A novel defined pyroptosis-related gene signature for predicting the prognosis of ovarian cancer
- PMID: 33828074
- PMCID: PMC8026591
- DOI: 10.1038/s41420-021-00451-x
A novel defined pyroptosis-related gene signature for predicting the prognosis of ovarian cancer
Abstract
Ovarian cancer (OC) is a highly malignant gynaecological tumour that has a very poor prognosis. Pyroptosis has been demonstrated in recent years to be an inflammatory form of programmed cell death. However, the expression of pyroptosis-related genes in OC and their correlations with prognosis remain unclear. In this study, we identified 31 pyroptosis regulators that were differentially expressed between OC and normal ovarian tissues. Based on these differentially expressed genes (DEGs), all OC cases could be divided into two subtypes. The prognostic value of each pyroptosis-related gene for survival was evaluated to construct a multigene signature using The Cancer Genome Atlas (TCGA) cohort. By applying the least absolute shrinkage and selection operator (LASSO) Cox regression method, a 7-gene signature was built and classified all OC patients in the TCGA cohort into a low- or high-risk group. OC patients in the low-risk group showed significantly higher survival possibilities than those in the high-risk group (P < 0.001). Utilizing the median risk score from the TCGA cohort, OC patients from a Gene Expression Omnibus (GEO) cohort were divided into two risk subgroups, and the low-risk group had increased overall survival (OS) time (P = 0.014). Combined with the clinical characteristics, the risk score was found to be an independent factor for predicting the OS of OC patients. Gene ontology (GO) and Kyoto Encylopedia of Genes and Genomes (KEGG) analyses indicated that immune-related genes were enriched and that the immune status was decreased in the high-risk group. In conclusion, pyroptosis-related genes play important roles in tumour immunity and can be used to predict the prognosis of OCs.
Conflict of interest statement
The authors declare no competing interests.
Figures
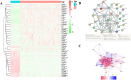
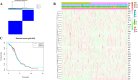
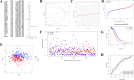
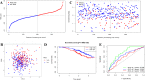
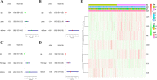
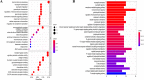
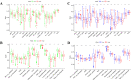
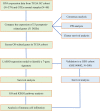
Similar articles
-
A Novel Defined Risk Signature of the Ferroptosis-Related Genes for Predicting the Prognosis of Ovarian Cancer.Front Mol Biosci. 2021 Apr 1;8:645845. doi: 10.3389/fmolb.2021.645845. eCollection 2021. Front Mol Biosci. 2021. PMID: 33869286 Free PMC article.
-
Construction and validation of a novel pyroptosis-related signature to predict prognosis in patients with cutaneous melanoma.Math Biosci Eng. 2022 Jan;19(1):688-706. doi: 10.3934/mbe.2022031. Epub 2021 Nov 19. Math Biosci Eng. 2022. PMID: 34903008
-
Prognostic Implications of Pyroptosis-Related Gene Signatures in Lung Squamous Cell Carcinoma.Front Pharmacol. 2022 Jan 27;13:806995. doi: 10.3389/fphar.2022.806995. eCollection 2022. Front Pharmacol. 2022. PMID: 35153782 Free PMC article.
-
Identification of a pyroptosis-related prognosis gene signature and its relationship with an immune microenvironment in gliomas.Medicine (Baltimore). 2022 Jul 15;101(28):e29391. doi: 10.1097/MD.0000000000029391. Medicine (Baltimore). 2022. PMID: 35839032 Free PMC article.
-
Personalized Medicine in Ovarian Cancer: A Perspective From Mexico.World J Oncol. 2021 Aug;12(4):85-92. doi: 10.14740/wjon1383. Epub 2021 Jul 10. World J Oncol. 2021. PMID: 34349852 Free PMC article. Review.
Cited by
-
Anoikis regulator GLI2 promotes NC cell immunity escape by TGF-β-mediated non-classic hedgehog signaling in colorectal cancer: based on artificial intelligence and big data analysis.Aging (Albany NY). 2023 Dec 29;15(24):14733-14748. doi: 10.18632/aging.205283. Epub 2023 Dec 29. Aging (Albany NY). 2023. PMID: 38159250 Free PMC article.
-
Derivation, Comprehensive Analysis, and Assay Validation of a Pyroptosis-Related lncRNA Prognostic Signature in Patients With Ovarian Cancer.Front Oncol. 2022 Feb 24;12:780950. doi: 10.3389/fonc.2022.780950. eCollection 2022. Front Oncol. 2022. PMID: 35280739 Free PMC article.
-
Pyroptosis-related lncRNAs are potential biomarkers for predicting prognoses and immune responses in patients with UCEC.Mol Ther Nucleic Acids. 2022 Jan 25;27:1036-1055. doi: 10.1016/j.omtn.2022.01.018. eCollection 2022 Mar 8. Mol Ther Nucleic Acids. 2022. PMID: 35228898 Free PMC article.
-
Development of a prognostic pyroptosis-related gene signature for head and neck squamous cell carcinoma patient.Cancer Cell Int. 2022 Feb 5;22(1):62. doi: 10.1186/s12935-022-02476-3. Cancer Cell Int. 2022. PMID: 35123464 Free PMC article.
-
Pyroptosis impacts the prognosis and treatment response in gastric cancer via immune system modulation.Am J Cancer Res. 2022 Apr 15;12(4):1511-1534. eCollection 2022. Am J Cancer Res. 2022. PMID: 35530274 Free PMC article.
References
-
- Siegel R, Miller K, Jemal A. Cancer statistics, 2019. CA. 2019;69:7–34. - PubMed
Grants and funding
LinkOut - more resources
Full Text Sources
Other Literature Sources