Decoupling epithelial-mesenchymal transitions from stromal profiles by integrative expression analysis
- PMID: 33972543
- PMCID: PMC8110844
- DOI: 10.1038/s41467-021-22800-1
Decoupling epithelial-mesenchymal transitions from stromal profiles by integrative expression analysis
Abstract
Epithelial-to-mesenchymal transition (EMT) is the most commonly cited mechanism for cancer metastasis, but it is difficult to distinguish from profiles of normal stromal cells in the tumour microenvironment. In this study we use published single cell RNA-seq data to directly compare mesenchymal signatures from cancer and stromal cells. Informed by these comparisons, we developed a computational framework to decouple these two sources of mesenchymal expression profiles using bulk RNA-seq datasets. This deconvolution offers the opportunity to characterise EMT across hundreds of tumours and examine its association with metastasis and other clinical features. With this approach, we find three distinct patterns of EMT, associated with squamous, gynaecological and gastrointestinal cancer types. Surprisingly, in most cancer types, EMT patterns are not associated with increased chance of metastasis, suggesting that other steps in the metastatic cascade may represent the main bottleneck. This work provides a comprehensive evaluation of EMT profiles and their functional significance across hundreds of tumours while circumventing the confounding effect of stromal cells.
Conflict of interest statement
The authors declare no competing interests.
Figures
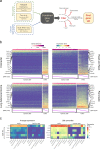
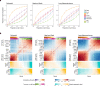
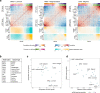
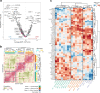
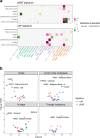
Similar articles
-
Stromal Signals Dominate Gene Expression Signature Scores That Aim to Describe Cancer Cell-intrinsic Stemness or Mesenchymality Characteristics.Cancer Res Commun. 2024 Feb 23;4(2):516-529. doi: 10.1158/2767-9764.CRC-23-0383. Cancer Res Commun. 2024. PMID: 38349551 Free PMC article.
-
Epithelial-to-mesenchymal transition signature assessment in colorectal cancer quantifies tumour stromal content rather than true transition.J Pathol. 2018 Dec;246(4):422-426. doi: 10.1002/path.5155. J Pathol. 2018. PMID: 30105762 Free PMC article.
-
MiR-137 regulates epithelial-mesenchymal transition in gastrointestinal stromal tumor.Tumour Biol. 2014 Sep;35(9):9131-8. doi: 10.1007/s13277-014-2177-5. Epub 2014 Jun 12. Tumour Biol. 2014. PMID: 25027394
-
Epithelial-mesenchymal transition in carcinomas of the female genital tract.Histopathology. 2013 Jan;62(1):31-43. doi: 10.1111/his.12057. Histopathology. 2013. PMID: 23240668 Review.
-
Long non-coding RNAs as emerging regulators of epithelial to mesenchymal transition in gynecologic cancers.Biosci Trends. 2018 Sep 19;12(4):342-353. doi: 10.5582/bst.2018.01181. Epub 2018 Aug 27. Biosci Trends. 2018. PMID: 30146551 Review.
Cited by
-
Signatures of EMT, immunosuppression, and inflammation in primary and recurrent human cutaneous squamous cell carcinoma at single-cell resolution.Theranostics. 2022 Oct 31;12(17):7532-7549. doi: 10.7150/thno.77528. eCollection 2022. Theranostics. 2022. PMID: 36438481 Free PMC article.
-
Mesenchymal ovarian cancer cells promote CD8+ T cell exhaustion through the LGALS3-LAG3 axis.NPJ Syst Biol Appl. 2023 Dec 12;9(1):61. doi: 10.1038/s41540-023-00322-4. NPJ Syst Biol Appl. 2023. PMID: 38086828 Free PMC article.
-
The Role of Tumor Epithelial-Mesenchymal Transition and Macrophage Crosstalk in Cancer Progression.Curr Osteoporos Rep. 2023 Apr;21(2):117-127. doi: 10.1007/s11914-023-00780-z. Epub 2023 Feb 27. Curr Osteoporos Rep. 2023. PMID: 36848026 Free PMC article. Review.
-
A transcriptomic map of EGFR-induced epithelial-to-mesenchymal transition identifies prognostic and therapeutic targets for head and neck cancer.Mol Cancer. 2022 Sep 8;21(1):178. doi: 10.1186/s12943-022-01646-1. Mol Cancer. 2022. PMID: 36076232 Free PMC article.
-
A hybrid epithelial/mesenchymal state in head and neck cancer: A biomarker for survival with differential prognosis by self-reported race.Med. 2024 Jul 12;5(7):826-831.e3. doi: 10.1016/j.medj.2024.05.014. Epub 2024 Jun 19. Med. 2024. PMID: 38901426
References
Publication types
MeSH terms
LinkOut - more resources
Full Text Sources
Other Literature Sources