j.gie.2020.08.024. Epub 2020 Aug 28. Gastrointest Endosc. 2021. PMID: 32861752
-
Computer-aided diagnosis for the classification of focal liver lesions by use of contrast-enhanced ultrasonography.Med Phys. 2008 May;35(5):1734-46. doi: 10.1118/1.2900109. Med Phys. 2008. PMID: 18561648 Free PMC article.
-
Convolutional neural network-based artificial intelligence for the diagnosis of early esophageal cancer based on endoscopic images: A meta-analysis.Saudi J Gastroenterol. 2022 Sep-Oct;28(5):332-340. doi: 10.4103/sjg.sjg_178_22. Saudi J Gastroenterol. 2022. PMID: 35848703 Free PMC article. Review.
-
Contrast-enhanced ultrasound using SonoVue® (sulphur hexafluoride microbubbles) compared with contrast-enhanced computed tomography and contrast-enhanced magnetic resonance imaging for the characterisation of focal liver lesions and detection of liver metastases: a systematic review and cost-effectiveness analysis.Health Technol Assess. 2013 Apr;17(16):1-243. doi: 10.3310/hta17160. Health Technol Assess. 2013. PMID: 23611316 Free PMC article. Review.
Cited by
-
Ultrasound-based artificial intelligence in gastroenterology and hepatology.World J Gastroenterol. 2022 Oct 14;28(38):5530-5546. doi: 10.3748/wjg.v28.i38.5530. World J Gastroenterol. 2022. PMID: 36304086 Free PMC article. Review.
-
Artificial intelligence techniques in liver cancer.Front Oncol. 2024 Sep 3;14:1415859. doi: 10.3389/fonc.2024.1415859. eCollection 2024. Front Oncol. 2024. PMID: 39290245 Free PMC article. Review.
-
Radiological Diagnosis of Chronic Liver Disease and Hepatocellular Carcinoma: A Review.J Med Syst. 2023 Jul 11;47(1):73. doi: 10.1007/s10916-023-01968-7. J Med Syst. 2023. PMID: 37432493 Free PMC article. Review.
-
Research Progress in Predicting Hepatocellular Carcinoma with Portal Vein Tumour Thrombus in the Era of Artificial Intelligence.J Hepatocell Carcinoma. 2024 Jul 17;11:1429-1438. doi: 10.2147/JHC.S474922. eCollection 2024. J Hepatocell Carcinoma. 2024. PMID: 39050809 Free PMC article. Review.
-
Diagnosis of focal liver lesions from ultrasound images using a pretrained residual neural network.J Appl Clin Med Phys. 2024 Jan;25(1):e14210. doi: 10.1002/acm2.14210. Epub 2023 Nov 22. J Appl Clin Med Phys. 2024. PMID: 37991141 Free PMC article.
References
-
- Chaiteerakij R, Chattieng P, Choi J, Pinchareon N, Thanapirom K, Geratikornsupuk N. Surveillance for Hepatocellular Carcinoma Reduces Mortality: an Inverse Probability of Treatment Weighted Analysis. Annals of hepatology. 2017;16(3):421–9. Epub 2017/04/21. doi: 10.5604/16652681.1235485 . - DOI - PubMed
-
- Singal AG, Pillai A, Tiro J. Early detection, curative treatment, and survival rates for hepatocellular carcinoma surveillance in patients with cirrhosis: a meta-analysis. PLoS medicine. 2014;11(4):e1001624. Epub 2014/04/03. doi: 10.1371/journal.pmed.1001624 ; PubMed Central PMCID: PMC3972088. - DOI - PMC - PubMed
-
- Yang JD, Mannalithara A, Piscitello AJ, Kisiel JB, Gores GJ, Roberts LR, et al.. Impact of surveillance for hepatocellular carcinoma on survival in patients with compensated cirrhosis. Hepatology (Baltimore, Md). 2018;68(1):78–88. Epub 2017/10/13. doi: 10.1002/hep.29594 ; PubMed Central PMCID: PMC5897179. - DOI - PMC - PubMed
Publication types
MeSH terms
Grants and funding
LinkOut - more resources
Full Text Sources
Medical