A pan-cancer transcriptome analysis of exitron splicing identifies novel cancer driver genes and neoepitopes
- PMID: 33861991
- PMCID: PMC8141048
- DOI: 10.1016/j.molcel.2021.03.028
A pan-cancer transcriptome analysis of exitron splicing identifies novel cancer driver genes and neoepitopes
Abstract
Exitron splicing (EIS) creates a cryptic intron (called an exitron) within a protein-coding exon to increase proteome diversity. EIS is poorly characterized, but emerging evidence suggests a role for EIS in cancer. Through a systematic investigation of EIS across 33 cancers from 9,599 tumor transcriptomes, we discovered that EIS affected 63% of human coding genes and that 95% of those events were tumor specific. Notably, we observed a mutually exclusive pattern between EIS and somatic mutations in their affected genes. Functionally, we discovered that EIS altered known and novel cancer driver genes for causing gain- or loss-of-function, which promotes tumor progression. Importantly, we identified EIS-derived neoepitopes that bind to major histocompatibility complex (MHC) class I or II. Analysis of clinical data from a clear cell renal cell carcinoma cohort revealed an association between EIS-derived neoantigen load and checkpoint inhibitor response. Our findings establish the importance of considering EIS alterations when nominating cancer driver events and neoantigens.
Keywords: GTEx; TCGA; cancer driver genes; checkpoint inhibition immunotherapy; exitron; immunopeptidome; neoantigens; non-canonical splicing; pan-cancer analysis; transcriptome alterations.
Copyright © 2021 Elsevier Inc. All rights reserved.
Conflict of interest statement
Declaration of interests The authors declare no competing interests.
Figures
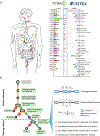
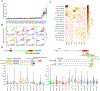
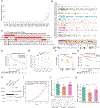
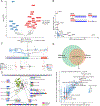
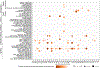
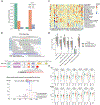
Similar articles
-
Integrated protocol for exitron and exitron-derived neoantigen identification using human RNA-seq data with ScanExitron and ScanNeo.STAR Protoc. 2021 Sep 3;2(3):100788. doi: 10.1016/j.xpro.2021.100788. eCollection 2021 Sep 17. STAR Protoc. 2021. PMID: 34522901 Free PMC article.
-
Comprehensive profiling of cancer neoantigens from aberrant RNA splicing.J Immunother Cancer. 2024 May 15;12(5):e008988. doi: 10.1136/jitc-2024-008988. J Immunother Cancer. 2024. PMID: 38754917 Free PMC article.
-
Unmasking alternative splicing inside protein-coding exons defines exitrons and their role in proteome plasticity.Genome Res. 2015 Jul;25(7):995-1007. doi: 10.1101/gr.186585.114. Epub 2015 May 1. Genome Res. 2015. PMID: 25934563 Free PMC article.
-
The pathobiology of splicing.J Pathol. 2010 Jan;220(2):152-63. doi: 10.1002/path.2649. J Pathol. 2010. PMID: 19918805 Free PMC article. Review.
-
Roles and mechanisms of alternative splicing in cancer - implications for care.Nat Rev Clin Oncol. 2020 Aug;17(8):457-474. doi: 10.1038/s41571-020-0350-x. Epub 2020 Apr 17. Nat Rev Clin Oncol. 2020. PMID: 32303702 Review.
Cited by
-
Crucial Parameters for Immunopeptidome Characterization: A Systematic Evaluation.Int J Mol Sci. 2024 Sep 3;25(17):9564. doi: 10.3390/ijms25179564. Int J Mol Sci. 2024. PMID: 39273511 Free PMC article.
-
ABA Mediates Plant Development and Abiotic Stress via Alternative Splicing.Int J Mol Sci. 2022 Mar 30;23(7):3796. doi: 10.3390/ijms23073796. Int J Mol Sci. 2022. PMID: 35409156 Free PMC article. Review.
-
Integrated protocol for exitron and exitron-derived neoantigen identification using human RNA-seq data with ScanExitron and ScanNeo.STAR Protoc. 2021 Sep 3;2(3):100788. doi: 10.1016/j.xpro.2021.100788. eCollection 2021 Sep 17. STAR Protoc. 2021. PMID: 34522901 Free PMC article.
-
Steering research on mRNA splicing in cancer towards clinical translation.Nat Rev Cancer. 2024 Dec;24(12):887-905. doi: 10.1038/s41568-024-00750-2. Epub 2024 Oct 9. Nat Rev Cancer. 2024. PMID: 39384951 Free PMC article. Review.
-
Relevance of mutation-derived neoantigens and non-classical antigens for anticancer therapies.Hum Vaccin Immunother. 2024 Dec 31;20(1):2303799. doi: 10.1080/21645515.2024.2303799. Epub 2024 Feb 12. Hum Vaccin Immunother. 2024. PMID: 38346926 Free PMC article. Review.
References
Publication types
MeSH terms
Substances
Grants and funding
LinkOut - more resources
Full Text Sources
Other Literature Sources
Research Materials